Automatic Segmentation of Continuous Time-Series Data Based on Prediction Error Using Deep Predictive Learning.
2024 IEEE/SICE International Symposium on System Integration (SII)(2024)
摘要
In deep learning, training with multiple modalities generally improves the learning performance. In robot motion learning, human knowledge information can be regarded as additional modalities and aids robots to perform their tasks. Previous studies have shown that annotation of action segments improves the generalizability of motion learning. However, manual segmentation causes inconsistencies of segmentation because of its subjectivity, Additionally manual segmentation is time-consuming for large-scale datasets. Automatic segmentation by generative models, such as hidden Markov models and predictive learning models, is an alternative approach to find the action segments and may be replaced with human knowledge information. In this study, we expand a predictive learning model using recurrent neural networks to automatic segmentation of continuous time-series data as a robot behavior. We assume that robot behavior can be described as sequences of complex time series composed of multiple simple patterns. As such, we utilize a learning model that can segment data into patterns through predictive learning. To do so, the continuous data is first segmented equally, and next the predictive learning model autonomously shifts the segmentation boundaries to minimize its prediction error. We conducted a numerical experiment that simulates a time series of sensor information. Comparing with the hidden Markov model, the results showed that our predictive learning model were able to estimate more suitable segmenting points.
更多查看译文
关键词
Time Series,Deep Learning,Continuous Data,Prediction Error,Time Series Data,Automatic Segmentation,Continuous Time Series,Time-series Database,Continuous Time Series Data,Prediction Model,Neural Network,Learning Models,Hidden Markov Model,Recurrent Neural Network,Multiple Modalities,Human Cognition,Robot Motion,Robot Behavior,Waveform,Deep Neural Network,Position Of Segment,Bias Parameter,Automatic Segmentation Method,Waveform Shape,Kinetic Data,Original Waveform,Segmentation Method,Average Prediction Error,Dynamic Sequence,Triangular Wave
AI 理解论文
溯源树
样例
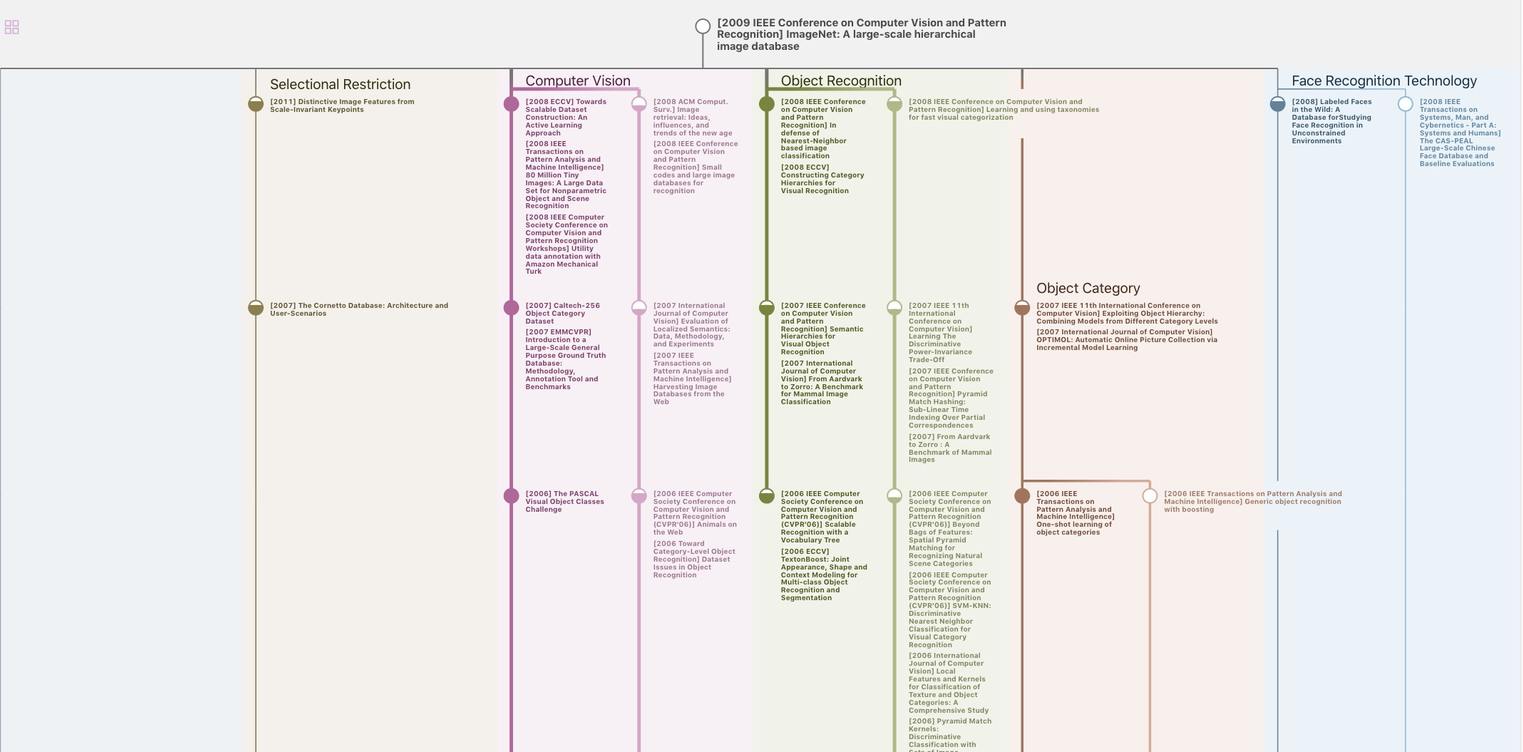
生成溯源树,研究论文发展脉络
Chat Paper
正在生成论文摘要