Deep learning-accelerated T2-weighted imaging versus conventional T2-weighted imaging in the female pelvic cavity: image quality and diagnostic performance.
Acta radiologica (Stockholm, Sweden : 1987)(2024)
摘要
BACKGROUND:The deep learning (DL)-based reconstruction algorithm reduces noise in magnetic resonance imaging (MRI), thereby enabling faster MRI acquisition.
PURPOSE:To compare the image quality and diagnostic performance of conventional turbo spin-echo (TSE) T2-weighted (T2W) imaging with DL-accelerated sagittal T2W imaging in the female pelvic cavity.
METHODS:This study evaluated 149 consecutive female pelvic MRI examinations, including conventional T2W imaging with TSE (acquisition time = 2:59) and DL-accelerated T2W imaging with breath hold (DL-BH) (1:05 [0:14 × 3 breath-holds]) in the sagittal plane. In 294 randomly ordered sagittal T2W images, two radiologists independently assessed image quality (sharpness, subjective noise, artifacts, and overall image quality), made a diagnosis for uterine leiomyomas, and scored diagnostic confidence. For the uterus and piriformis muscle, quantitative imaging analysis was also performed. Wilcoxon signed rank tests were used to compare the two sets of T2W images.
RESULTS:In the qualitative analysis, DL-BH showed similar or significantly higher scores for all features than conventional T2W imaging (P <0.05). In the quantitative analysis, the noise in the uterus was lower in DL-BH, but the noise in the muscle was lower in conventional T2W imaging. In the uterus and muscle, the signal-to-noise ratio was significantly lower in DL-BH than in conventional T2W imaging (P <0.001). The diagnostic performance of the two sets of T2W images was not different for uterine leiomyoma.
CONCLUSIONS:DL-accelerated sagittal T2W imaging obtained with three breath-holds demonstrated superior or comparable image quality to conventional T2W imaging with no significant difference in diagnostic performance for uterine leiomyomas.
更多查看译文
AI 理解论文
溯源树
样例
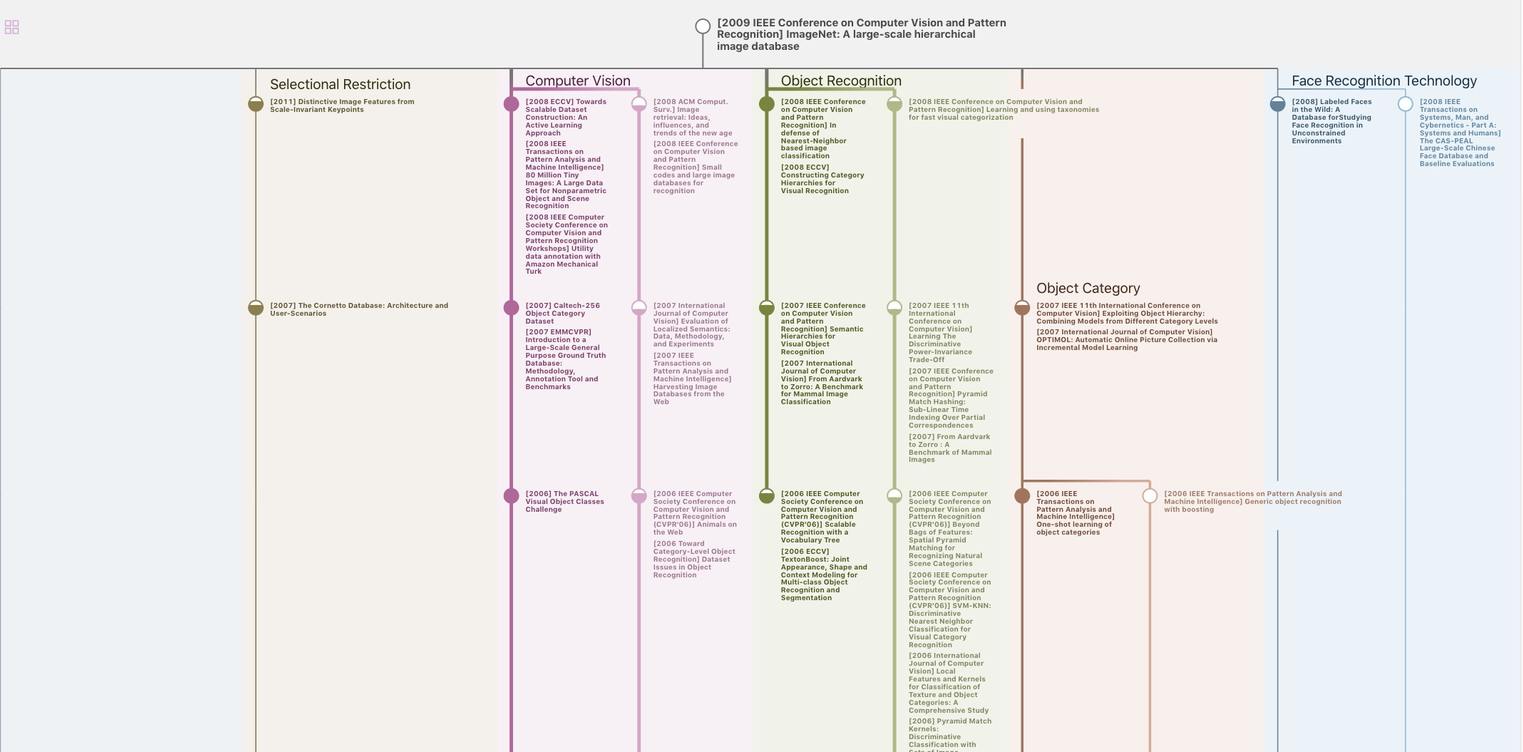
生成溯源树,研究论文发展脉络
Chat Paper
正在生成论文摘要