Adaptive Optimal Output Regulation of Interconnected Singularly Perturbed Systems with Application to Power Systems.
IEEE CAA J. Autom. Sinica(2024)
摘要
This article studies the adaptive optimal output regulation problem for a class of interconnected singularly perturbed systems (SPSs) with unknown dynamics based on reinforcement learning (RL). Taking into account the slow and fast characteristics among system states, the interconnected SPS is decomposed into the slow time-scale dynamics and the fast time-scale dynamics through singular perturbation theory. For the fast time-scale dynamics with interconnections, we devise a decentralized optimal control strategy by selecting appropriate weight matrices in the cost function. For the slow time-scale dynamics with unknown system parameters, an off-policy RL algorithm with convergence guarantee is given to learn the optimal control strategy in terms of measurement data. By combining the slow and fast controllers, we establish the composite decentralized adaptive optimal output regulator, and rigorously analyze the stability and optimality of the closed-loop system. The proposed decomposition design not only bypasses the numerical stiffness but also alleviates the high-dimensionality. The efficacy of the proposed methodology is validated by a load-frequency control application of a two-area power system.
更多查看译文
关键词
Adaptive optimal control,decentralized control,output regulation,reinforcement learning (RL),singularly perturbed systems (SPSs)
AI 理解论文
溯源树
样例
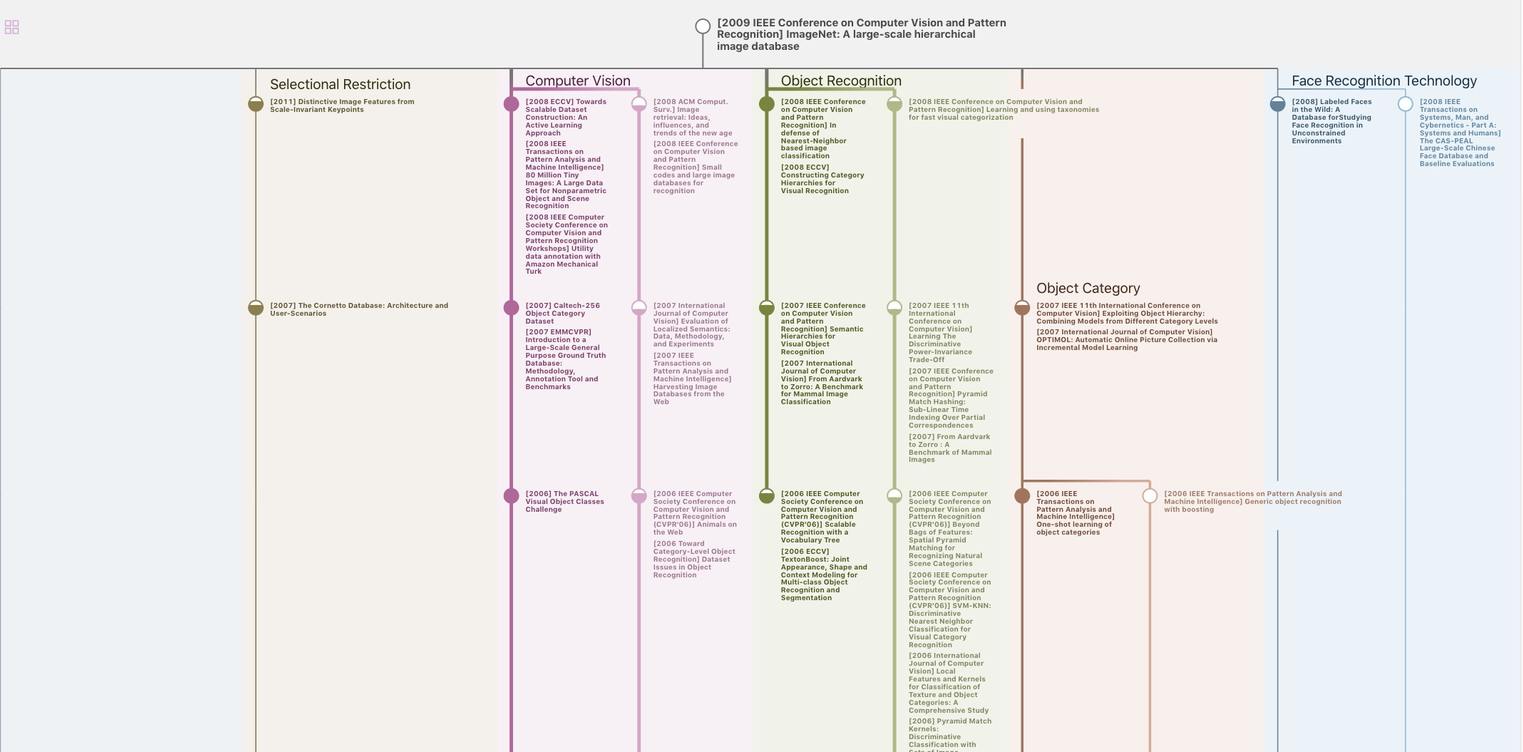
生成溯源树,研究论文发展脉络
Chat Paper
正在生成论文摘要