Model Editing with Canonical Examples
CoRR(2024)
摘要
We introduce model editing with canonical examples, a setting in which (1) a
single learning example is provided per desired behavior, (2) evaluation is
performed exclusively out-of-distribution, and (3) deviation from an initial
model is strictly limited. A canonical example is a simple instance of good
behavior, e.g., The capital of Mauritius is Port Louis) or bad behavior, e.g.,
An aspect of researchers is coldhearted). The evaluation set contains more
complex examples of each behavior (like a paragraph in which the capital of
Mauritius is called for.) We create three datasets and modify three more for
model editing with canonical examples, covering knowledge-intensive
improvements, social bias mitigation, and syntactic edge cases. In our
experiments on Pythia language models, we find that LoRA outperforms full
finetuning and MEMIT. We then turn to the Backpack language model architecture
because it is intended to enable targeted improvement. The Backpack defines a
large bank of sense vectors–a decomposition of the different uses of each
word–which are weighted and summed to form the output logits of the model. We
propose sense finetuning, which selects and finetunes a few (≈ 10)
sense vectors for each canonical example, and find that it outperforms other
finetuning methods, e.g., 4.8
GPT-J-6B by an inference-time ensemble with just the changes from sense
finetuning of a 35x smaller Backpack, in one setting outperforming editing
GPT-J itself (4.1
更多查看译文
AI 理解论文
溯源树
样例
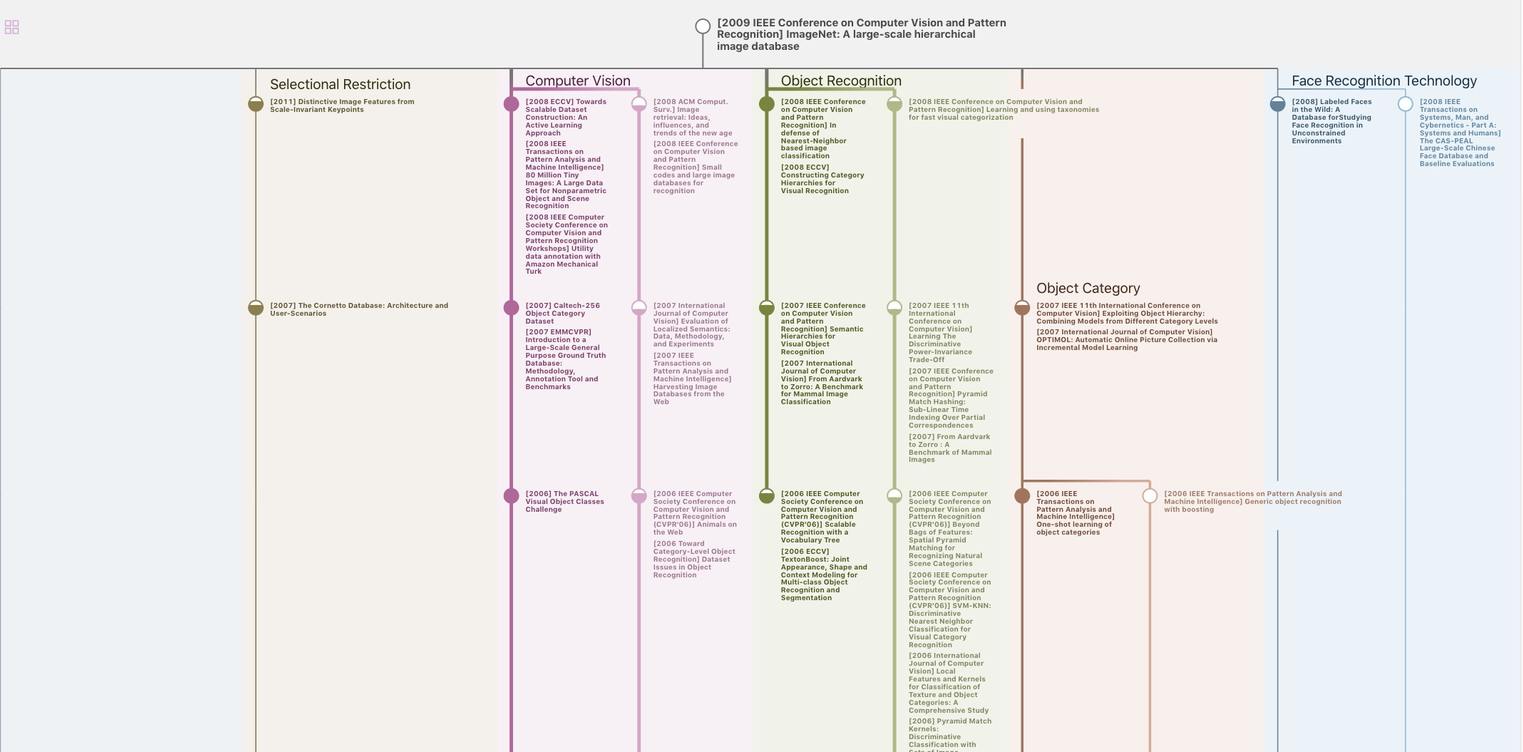
生成溯源树,研究论文发展脉络
Chat Paper
正在生成论文摘要