Masking Hierarchical Tokens for Underwater Acoustic Target Recognition With Self-Supervised Learning
IEEE-ACM TRANSACTIONS ON AUDIO SPEECH AND LANGUAGE PROCESSING(2024)
摘要
Deep learning has made data-driven methods effective in underwater acoustic target recognition (UATR) using passive sonar signals. However, a major current challenge is the limited availability of underwater acoustic data, leading to suboptimal performance without sufficient data. Self-supervised learning (SSL) can help address this problem by learning intrinsic patterns within acoustic data. Nonetheless, applying SSL in UATR systems requires efficient learning of meaningful representations that can provide quick prediction speed for real-time recognition systems. To this end, we propose the masking hierarchical tokens (MHT) method to learn meaningful representations via efficient self-supervised learning for our previously proposed UATR-Transformer, giving rise to the MHT-UATR-Transformer. In particular, the MHT-UATR-Transformer first exploits a new designed token-convolution-based hierarchical tokenization to efficiently obtain rich time-frequency information from the input Mel-spectrogram. Then, most of these tokens are masked with a high masking ratio and subsequently reconstructed by an integrated Encoder-Decoder structure. In this way, the MHT-UATR-Transformer can learn intrinsic representations of underwater acoustic signals to achieve better recognition performance with fewer labeled data, which is expected to alleviate the dependency on expensive acoustic data. Experimental results on two widely studied underwater databases show that our proposed method achieves better performance than supervised learning and state-of-the-art SSL method in both accuracy and speed, especially in few-shot and noisy scenarios, thus enhancing its practicality in real marine applications.
更多查看译文
关键词
Transformers,Tokenization,Feature extraction,Underwater acoustics,Task analysis,Target recognition,Spectrogram,Underwater acoustic target recognition,self-supervised,hierarchical tokens,UATR-Transformer
AI 理解论文
溯源树
样例
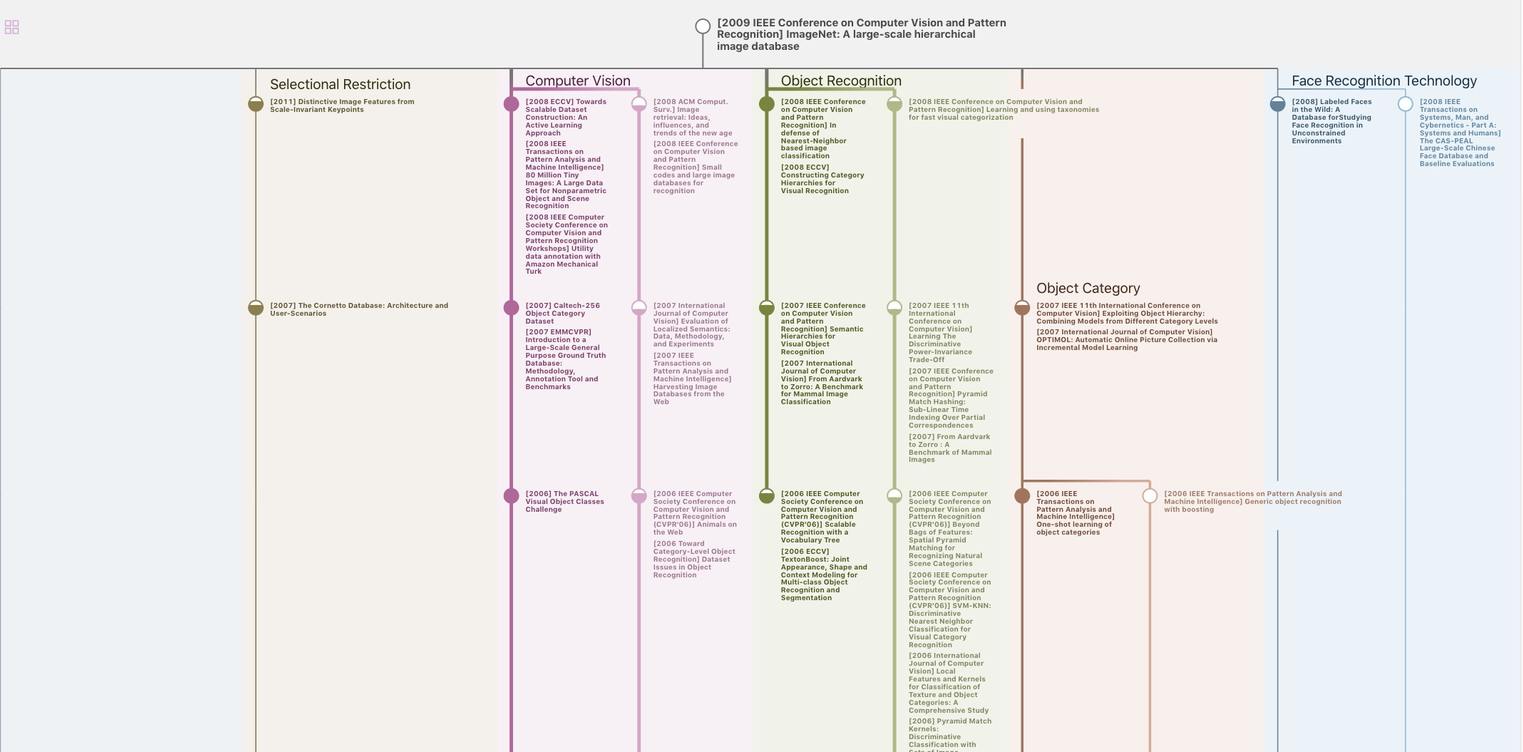
生成溯源树,研究论文发展脉络
Chat Paper
正在生成论文摘要