Accurate top protein variant discovery via low-N pick-and-validate machine learning
CELL SYSTEMS(2024)
摘要
A strategy to obtain the greatest number of best -performing variants with least amount of experimental effort over the vast combinatorial mutational landscape would have enormous utility in boosting resource producibility for protein engineering. Toward this goal, we present a simple and effective machine learning -based strategy that outperforms other state-of-the-art methods. Our strategy integrates zero -shot prediction and multi -round sampling to direct active learning via experimenting with only a few predicted top variants. We find that four rounds of low -N pick -and -validate sampling of 12 variants for machine learning yielded the best accuracy of up to 92.6% in selecting the true top 1% variants in combinatorial mutant libraries, whereas two rounds of 24 variants can also be used. We demonstrate our strategy in successfully discovering high-performance protein variants from diverse families including the CRISPR-based genome editors, supporting its generalizable application for solving protein engineering tasks. A record of this paper's transparent peer review process is included in the supplemental information.
更多查看译文
关键词
machine learning,low-N,zero-shot,CRISPR,base editor,genome editing,Cas9,combinatorial mutagenesis,protein engineering,active learning
AI 理解论文
溯源树
样例
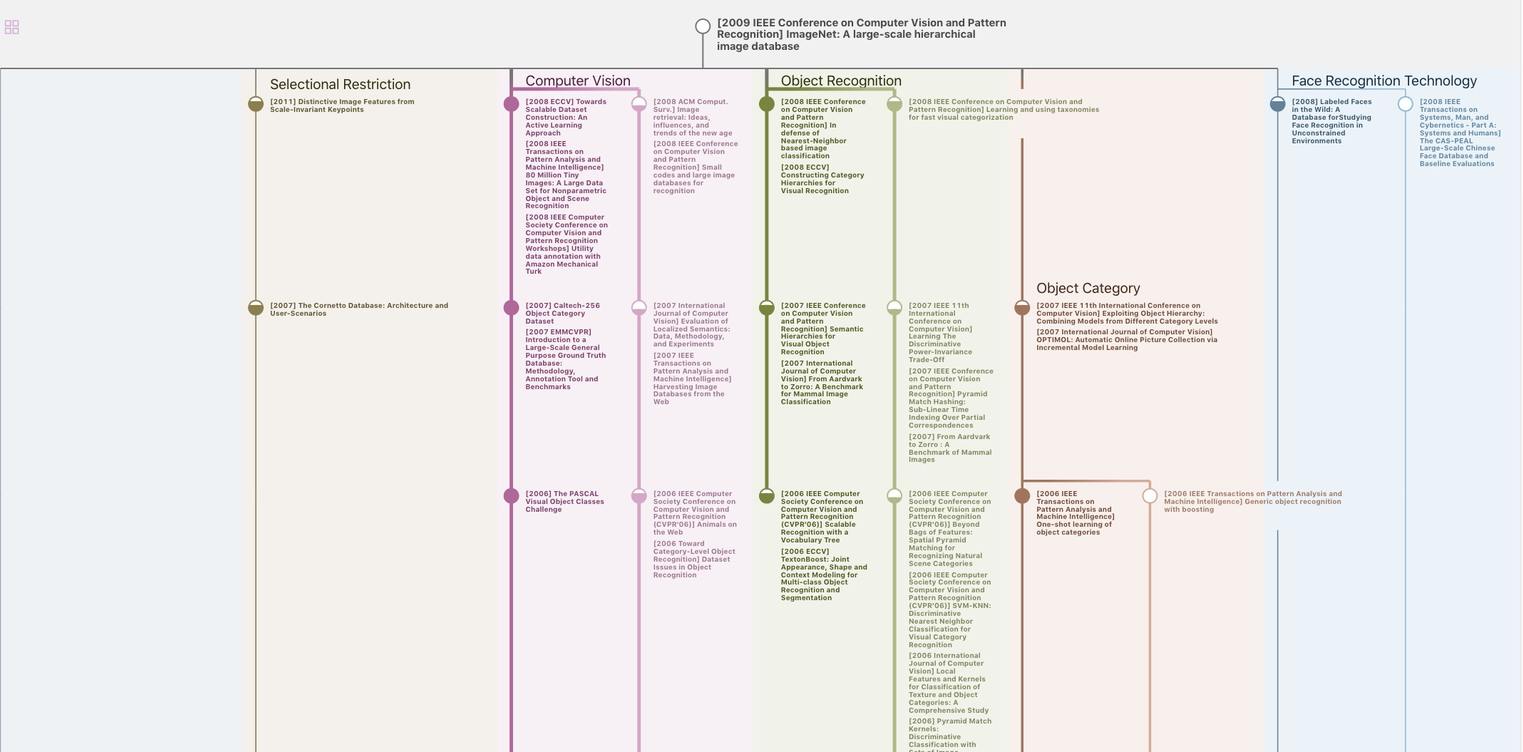
生成溯源树,研究论文发展脉络
Chat Paper
正在生成论文摘要