Graph Descriptive Order Improves Reasoning with Large Language Model
CoRR(2024)
摘要
In recent years, large language models have achieved state-of-the-art
performance across multiple domains. However, the progress in the field of
graph reasoning with LLM remains limited. Our work delves into this gap by
thoroughly investigating graph reasoning with LLMs. In this work, we reveal the
impact of the order of graph description on LLMs' graph reasoning performance,
which significantly affects LLMs' reasoning abilities. By altering this order,
we enhance the performance of LLMs from 42.22% to 70%. Furthermore, we
introduce the Scaled Graph Reasoning benchmark for assessing LLMs' performance
across various graph sizes and evaluate the relationship between LLMs' graph
reasoning abilities and graph size. We discover that the graph reasoning
performance of LLMs does not monotonically decrease with the increase in graph
size. The experiments span several mainstream models, including GPT-3.5,
LLaMA-2-7B, and LLaMA-2-13B, to offer a comprehensive evaluation.
更多查看译文
AI 理解论文
溯源树
样例
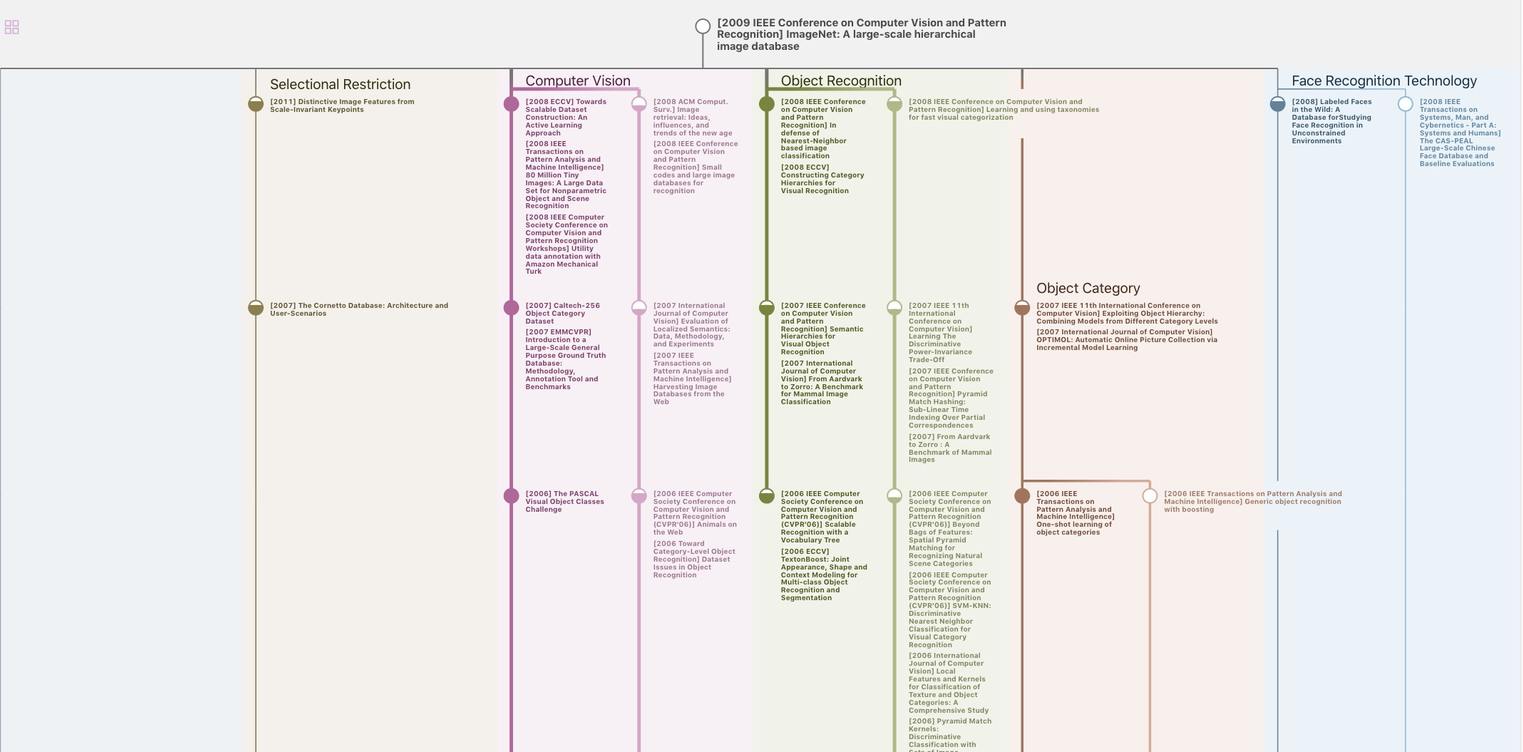
生成溯源树,研究论文发展脉络
Chat Paper
正在生成论文摘要