High-Dimensional Feature Selection for Automatic Classification of Coronary Stenosis Using an Evolutionary Algorithm
DIAGNOSTICS(2024)
摘要
In this paper, a novel strategy to perform high-dimensional feature selection using an evolutionary algorithm for the automatic classification of coronary stenosis is introduced. The method involves a feature extraction stage to form a bank of 473 features considering different types such as intensity, texture and shape. The feature selection task is carried out on a high-dimensional feature bank, where the search space is denoted by O(2n) and n=473. The proposed evolutionary search strategy was compared in terms of the Jaccard coefficient and accuracy classification with different state-of-the-art methods. The highest feature selection rate, along with the best classification performance, was obtained with a subset of four features, representing a 99% discrimination rate. In the last stage, the feature subset was used as input to train a support vector machine using an independent testing set. The classification of coronary stenosis cases involves a binary classification type by considering positive and negative classes. The highest classification performance was obtained with the four-feature subset in terms of accuracy (0.86) and Jaccard coefficient (0.75) metrics. In addition, a second dataset containing 2788 instances was formed from a public image database, obtaining an accuracy of 0.89 and a Jaccard Coefficient of 0.80. Finally, based on the performance achieved with the four-feature subset, they can be suitable for use in a clinical decision support system.
更多查看译文
关键词
bank of features,coronary angiograms,evolutionary algorithm,feature selection,K-nearest neighbor,stenosis classification
AI 理解论文
溯源树
样例
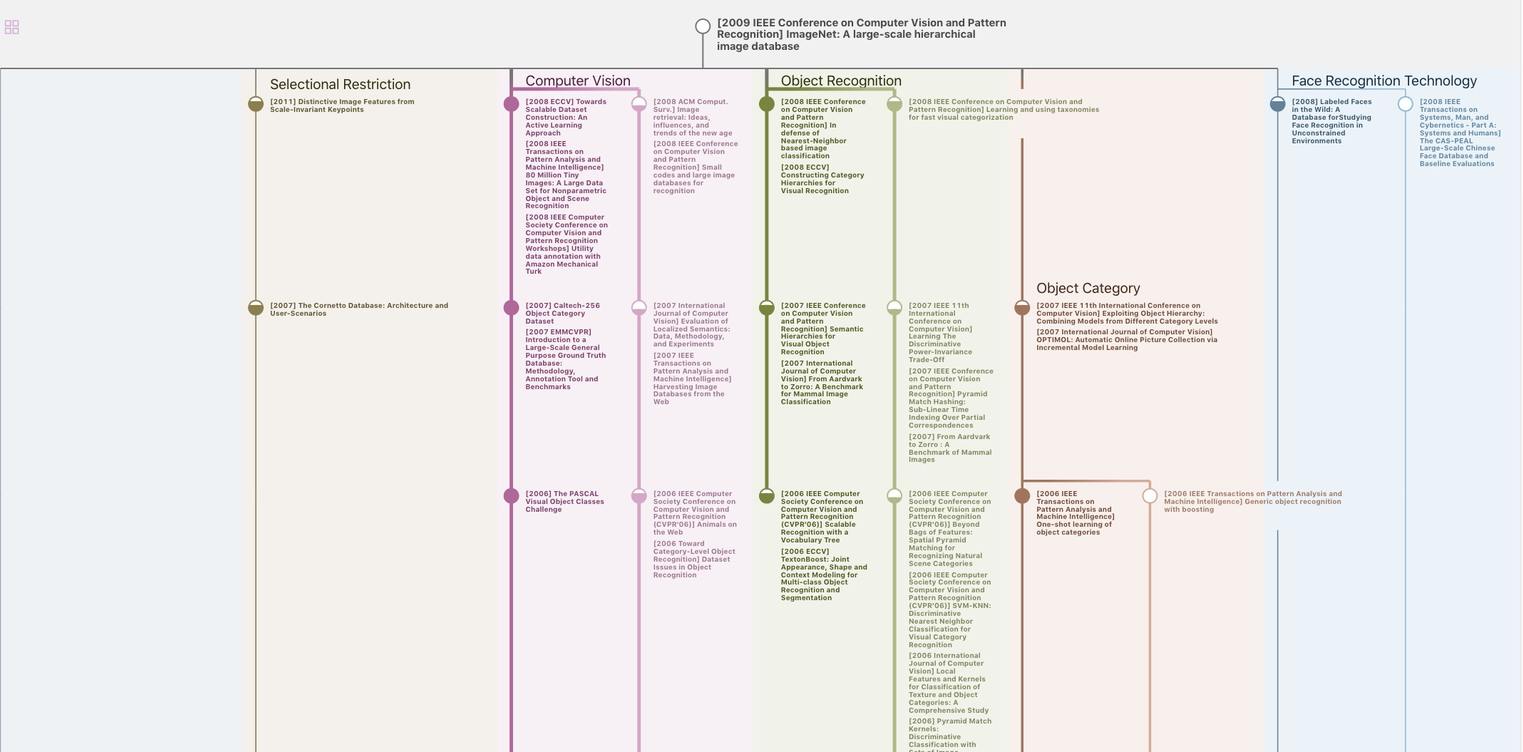
生成溯源树,研究论文发展脉络
Chat Paper
正在生成论文摘要