On the irreducibility and convergence of a class of nonsmooth nonlinear state-space models on manifolds
arxiv(2024)
摘要
In this paper, we analyze a large class of general nonlinear state-space
models on a state-space X, defined by the recursion $\phi_{k+1} =
F(\phi_k,\alpha(\phi_k,U_{k+1}))$, $k \in\bN$, where $F,\alpha$ are some
functions and $\{U_{k+1}\}_{k\in\bN}$ is a sequence of i.i.d. random variables.
More precisely, we extend conditions under which this class of Markov chains is
irreducible, aperiodic and satisfies important continuity properties, relaxing
two key assumptions from prior works. First, the state-space X is supposed to
be a smooth manifold instead of an open subset of a Euclidean space. Second, we
only suppose that $F is locally Lipschitz continuous.
We demonstrate the significance of our results through their application to
Markov chains underlying optimization algorithms. These schemes belong to the
class of evolution strategies with covariance matrix adaptation and step-size
adaptation.
更多查看译文
AI 理解论文
溯源树
样例
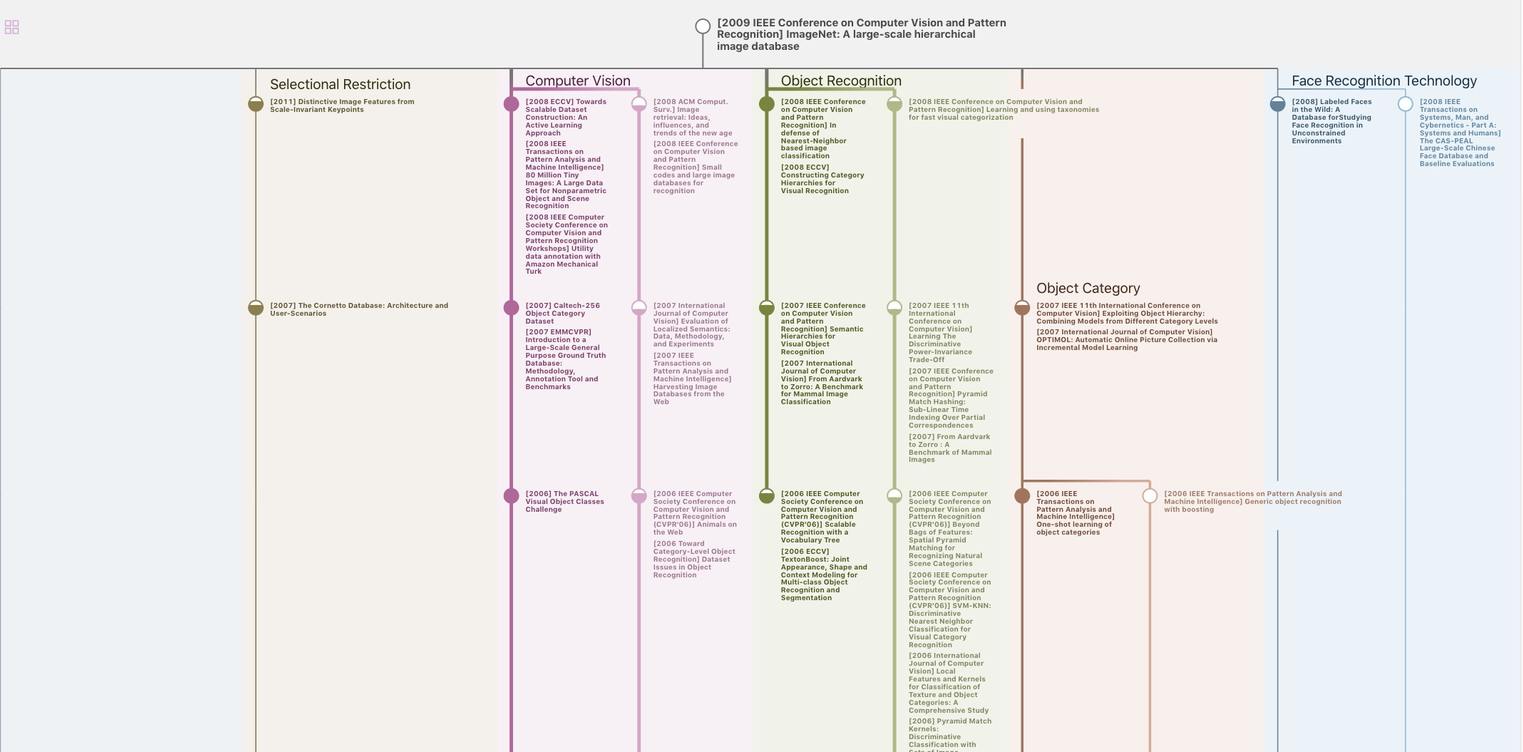
生成溯源树,研究论文发展脉络
Chat Paper
正在生成论文摘要