Enhancing Mass spectrometry-based tumor immunopeptide identification: machine learning filter leveraging HLA binding affinity, aliphatic index and retention time deviation
COMPUTATIONAL AND STRUCTURAL BIOTECHNOLOGY JOURNAL(2024)
摘要
Accurately identifying neoantigens is crucial for developing effective cancer vaccines and improving tumor immunotherapy. Mass spectrometry-based immunopeptidomics has emerged as a promising approach to identifying human leukocyte antigen (HLA) peptides presented on the surface of cancer cells, but false-positive identifications remain a significant challenge. In this study, liquid chromatography-tandem mass spectrometry-based proteomics and next-generation sequencing were utilized to identify HLA-presenting neoantigenic peptides resulting from non-synonymous single nucleotide variations in tumor tissues from 18 patients with renal cell carcinoma or pancreatic cancer. Machine learning was utilized to evaluate Mascot identifications through the prediction of MS/MS spectral consistency, and four descriptors for each candidate sequence: the max Mascot ion score, predicted HLA binding affinity, aliphatic index and retention time deviation, were selected as important features in filtering out identifications with inadequate fragmentation consistency. This suggests that incorporating rescoring filters based on peptide physicochemical characteristics could enhance the identification rate of MS-based immunopeptidomics compared to the traditional Mascot approach predominantly used for proteomics, indicating the potential for optimizing neoantigen identification pipelines as well as clinical applications.
更多查看译文
关键词
Immunopeptidomics,Mass spectrometry,Machine learning,Aliphatic index
AI 理解论文
溯源树
样例
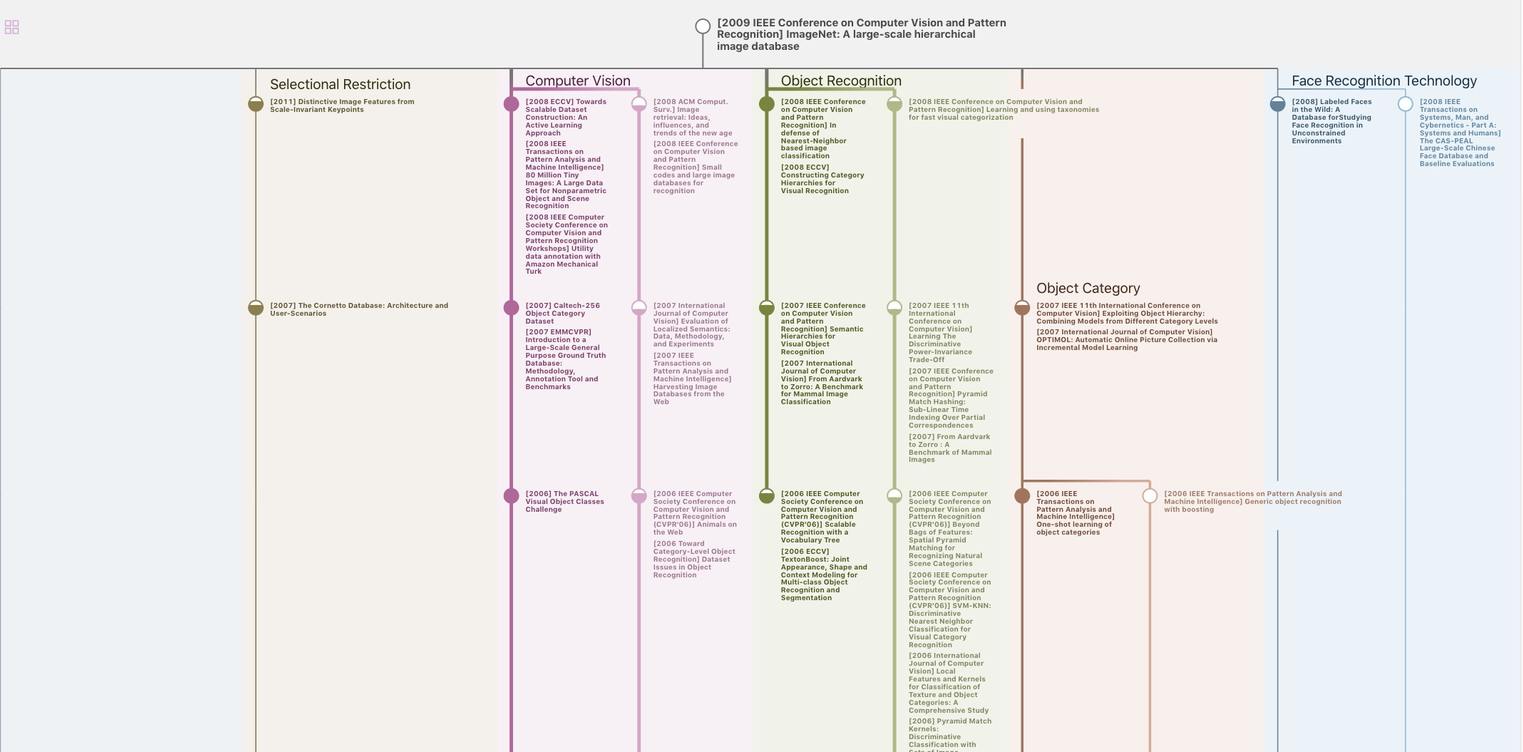
生成溯源树,研究论文发展脉络
Chat Paper
正在生成论文摘要