On the Distance from Calibration in Sequential Prediction
CoRR(2024)
摘要
We study a sequential binary prediction setting where the forecaster is
evaluated in terms of the calibration distance, which is defined as the L_1
distance between the predicted values and the set of predictions that are
perfectly calibrated in hindsight. This is analogous to a calibration measure
recently proposed by Błasiok, Gopalan, Hu and Nakkiran (STOC 2023) for the
offline setting. The calibration distance is a natural and intuitive measure of
deviation from perfect calibration, and satisfies a Lipschitz continuity
property which does not hold for many popular calibration measures, such as the
L_1 calibration error and its variants.
We prove that there is a forecasting algorithm that achieves an O(√(T))
calibration distance in expectation on an adversarially chosen sequence of T
binary outcomes. At the core of this upper bound is a structural result showing
that the calibration distance is accurately approximated by the lower
calibration distance, which is a continuous relaxation of the former. We then
show that an O(√(T)) lower calibration distance can be achieved via a
simple minimax argument and a reduction to online learning on a Lipschitz
class.
On the lower bound side, an Ω(T^1/3) calibration distance is shown
to be unavoidable, even when the adversary outputs a sequence of independent
random bits, and has an additional ability to early stop (i.e., to stop
producing random bits and output the same bit in the remaining steps).
Interestingly, without this early stopping, the forecaster can achieve a much
smaller calibration distance of polylog(T).
更多查看译文
AI 理解论文
溯源树
样例
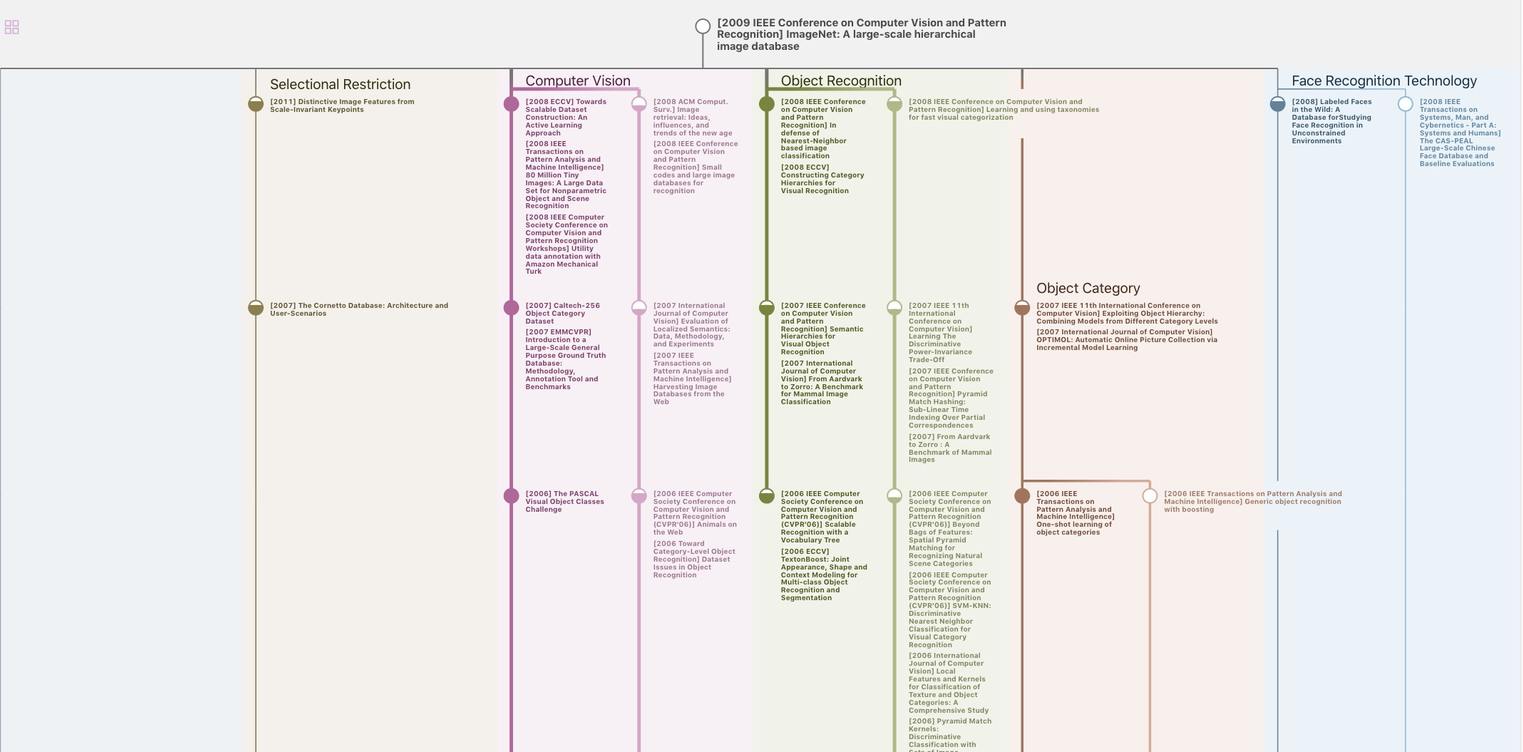
生成溯源树,研究论文发展脉络
Chat Paper
正在生成论文摘要