Efficient Small-Object Detection in Underwater Images Using the Enhanced YOLOv8 Network
APPLIED SCIENCES-BASEL(2024)
摘要
Underwater object detection plays a significant role in marine ecosystem research and marine species conservation. The improvement of related technologies holds practical significance. Although existing object-detection algorithms have achieved an excellent performance on land, they are not satisfactory in underwater scenarios due to two limitations: the underwater objects are often small, densely distributed, and prone to occlusion characteristics, and underwater embedded devices have limited storage and computational capabilities. In this paper, we propose a high-precision, lightweight underwater detector specifically optimizing for underwater scenarios based on the You Only Look Once Version 8 (YOLOv8) model. Firstly, we replace the Darknet-53 backbone of YOLOv8s with FasterNet-T0, reducing model parameters by 22.52%, FLOPS by 23.59%, and model size by 22.73%, achieving model lightweighting. Secondly, we add a Prediction Head for Small Objects, increase the number of channels for high-resolution feature map detection heads, and decrease the number of channels for low-resolution feature map detection heads. This results in a 1.2% improvement in small-object detection accuracy, while the remaining model parameters and memory consumption are nearly unchanged. Thirdly, we use Deformable ConvNets and Coordinate Attention in the neck part to enhance the accuracy in the detection of irregularly shaped and densely occluded small targets. This is achieved by learning convolution offsets from feature maps and emphasizing the regions of interest (RoIs). Our method achieves 52.12% AP on the underwater dataset UTDAC2020, with only 8.5 M parameters, 25.5 B FLOPS, and 17 MB model size. It surpasses the performance of large model YOLOv8l, at 51.69% AP, with 43.6 M parameters, 164.8 B FLOPS, and 84 MB model size. Furthermore, by increasing the input image resolution to 1280 x 1280 pixels, our model achieves 53.18% AP, making it the state-of-the-art (SOTA) model for the UTDAC2020 underwater dataset. Additionally, we achieve 84.4% mAP on the Pascal VOC dataset, with a substantial reduction in model parameters compared to previous, well-established detectors. The experimental results demonstrate that our proposed lightweight method retains effectiveness on underwater datasets and can be generalized to common datasets.
更多查看译文
关键词
YOLO,lightweight detector,small-object detection,deep learning
AI 理解论文
溯源树
样例
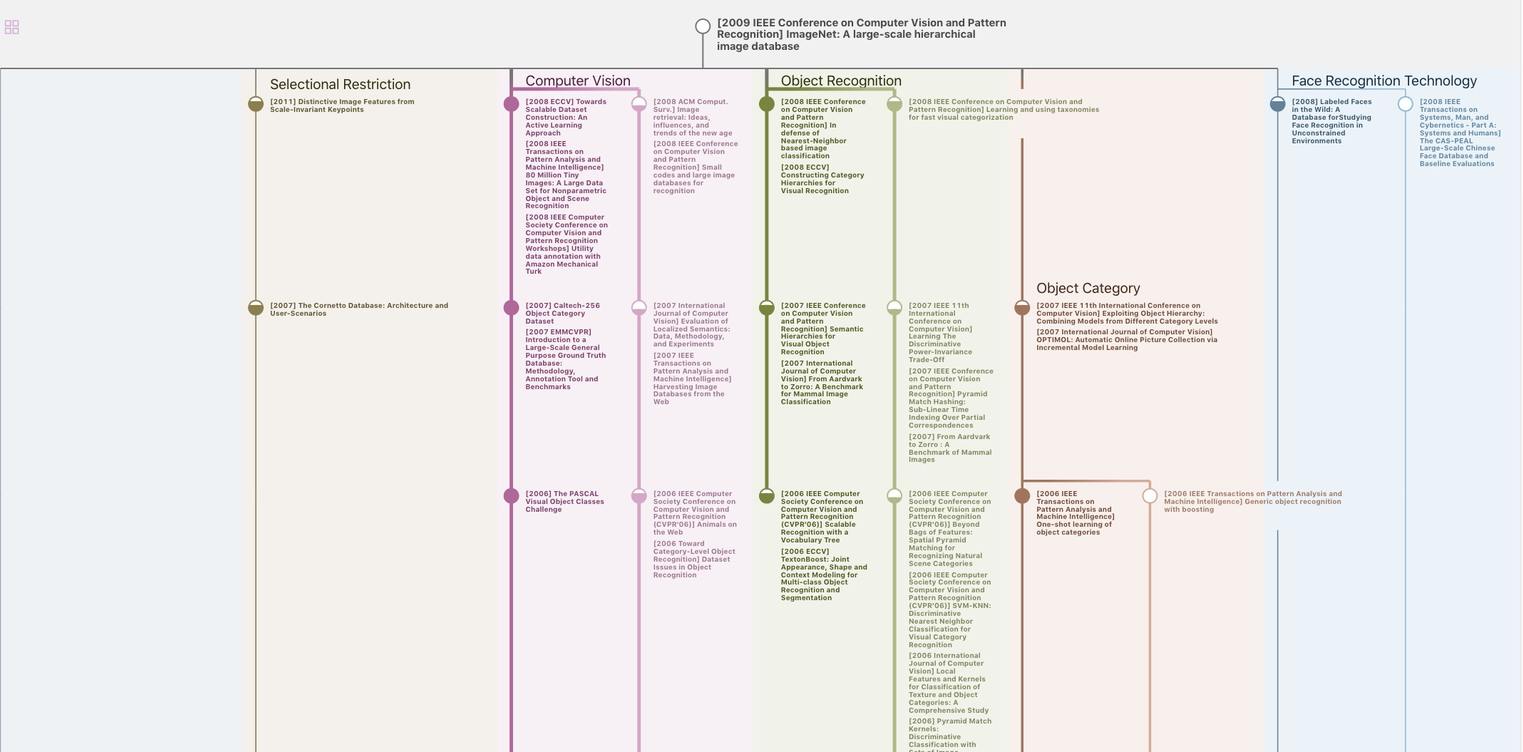
生成溯源树,研究论文发展脉络
Chat Paper
正在生成论文摘要