Basal Cell Carcinoma Diagnosis with Fusion of Deep Learning and Telangiectasia Features
Journal of Imaging Informatics in Medicine(2024)
摘要
In recent years, deep learning (DL) has been used extensively and successfully to diagnose different cancers in dermoscopic images. However, most approaches lack clinical inputs supported by dermatologists that could aid in higher accuracy and explainability. To dermatologists, the presence of telangiectasia, or narrow blood vessels that typically appear serpiginous or arborizing, is a critical indicator of basal cell carcinoma (BCC). Exploiting the feature information present in telangiectasia through a combination of DL-based techniques could create a pathway for both, improving DL results as well as aiding dermatologists in BCC diagnosis. This study demonstrates a novel “fusion” technique for BCC vs non-BCC classification using ensemble learning on a combination of (a) handcrafted features from semantically segmented telangiectasia (U-Net-based) and (b) deep learning features generated from whole lesion images (EfficientNet-B5-based). This fusion method achieves a binary classification accuracy of 97.2%, with a 1.3% improvement over the corresponding DL-only model, on a holdout test set of 395 images. An increase of 3.7% in sensitivity, 1.5% in specificity, and 1.5% in precision along with an AUC of 0.99 was also achieved. Metric improvements were demonstrated in three stages: (1) the addition of handcrafted telangiectasia features to deep learning features, (2) including areas near telangiectasia (surround areas), (3) discarding the noisy lower-importance features through feature importance. Another novel approach to feature finding with weak annotations through the examination of the surrounding areas of telangiectasia is offered in this study. The experimental results show state-of-the-art accuracy and precision in the diagnosis of BCC, compared to three benchmark techniques. Further exploration of deep learning techniques for individual dermoscopy feature detection is warranted.
更多查看译文
关键词
Basal cell carcinoma,Deep learning,Fusion,Telangiectasia,Transfer learning,Dermoscopy
AI 理解论文
溯源树
样例
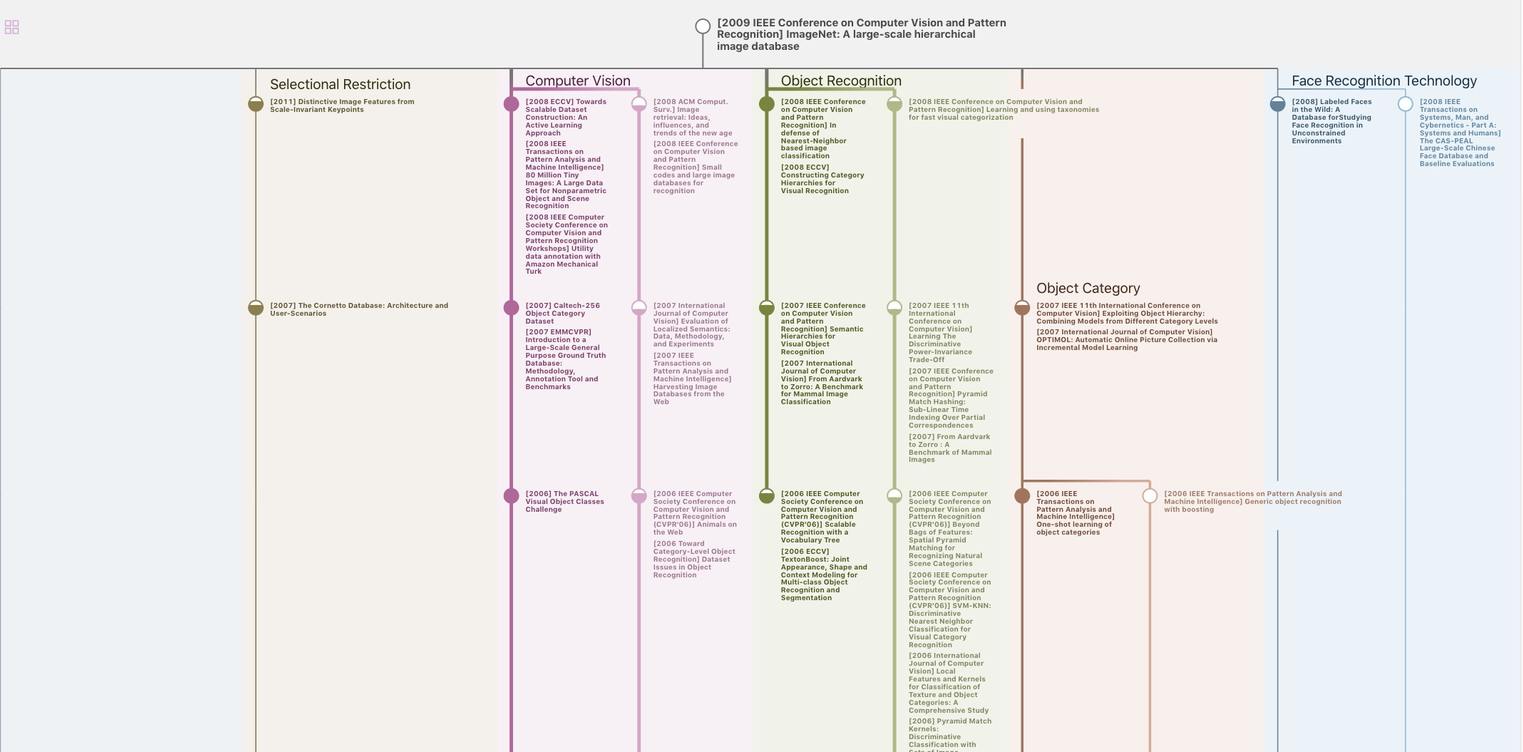
生成溯源树,研究论文发展脉络
Chat Paper
正在生成论文摘要