SREMIC: Spatial Relation Extraction-based Malware Image Classification.
International Conference on Ubiquitous Information Management and Communication(2024)
摘要
Around 800,000 people fall prey to cyberattacks annually, most often by “malware”. Malware has the potential to become a destructive weapon in Cyber-world. It is a difficult task to manually thwart an assault by malware. It is crucial to properly categorize malware binaries in order to identify their origins. Furthermore, malware structure discovery through basic feature extraction approaches are time-consuming and challenging. Malware classification was previously solved using naive machine learning approaches like support vector machine (SVM) and extreme gradient boosting (XGBoost). Recently, deep learning (DL) has shown to be impactful in finding malicious patterns. Without DL, analysis of the vast amounts of available data tends to impossible. Existing methods (e.g., transfer learning, fusion methodology, ensemble learning) may not be effective on actual malware binary files. Moreover, some single image-based malware classification used rudimentary convolutional neural network (CNN) that does not perform well. Faced with these challenges, we propose in this paper a novel model with of a spatial CNN with sufficient regularization and data augmentation that can identify and classify malware in images effectively and efficiently. Our model is evaluated using datasets like MalImg and Microfsoft-Big. The proposed model achieves validation score of 99.93% for MalImg and 99.72% for Microsoft-Big datasets. Our approach outperforms VGG16, VGG19, ResNet50, EfficientNetB1, and Google's Inception v3, including state-of-the-art (SOTA) techniques.
更多查看译文
关键词
Malware classification,Deep learning,Convolutional neural network (CNN),Spatial relation
AI 理解论文
溯源树
样例
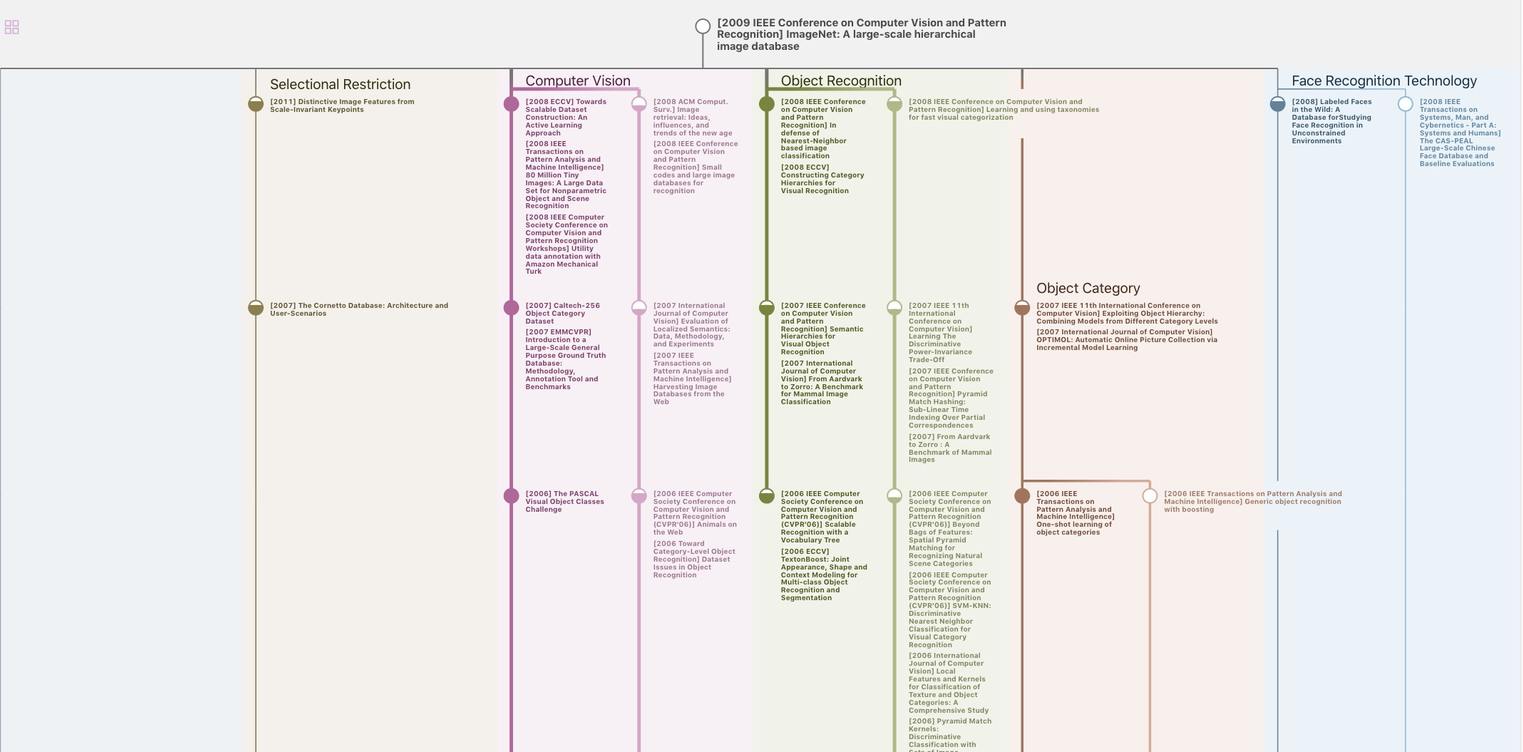
生成溯源树,研究论文发展脉络
Chat Paper
正在生成论文摘要