More than the Sum of Its Parts: Ensembling Backbone Networks for Few-Shot Segmentation
CoRR(2024)
摘要
Semantic segmentation is a key prerequisite to robust image understanding for
applications in ai and Robotics. fss, in particular,
concerns the extension and optimization of traditional segmentation methods in
challenging conditions where limited training examples are available. A
predominant approach in fss is to rely on a single backbone for
visual feature extraction. Choosing which backbone to leverage is a deciding
factor contributing to the overall performance. In this work, we interrogate on
whether fusing features from different backbones can improve the ability of
fss models to capture richer visual features. To tackle this
question, we propose and compare two ensembling techniques-Independent Voting
and Feature Fusion. Among the available fss methods, we implement the
proposed ensembling techniques on PANet. The module dedicated to predicting
segmentation masks from the backbone embeddings in PANet avoids trainable
parameters, creating a controlled `in vitro' setting for isolating the impact
of different ensembling strategies. Leveraging the complementary strengths of
different backbones, our approach outperforms the original single-backbone
PANet across standard benchmarks even in challenging one-shot learning
scenarios. Specifically, it achieved a performance improvement of +7.37% on
PASCAL-5i and of +10.68% on COCO-20i in
the top-performing scenario where three backbones are combined. These results,
together with the qualitative inspection of the predicted subject masks,
suggest that relying on multiple backbones in PANet leads to a more
comprehensive feature representation, thus expediting the successful
application of fss methods in challenging, data-scarce environments.
更多查看译文
AI 理解论文
溯源树
样例
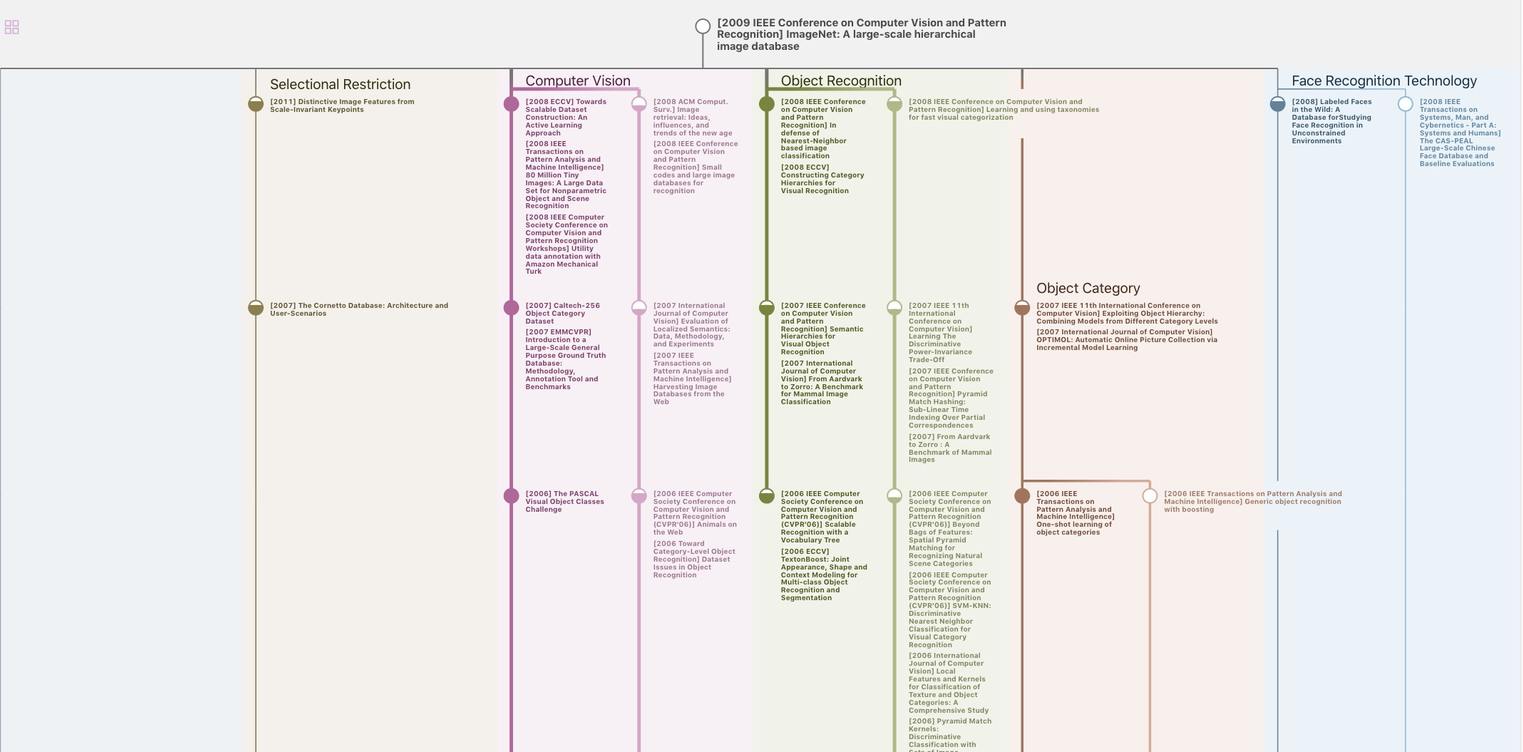
生成溯源树,研究论文发展脉络
Chat Paper
正在生成论文摘要