Application of Machine Learning Methods for Process Optimization in Electronic Packaging Processes
2023 24th European Microelectronics and Packaging Conference & Exhibition (EMPC)(2023)
摘要
Epoxy resins are commonly used as encapsulation materials in electronic packaging processes. Fluctuations in the materials lead to both changes in processability and to varying quality. Ideally, these variations should be identified, and measures taken as quickly as possible to reduce scrap parts and thus costs. A promising optimization approach for encapsulation processes are machine learning models, which have already shown good results in quality predictions, especially for injection molding. Subsequent quality measurements are avoided with good quality prediction models. With this type of models, not only predictions can be made, but also optimal parameter combinations can be found. In this paper, models for predicting quality criteria warpage and residual enthalpy of the epoxy molding compound were set up, trained and validated. Time series of in-situ sensors were used, from which relevant features were extracted and which, together with machine parameters, provide a dataset for prediction. The most promising prediction models are random forest regression and gradient boosting regression. They predict warpage with an accuracy of 90 % to 91 % and the residual enthalpy with an accuracy of 95 %. Subsequently, optimization models of the machine parameters were set up. All relevant target variables were considered in a cost function, through the minimization of which an optimal parameter set was found. The gradient boosted tree and Bayesian optimization were determined to be the most promising models, as they lead to the lowest values of the respective cost function.
更多查看译文
关键词
machine learning methods,warpage prediction,enthalpy prediction,process optimization,molding processes,epoxy molding compounds,electronic packaging
AI 理解论文
溯源树
样例
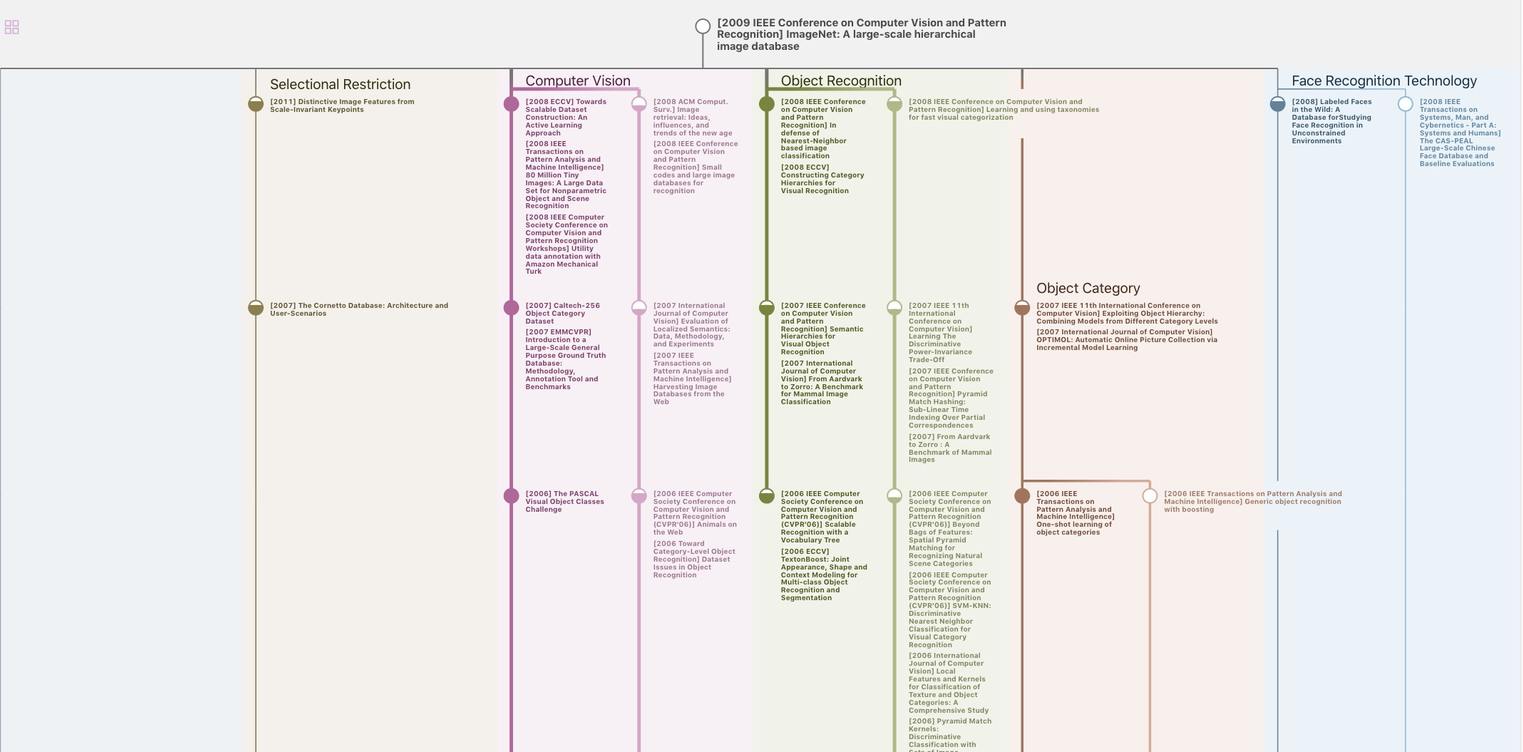
生成溯源树,研究论文发展脉络
Chat Paper
正在生成论文摘要