Doing Experiments and Revising Rules with Natural Language and Probabilistic Reasoning
arxiv(2024)
摘要
We build a computational model of how humans actively infer hidden rules by
doing experiments. The basic principles behind the model is that, even if the
rule is deterministic, the learner considers a broader space of fuzzy
probabilistic rules, which it represents in natural language, and updates its
hypotheses online after each experiment according to approximately Bayesian
principles. In the same framework we also model experiment design according to
information-theoretic criteria. We find that the combination of these three
principles – explicit hypotheses, probabilistic rules, and online updates –
can explain human performance on a Zendo-style task, and that removing any of
these components leaves the model unable to account for the data.
更多查看译文
AI 理解论文
溯源树
样例
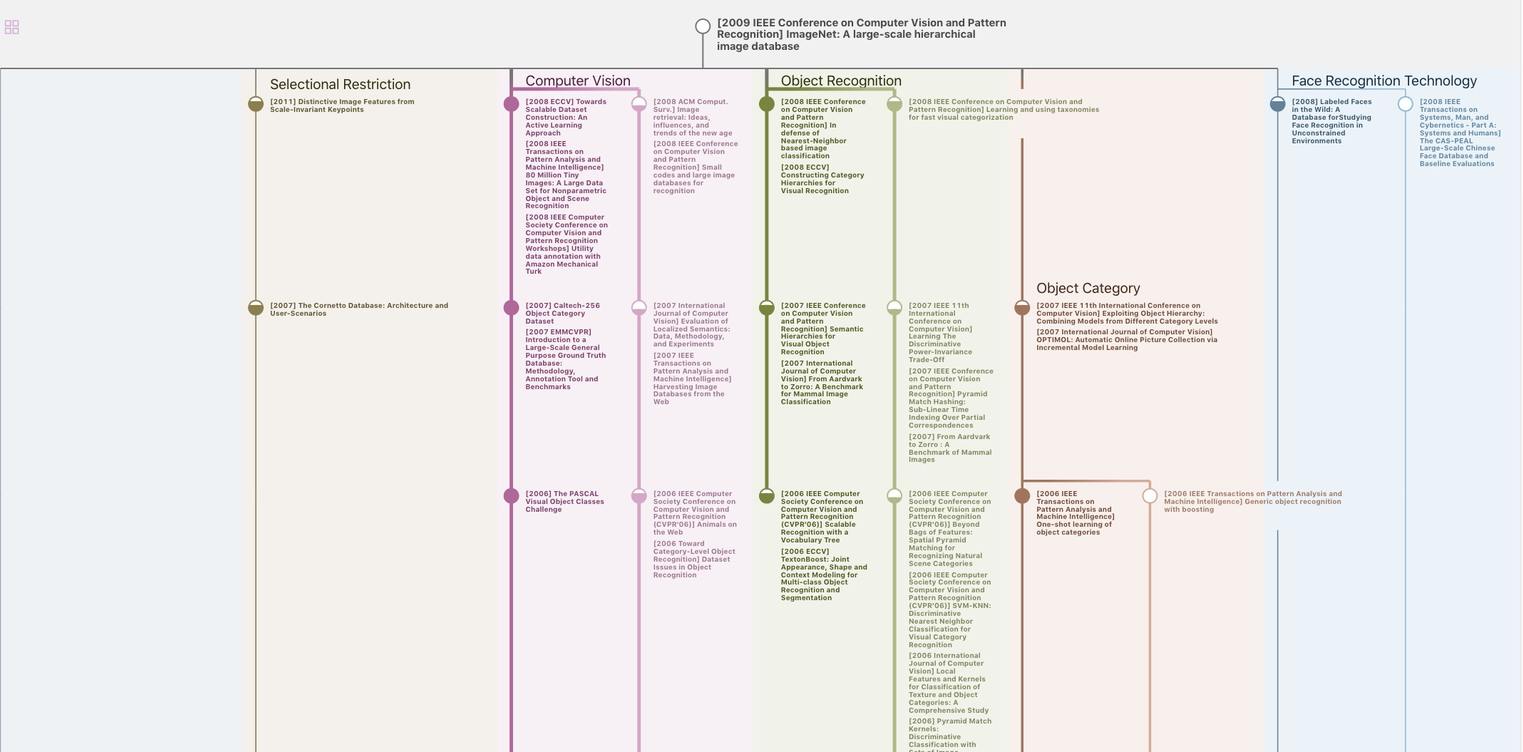
生成溯源树,研究论文发展脉络
Chat Paper
正在生成论文摘要