Decision Theory-Guided Deep Reinforcement Learning for Fast Learning
CoRR(2024)
摘要
This paper introduces a novel approach, Decision Theory-guided Deep
Reinforcement Learning (DT-guided DRL), to address the inherent cold start
problem in DRL. By integrating decision theory principles, DT-guided DRL
enhances agents' initial performance and robustness in complex environments,
enabling more efficient and reliable convergence during learning. Our
investigation encompasses two primary problem contexts: the cart pole and maze
navigation challenges. Experimental results demonstrate that the integration of
decision theory not only facilitates effective initial guidance for DRL agents
but also promotes a more structured and informed exploration strategy,
particularly in environments characterized by large and intricate state spaces.
The results of experiment demonstrate that DT-guided DRL can provide
significantly higher rewards compared to regular DRL. Specifically, during the
initial phase of training, the DT-guided DRL yields up to an 184
accumulated reward. Moreover, even after reaching convergence, it maintains a
superior performance, ending with up to 53
large maze problems. DT-guided DRL represents an advancement in mitigating a
fundamental challenge of DRL by leveraging functions informed by human
(designer) knowledge, setting a foundation for further research in this
promising interdisciplinary domain.
更多查看译文
AI 理解论文
溯源树
样例
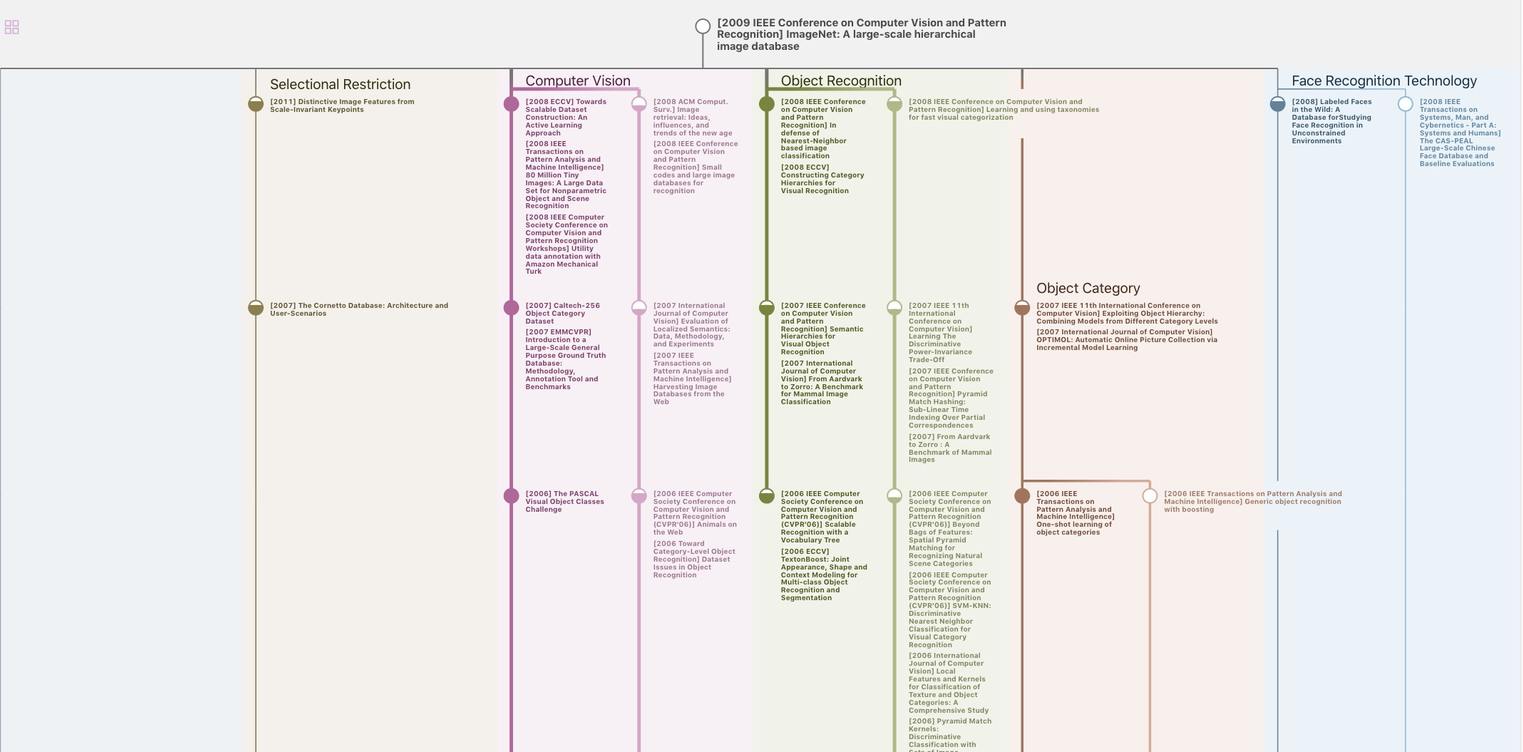
生成溯源树,研究论文发展脉络
Chat Paper
正在生成论文摘要