Generative Deep Learning Techniques for Traffic Matrix Estimation From Link Load Measurements
IEEE OPEN JOURNAL OF THE COMMUNICATIONS SOCIETY(2024)
摘要
Traffic matrices (TMs) contain crucial information for managing networks, optimizing traffic flow, and detecting anomalies. However, directly measuring traffic to construct a TM is resource-intensive and computationally expensive. A more practical approach involves estimating the TM from readily available link load measurements, which falls under the category of inferential network monitoring based on indirect measurements known as network tomography. This paper focuses on solving the problem of estimating the traffic matrix from link loads by utilizing deep generative models. The proposed models are trained using historical data-specifically, previously observed TMs-and are then leveraged to transform traffic matrix estimation (TME) into a simpler minimization problem in a lower-dimensional latent space. This transformed problem can be efficiently solved using a gradient-based optimizer. Our work aims to examine and test different model architectures and optimization approaches. The performance of the proposed methods is comparatively evaluated over a comprehensive set of suitable metrics on two publicly available datasets comprising actual traffic matrices obtained from real backbone networks. In addition, we compare our approach with a state-of-the-art method previously published in the literature.
更多查看译文
关键词
Estimation,Routing,Data models,Mathematical models,Load modeling,Optimization,Deep learning,tomography,traffic matrix estimation,variational autoencoder
AI 理解论文
溯源树
样例
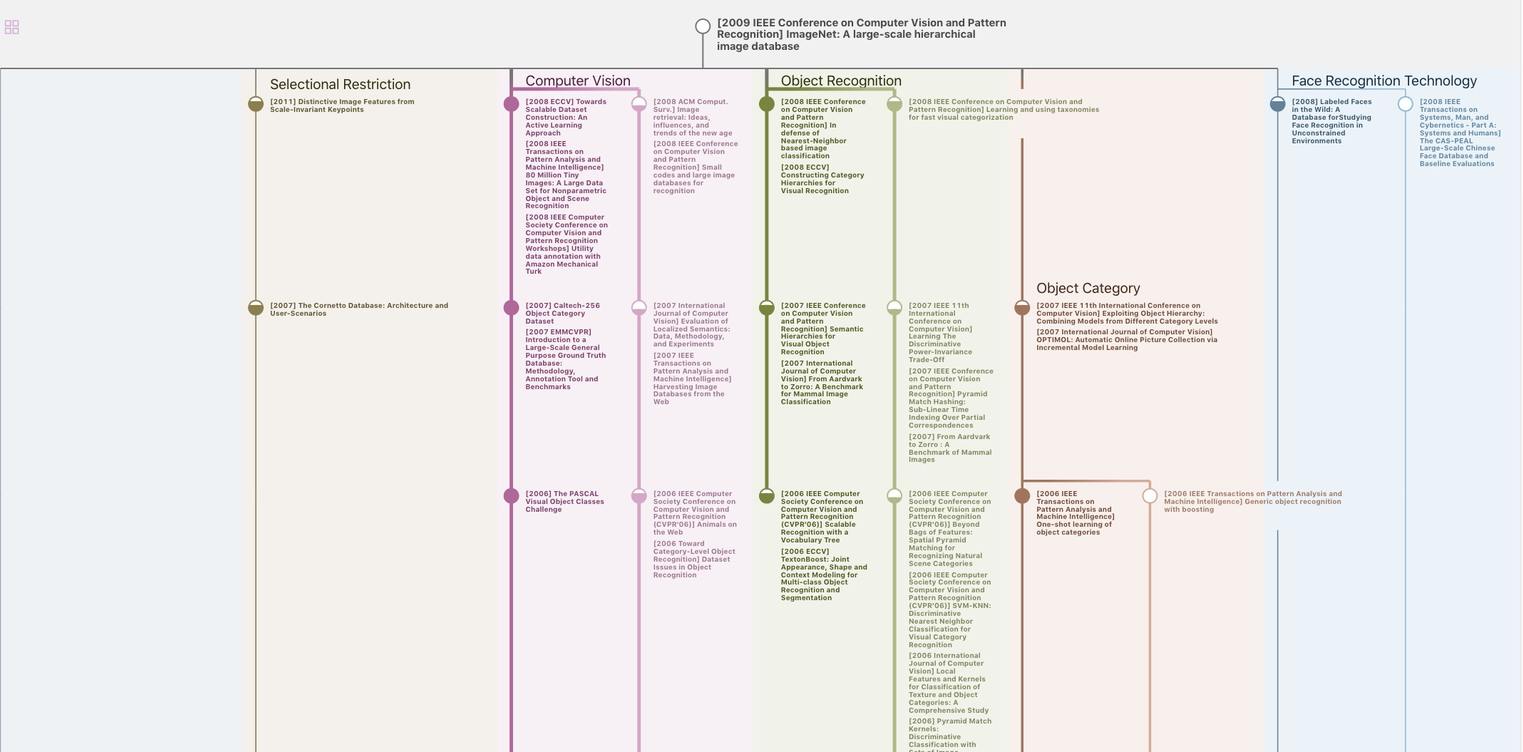
生成溯源树,研究论文发展脉络
Chat Paper
正在生成论文摘要