Feature extraction of particle morphologies of pharmaceutical excipients from scanning electron microscope images using convolutional neural networks
INTERNATIONAL JOURNAL OF PHARMACEUTICS(2024)
摘要
Scanning electron microscopy (SEM) images are the most widely used tool for evaluating particle morphology; however, quantitative evaluation using SEM images is time-consuming and often neglected. In this study, we aimed to extract features related to particle morphology of pharmaceutical excipients from SEM images using a convolutional neural network (CNN). SEM images of 67 excipients were acquired and used as models. A classification CNN model of the excipients was constructed based on the SEM images. Further, features were extracted from the middle layer of this CNN model, and the data was compressed to two dimensions using uniform manifold approximation and projection. Lastly, hierarchical clustering analysis (HCA) was performed to categorize the excipients into several clusters and identify similarities among the samples. The classification CNN model showed high accuracy, allowing each excipient to be identified with a high degree of accuracy. HCA revealed that the 67 excipients were classified into seven clusters. Additionally, the particle morphologies of excipients belonging to the same cluster were found to be very similar. These results suggest that CNN models are useful tools for extracting information and identifying similarities among the particle morphologies of excipients.
更多查看译文
关键词
Convolutional neural networks,Machine learning,Clustering,Scanning electron microscope,Raw materials database,Powder
AI 理解论文
溯源树
样例
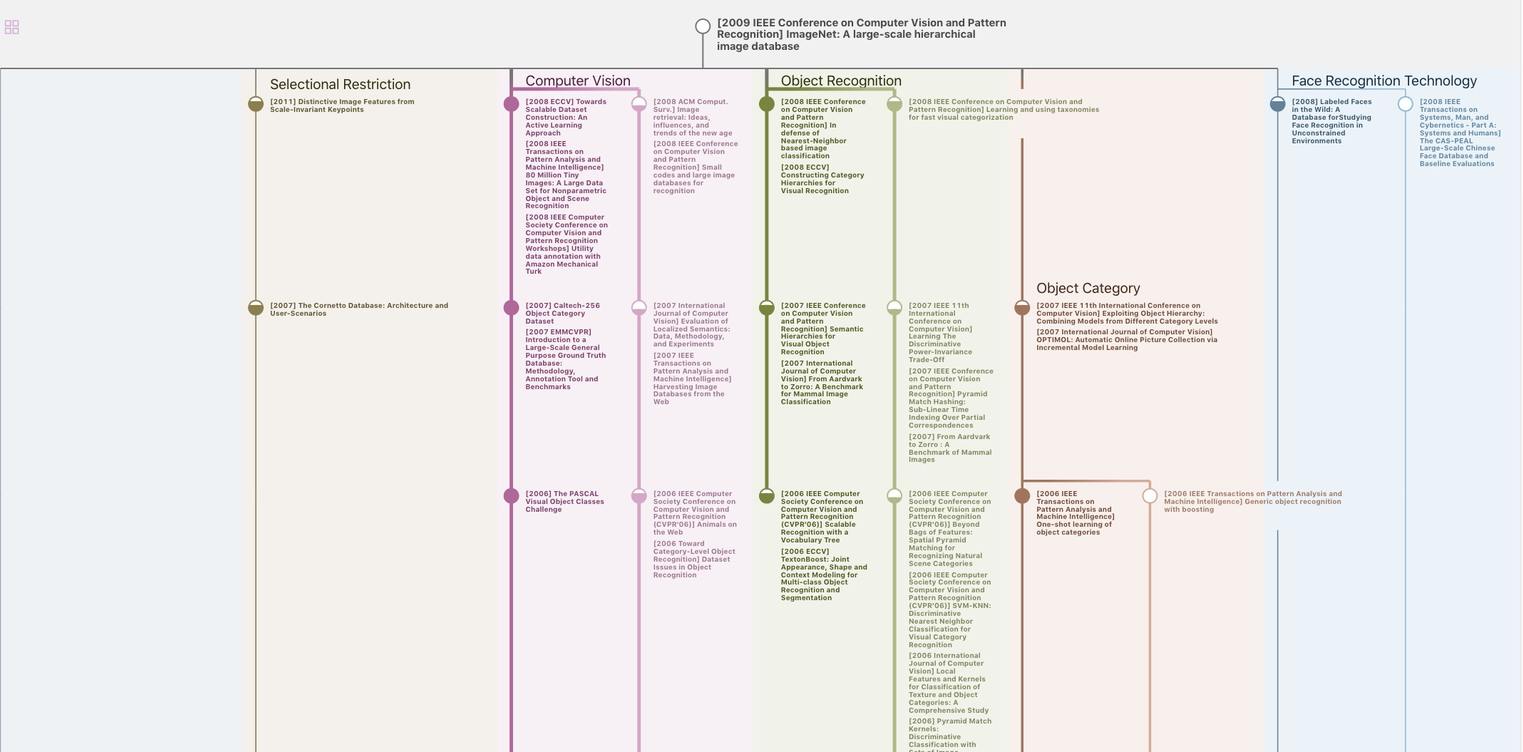
生成溯源树,研究论文发展脉络
Chat Paper
正在生成论文摘要