Generating Long-Horizon Task Actions by Leveraging Predictions of Environmental States.
2024 IEEE/SICE International Symposium on System Integration (SII)(2024)
摘要
To expand the usage of robots in daily life, it is crucial for robots to learn compounded and long-horizon tasks. To learn long-horizon tasks, the tasks are generally segmented into shorter subtasks. The segmentation of the task can be done autonomously or manually, and is often based on the environment changes such as movement of object positions. The environmental state of each subtask contains valuable information that may be used as an auxiliary input for the robot learning model. However, leveraging environmental states for robot learning has the following two difficulties: maintaining and remembering environmental states that are not observable, and predicting accurate environmental states. To address these difficulties, we propose a method that predicts environmental states and confidence of prediction to leverage environmental states. The proposed method improves learning performance in long-horizon tasks by maintaining the long-term memory of the environmental states through its prediction and considering the accuracy of the environmental states. In this study, we evaluate our method by executing a long-horizon manipulation task that requires memory of past environmental states to pick-and-place multiple cubes into appropriate boxes. The six-degree-of-freedom robot was able to correctly remember the past behavior and placed the cube into the box appropriately.
更多查看译文
关键词
Environmental Conditions,Long-horizon Tasks,Daily Life,Environmental Changes,Learning Models,Long-term Memory,Manipulation Tasks,Robot Learning,Improve Learning Performance,Training Data,Deep Learning,Time Step,Convolutional Layers,Prediction Error,Recurrent Neural Network,Sequential Model,Task Execution,Human Cognition,Fully-connected Layer,Binary Cross-entropy Loss,Inverse Reinforcement Learning,Daily Life Tasks,Sensor Information,Convolutional Autoencoder,Third Power,Vanishing Gradient,Environmental Transitions,Deconvolutional Layers,Action Labels,Gaussian Noise
AI 理解论文
溯源树
样例
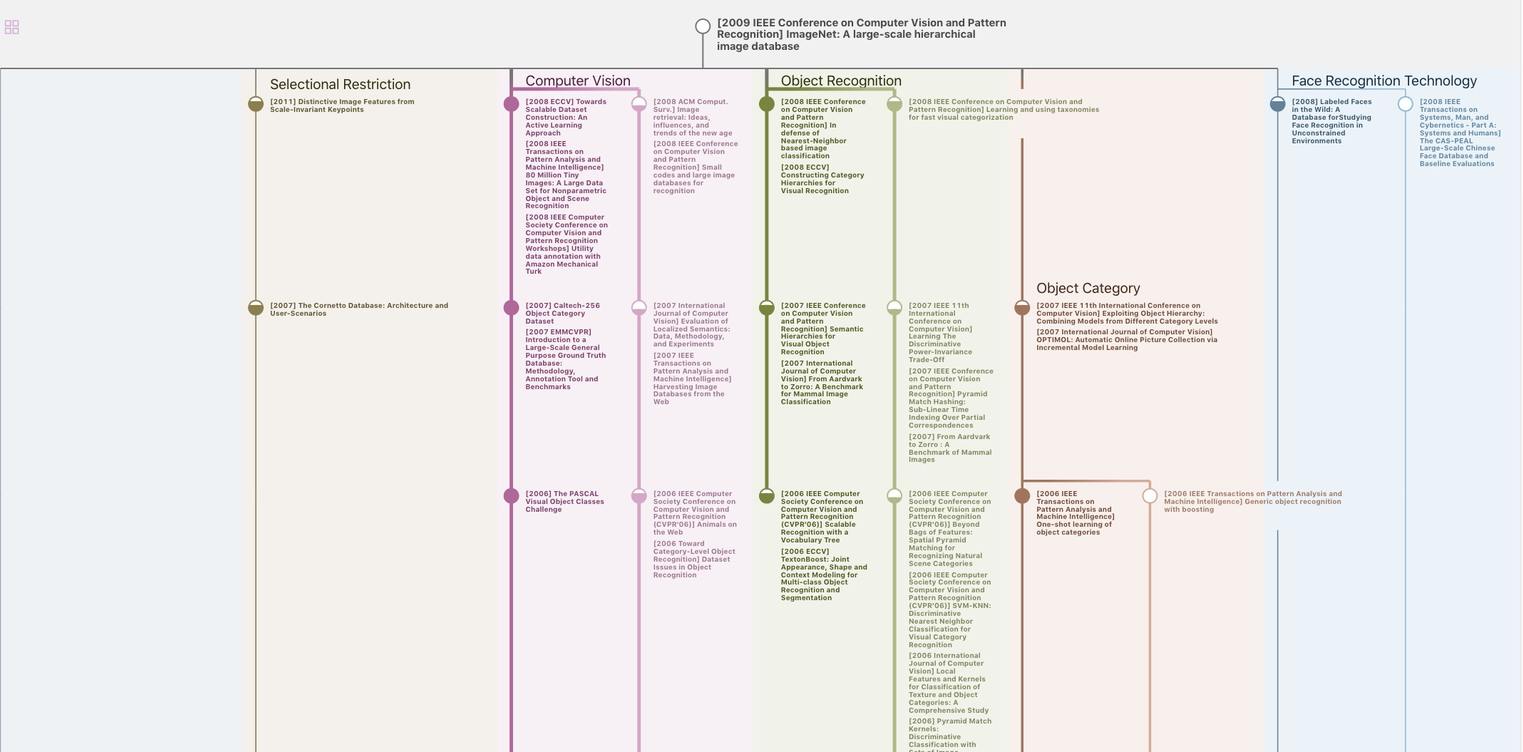
生成溯源树,研究论文发展脉络
Chat Paper
正在生成论文摘要