CDNA-SNN: A New Spiking Neural Network for Pattern Classification Using Neuronal Assemblies
IEEE TRANSACTIONS ON NEURAL NETWORKS AND LEARNING SYSTEMS(2024)
摘要
Spiking neural networks (SNNs) mimic their bio-logical counterparts more closely than their predecessors and are considered the third generation of artificial neural networks .It has been proven that networks of spiking neurons have a higher computational capacity and lower power requirements than sigmoidal neural networks. This article introduces a new type of SNN that draws inspiration and incorporates concepts from neuronal assemblies in the human brain. The proposed network, termed as class-dependent neuronal activation-based SNN (CDNA-SNN), assigns each neuron learnable values known as CDNAs which indicate the neuron's average relative spiking activity in response to samples from different classes. A new learning algorithm that categorizes the neurons into different class assemblies based on their CDNAs is also presented. These neuronal assemblies are trained via a novel training method based on spike-timing-dependent plasticity (STDP) to have high activity for their associated class and low firing rate for other classes. Also, using CDNAs, a new type of STDP that con-trols the amount of plasticity based on the assemblies of pre-and postsynaptic neurons is proposed. The performance of CDNA-SNN is evaluated on five datasets from the University of California, Irvine (UCI) machine learning repository, as well as Modified National Institute of Standards and Technology(MNIST) and Fashion MNIST, using nested cross-validation(N-CV) for hyperparameter optimization. Our results show that CDNA-SNN significantly outperforms synaptic weight associationtraining (SWAT) (p<0.0005) and Spike Prop (p<0.05) on 3/5and self-regulating evolving spiking neural (SRESN) (p<0.05)on 2/5 UCI datasets while using the significantly lower number of trainable parameters. Furthermore, compared to other super-vised, fully connected SNNs, the proposed SNN reaches the best performance for Fashion MNIST and comparable performance for MNIST and neuromorphic-MNIST (N-MNIST), also utilizingmuch less (1%-35%) parameters.
更多查看译文
关键词
Class-dependent neuronal activation (CDNA),leaky integrate and fire (LIF),neuronal assembly,spiking neural network (SNN),spiking neurons
AI 理解论文
溯源树
样例
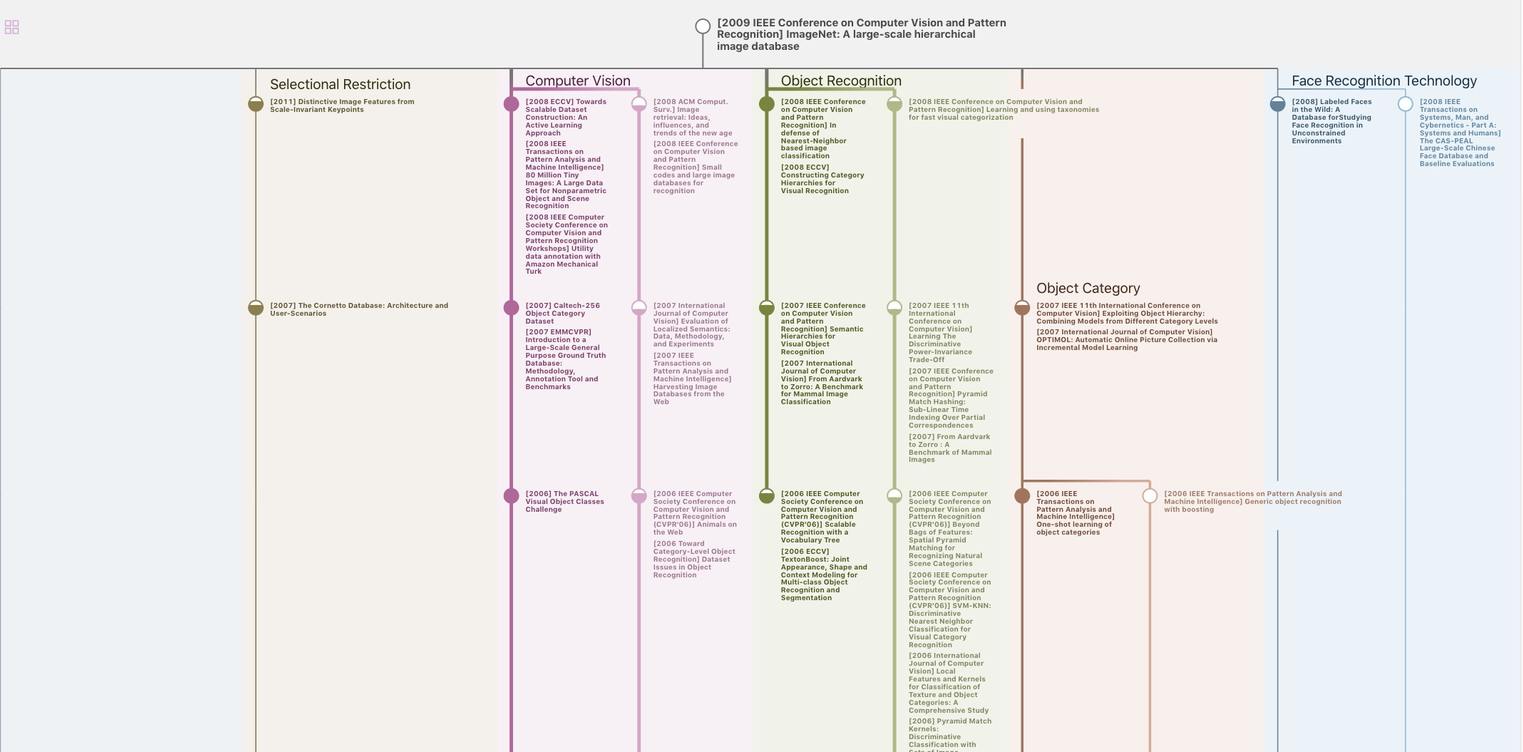
生成溯源树,研究论文发展脉络
Chat Paper
正在生成论文摘要