Reconfigurable stochastic neurons based on strain engineered low barrier nanomagnets.
Nanotechnology(2024)
摘要
Stochastic neurons are efficient hardware accelerators for solving a large variety of combinatorial optimization problems. "Binary" stochastic neurons (BSN) are those whose states fluctuate randomly between two levels +1 and -1, with the probability of being in either level determined by an external bias. "Analog" stochastic neurons (ASNs), in contrast, can assume any state between the two levels randomly (hence "analog") and can perform analog signal processing. They may be leveraged for such tasks as temporal sequence learning, processing and prediction. Both BSNs and ASNs can be used to build efficient and scalable neural networks. Both can be implemented with low (potential energy) barrier nanomagnets (LBMs) whose random magnetization orientations encode the binary or analog state variables. The difference between them is that the potential energy barrier in a BSN LBM, albeit low, is much higher than that in an ASN LBM. As a result, a BSN LBM has a clear double well potential profile, which makes its magnetization orientation assume one of two orientations at any time, resulting in the binary behavior. ASN nanomagnets, on the other hand, hardly have any energy barrier at all and hence lack the double well feature. That makes their magnetizations fluctuate in an analog fashion. Hence, one can reconfigure an ASN to a BSN, and vice-versa, by simply raising and lowering the energy barrier. If the LBM is magnetostrictive, then this can be done with local (electrically generated) strain. Such a reconfiguration capability heralds a powerful field programmable architecture for a p-computer whereby hardware for very different functionalities such as combinatorial optimization and temporal sequence learning can be integrated in the same substrate in the same processing run. This is somewhat reminiscent of heterogeneous integration, except this is integration of functionalities or computational fabrics rather than components. The energy cost of reconfiguration is miniscule. There are also other applications of strain mediated barrier control that do not involve reconfiguring a BSN to an ASN or vice versa, e.g., adaptive annealing in energy minimization computing (Boltzmann or Ising machines), emulating memory hierarchy in a dynamically reconfigurable fashion, and control over belief uncertainty in analog stochastic neurons. Here, we present a study of strain engineered barrier control in unconventional computing.
更多查看译文
AI 理解论文
溯源树
样例
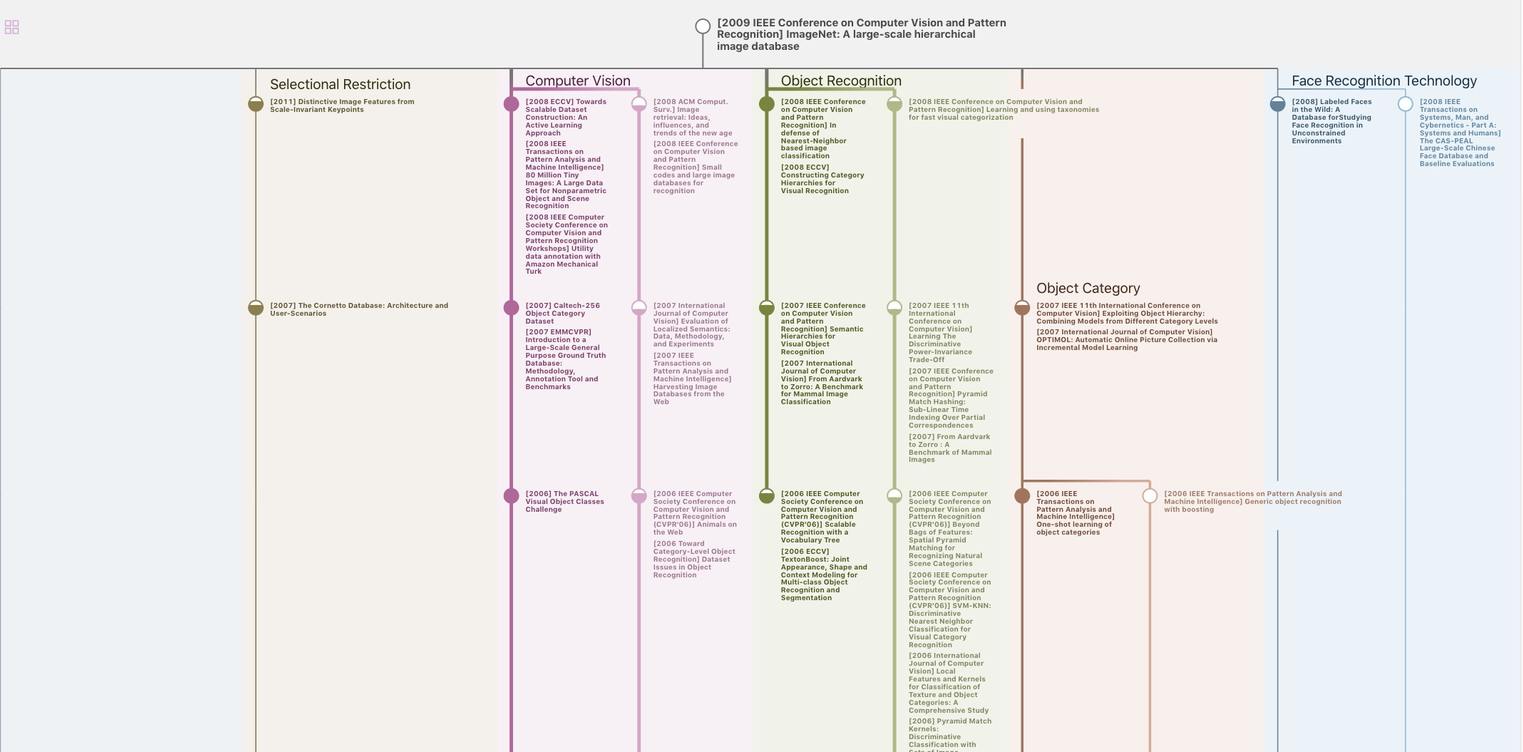
生成溯源树,研究论文发展脉络
Chat Paper
正在生成论文摘要