Learning Spatio-Temporal Radon Footprints for Assessment of Parkinson’s Dyskinesia
Electronics(2024)
摘要
Parkinson’s disease is a severe neurodegenerative disorder that leads to loss of control over various motor and mental functions. Its progression can be limited with medication, particularly through the use of levodopa. However, prolonged administration of levodopa often results in disorders independent of those caused by the disease. The detection of these disorders is based on the clinical examination of patients, through different type of activities and tasks, using the Unified Dyskinesia Rating Scale (UDysRS). In the present work, our aim is to develop a state-of-the-art assessment system for levodopa-induced dyskinesia (LID) using the joint coordinate data of a human skeleton body depicted on videotaped activities related to UDysRS. For this reason, we combine a robust mathematical method for encoding action sequences known as Spatio-temporal Radon Footprints (SRF) with a Convolutional Neural Network (CNN), in order to estimate dyskinesia’s ratings for six body parts. We introduce two different methodological approaches: Global SRF-CNN and Local SRF-CNN, based on the set of skeletal points used in the encoding scheme. A comparison between these approaches reveals that Local SRF-CNN demonstrates better performance than the Global one. Finally, Local SRF-CNN outperforms the state-of-the-art technique, on both tasks, for UDysRS dyskinesia assessment, using joint coordinate data of the human body, achieving an overall performance in mean RMSE value of 0.6198 for Drinking task and 0.4885 for Communication, compared to 0.6575 and 0.5175, respectively. This illustrates the ability of the proposed machine learning system to successfully assess LID.
更多查看译文
关键词
spatio-temporal radon footprints,convolutional neural networks,action recognition,Parkinson’s disease,unified dyskinesia rating scale
AI 理解论文
溯源树
样例
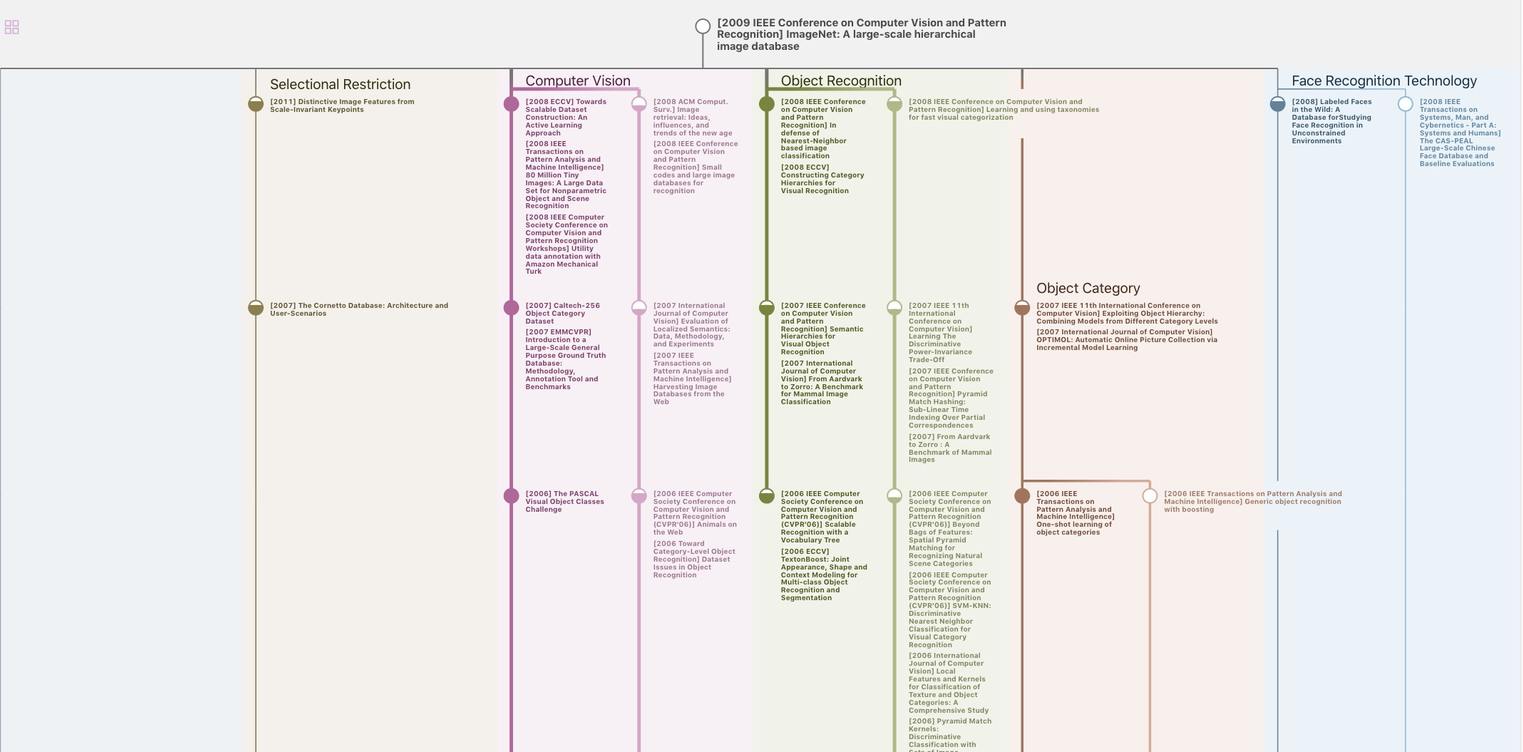
生成溯源树,研究论文发展脉络
Chat Paper
正在生成论文摘要