AutoSR4EO: An AutoML Approach to Super-Resolution for Earth Observation Images
REMOTE SENSING(2024)
摘要
Super-resolution (SR), a technique to increase the resolution of images, is a pre-processing step in the pipelines of applications of Earth observation (EO) data. The manual design and optimisation of SR models that are specific to every possible EO use case is a laborious process that creates a bottleneck for EO analysis. In this work, we develop an automated machine learning (AutoML) method to automate the creation of dataset-specific SR models. AutoML is the study of the automatic design of high-performance machine learning models. We present the following contributions. (i) We propose AutoSR4EO, an AutoML method for automatically constructing neural networks for SR. We design a search space based on state-of-the-art residual neural networks for SR and incorporate transfer learning. Our search space is extendable, making it possible to adapt AutoSR4EO to future developments in the field. (ii) We introduce a new real-world single-image SR (SISR) dataset, called SENT-NICFI. (iii) We evaluate the performance of AutoSR4EO on four different datasets against the performance of four state-of-the-art baselines and a vanilla AutoML SR method, with AutoSR4EO achieving the highest average ranking. Our results show that AutoSR4EO performs consistently well over all datasets, demonstrating that AutoML is a promising method for improving SR techniques for EO images.
更多查看译文
关键词
super-resolution,neural architecture search,automated machine learning
AI 理解论文
溯源树
样例
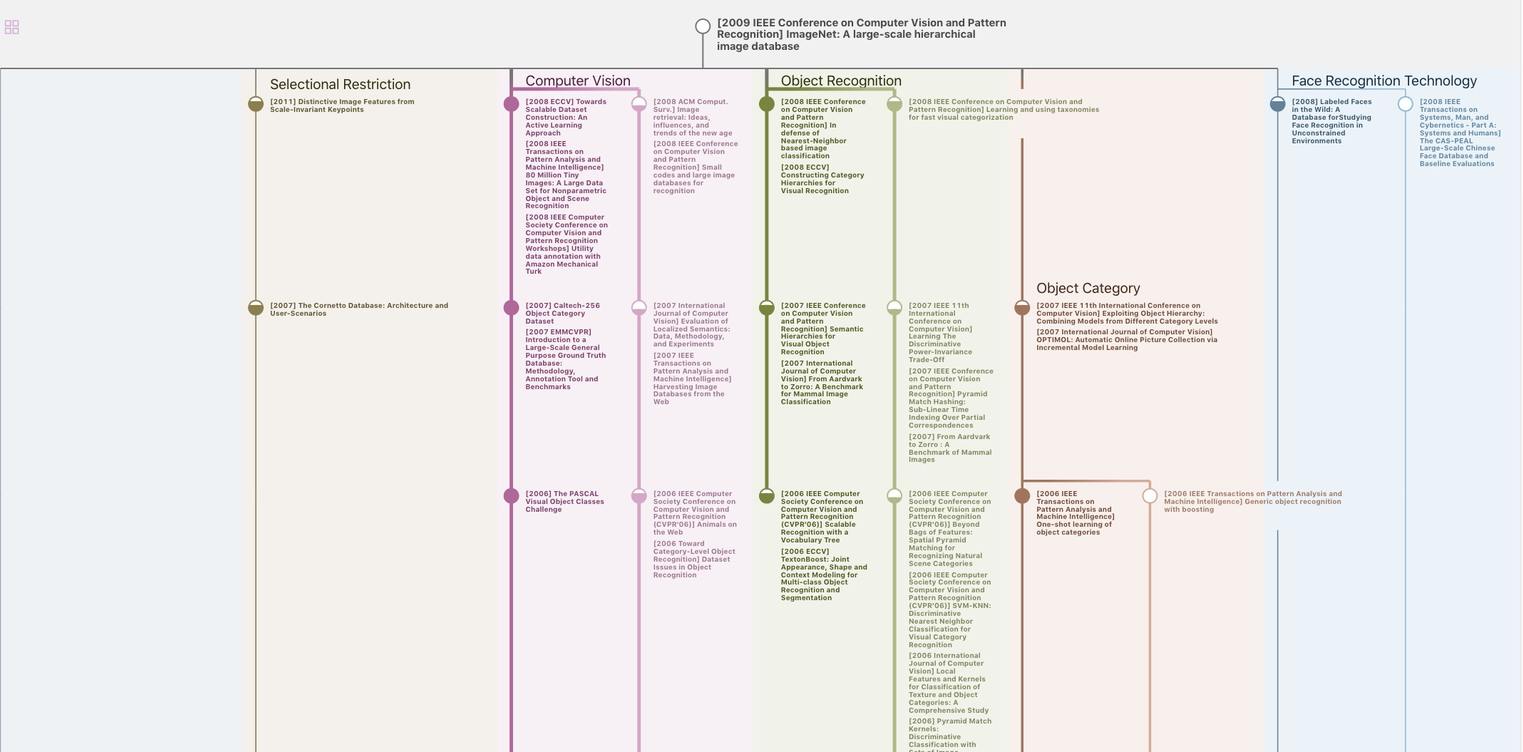
生成溯源树,研究论文发展脉络
Chat Paper
正在生成论文摘要