Extracting Building Footprints in SAR Images via Distilling Boundary Information From Optical Images.
IEEE Transactions on Geoscience and Remote Sensing(2024)
摘要
Buildings represent pivotal entities in remote sensing imagery for various applications such as urban planning and land resource management. Predominantly, methods for building footprint extraction in the literature focus on optical imagery with visual attributes that faithfully mirror the physical world. Nevertheless, the acquisition of high-quality optical images presents formidable challenges due to the susceptibility to illumination conditions and scene visibility. In contrast, synthetic aperture radar (SAR) images can be acquired in all-weather and all-time situations, unburdened by the aforementioned constraints. However, the coherent imaging mechanism engenders intricate complexities for building footprint extraction SAR images. To address this issue, this article introduces the boundary information distillation network (BIDNet) to improve the prediction accuracy in SAR images by distilling knowledge from optical images. The proposed approach adopts a teacher–student framework, featuring two customized components: the explicit distillation module (EDM) and the latent distillation module (LDM). Different from the conventional practice of directly aligning feature maps, BIDNet focuses on leveraging the more conspicuous boundary information in optical images. The EDM operates by simultaneously yielding a boundary map to emphasize the boundary area and assimilating the explicit low-level features of two modalities. The LDM represents the structural attributes within the high-level latent feature space and aligns the representations of the two modalities. Within this module, intrinsic self-correlations among features originating from boundary regions are encoded, and so are the cross-correlations established between features from boundary regions and alternative areas. The two modules also serve as the conduit for knowledge distillation (KD) from the teacher network to the student network, enabling the utilization of optical imagery for enhancing the building footprint extraction in SAR imagery. Extensive experiments demonstrate that our BIDNet achieves state-of-the-art performance on the Multi-Sensor All Weather Mapping (MSAW) dataset, outperforming the strong baseline by 4.3–7.2 points in F1-score and 4.9–8.0 points in IoU. The source code and trained models will be publicly available at
https://github.com/wangyx-chn/BIDNet
.
更多查看译文
关键词
Building footprint extraction,knowledge distillation (KD),semantic segmentation,synthetic aperture radar (SAR) image
AI 理解论文
溯源树
样例
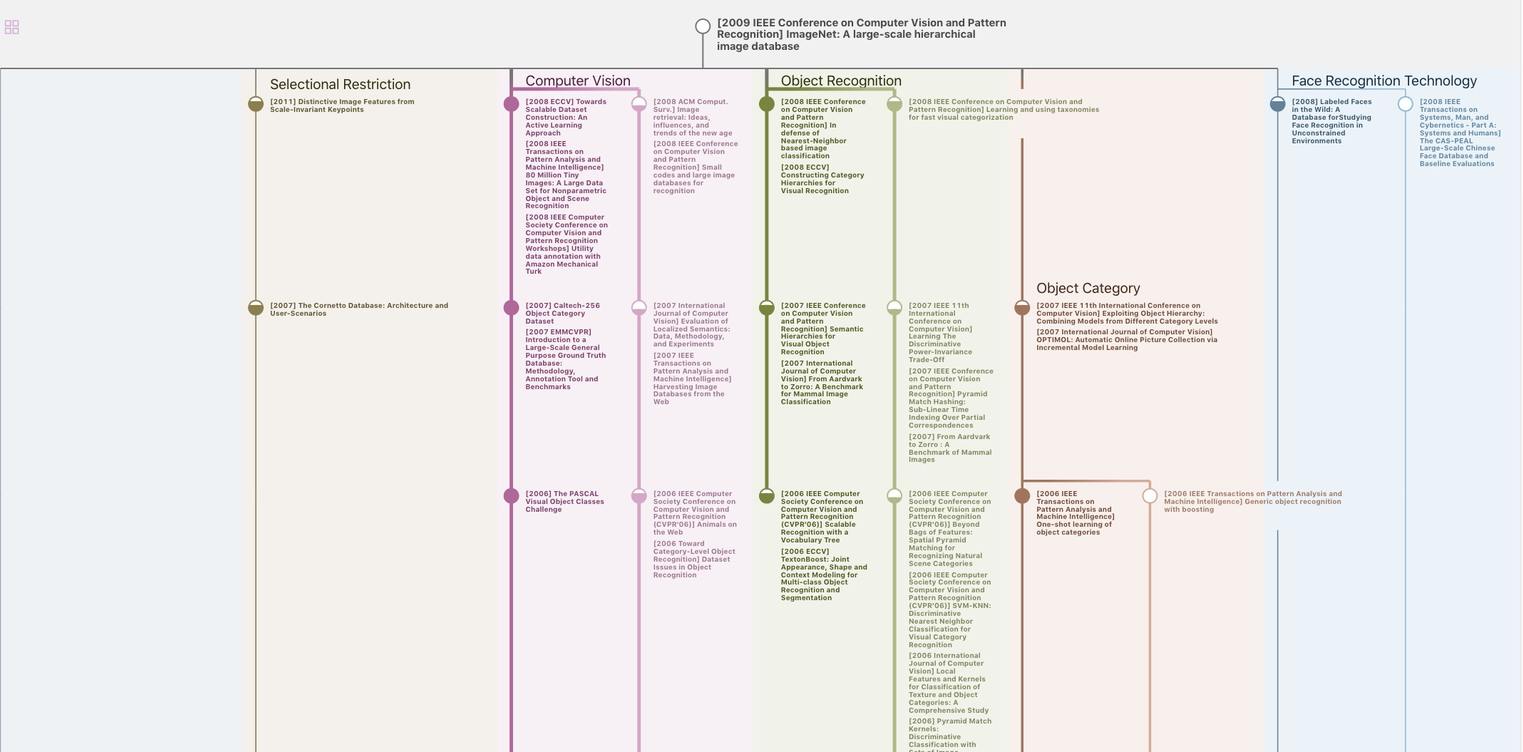
生成溯源树,研究论文发展脉络
Chat Paper
正在生成论文摘要