Double Augmentation: A Modal Transforming Method for Ship Detection in Remote Sensing Imagery
REMOTE SENSING(2024)
摘要
Ship detection in remote sensing images plays an important role in maritime surveillance. Recently, convolution neural network (CNN)-based methods have achieved state-of-the-art performance in ship detection. Even so, there are still two problems that remain in remote sensing. One is that the different modal images observed by multiple satellite sensors and the existing dataset cannot satisfy network-training requirements. The other is the false alarms in detection, as the ship target is usually faint in real view remote sensing images and many false-alarm targets can be detected in ocean backgrounds. To solve these issues, we propose a double augmentation framework for ship detection in cross-modal remote sensing imagery. Our method can be divided into two main steps: the front augmentation in the training process and the back augmentation verification in the detection process; the front augmentation uses a modal recognition network to reduce the modal difference in training and in using the detection network. The back augmentation verification uses batch augmentation and results clustering to reduce the rate of false-alarm detections and improve detection accuracy. Real-satellite-sensing experiments have been conducted to demonstrate the effectiveness of our method, which shows promising performance in quantitative evaluation metrics.
更多查看译文
关键词
remote sensing processing,maritime surveillance,ship detection,ocean engineering,cross-modal transforming
AI 理解论文
溯源树
样例
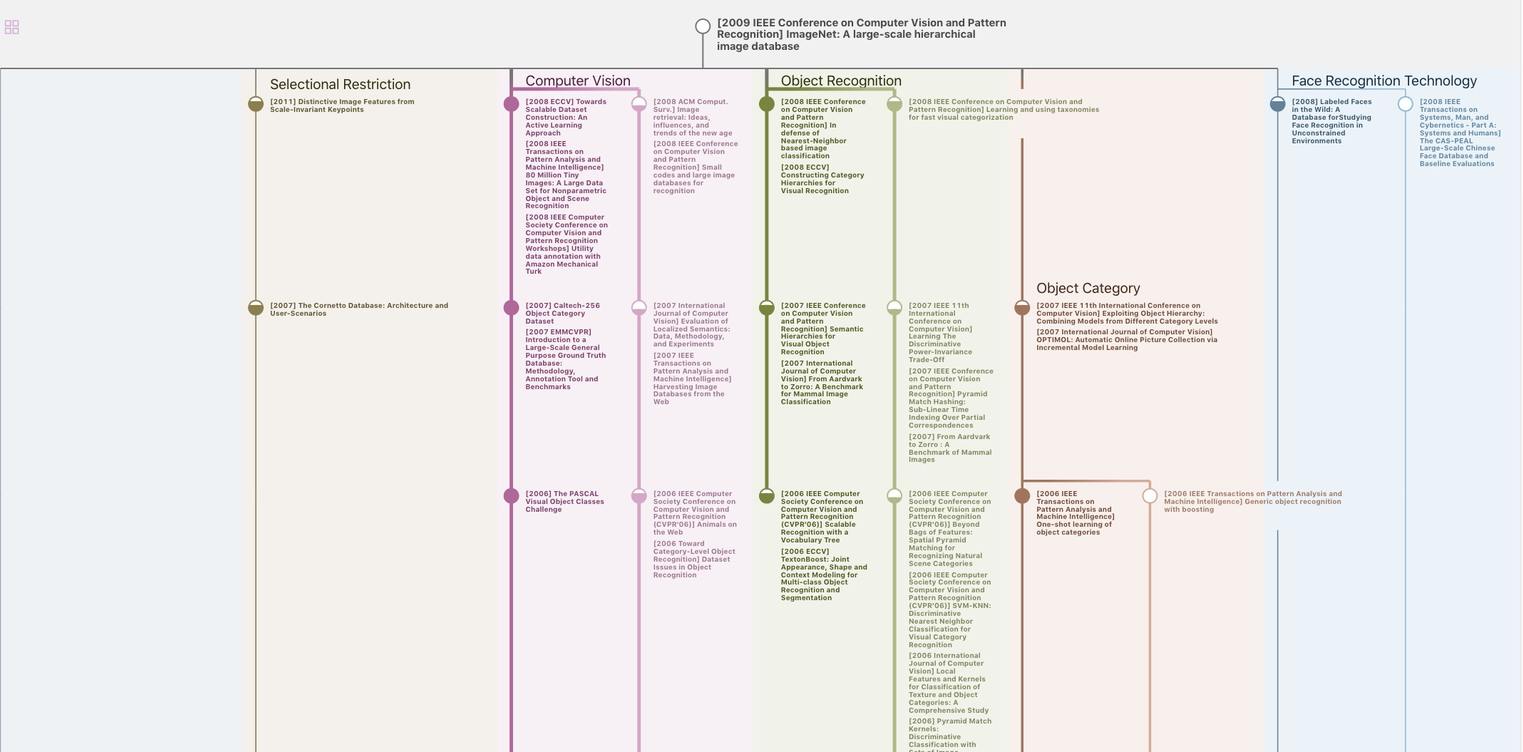
生成溯源树,研究论文发展脉络
Chat Paper
正在生成论文摘要