Non-autoregressive Generative Models for Reranking Recommendation
CoRR(2024)
摘要
In a multi-stage recommendation system, reranking plays a crucial role by
modeling the intra-list correlations among items.The key challenge of reranking
lies in the exploration of optimal sequences within the combinatorial space of
permutations. Recent research proposes a generator-evaluator learning paradigm,
where the generator generates multiple feasible sequences and the evaluator
picks out the best sequence based on the estimated listwise score. Generator is
of vital importance, and generative models are well-suited for the generator
function. Current generative models employ an autoregressive strategy for
sequence generation. However, deploying autoregressive models in real-time
industrial systems is challenging. Hence, we propose a Non-AutoRegressive
generative model for reranking Recommendation (NAR4Rec) designed to enhance
efficiency and effectiveness. To address challenges related to sparse training
samples and dynamic candidates impacting model convergence, we introduce a
matching model. Considering the diverse nature of user feedback, we propose a
sequence-level unlikelihood training objective to distinguish feasible from
unfeasible sequences. Additionally, to overcome the lack of dependency modeling
in non-autoregressive models regarding target items, we introduce contrastive
decoding to capture correlations among these items. Extensive offline
experiments on publicly available datasets validate the superior performance of
our proposed approach compared to the existing state-of-the-art reranking
methods. Furthermore, our method has been fully deployed in a popular video app
Kuaishou with over 300 million daily active users, significantly enhancing
online recommendation quality, and demonstrating the effectiveness and
efficiency of our approach.
更多查看译文
AI 理解论文
溯源树
样例
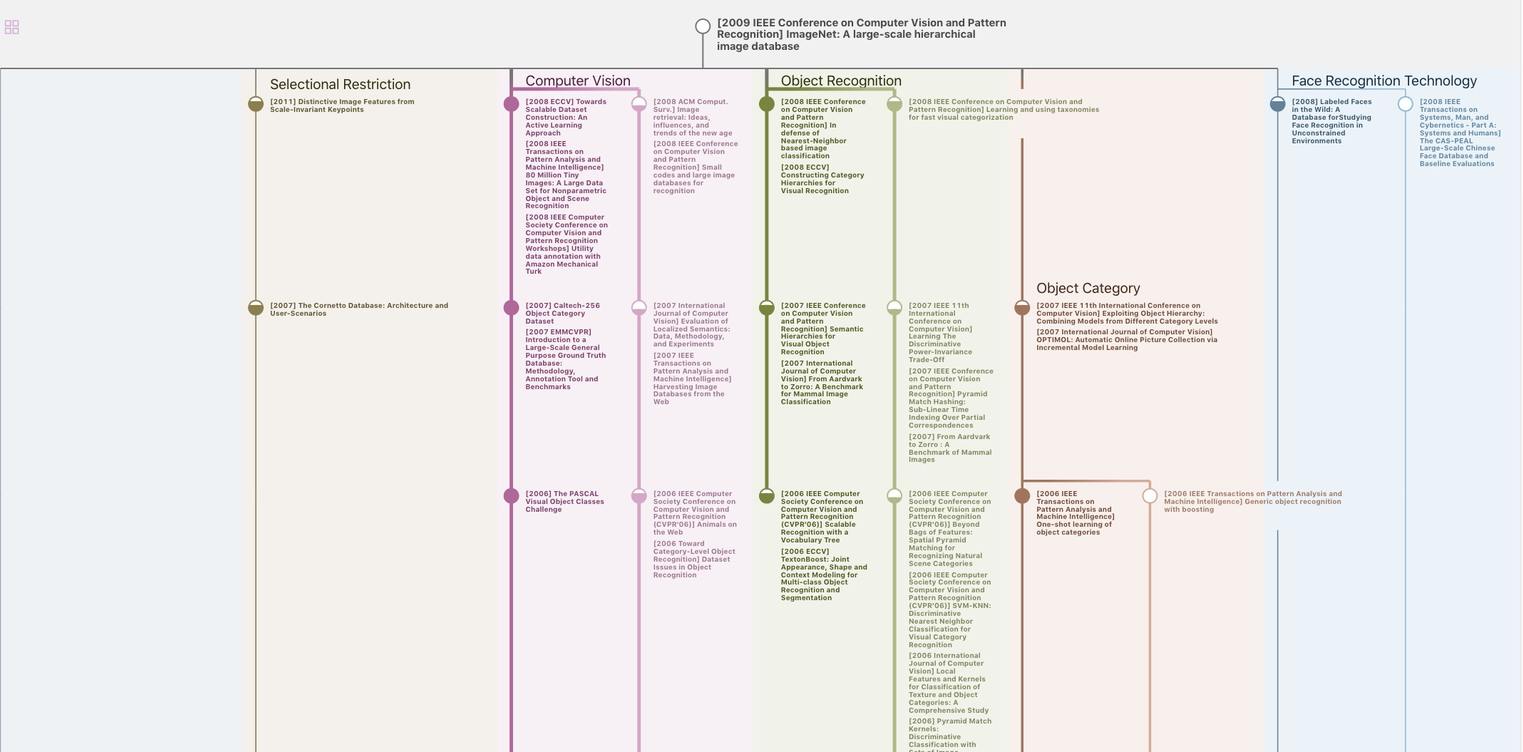
生成溯源树,研究论文发展脉络
Chat Paper
正在生成论文摘要