Myoelectric Prosthesis Control using Recurrent Convolutional Neural Network Regression Mitigates the Limb Position Effect
biorxiv(2024)
摘要
Although state-of-the-art myoelectric prostheses offer persons with upper limb amputation extensive movement capabilities, users have not been afforded a reliable means to control common movements required in daily living. Many proposed prosthesis controllers use pattern recognition, a method that learns and recognizes patterns of electromyographic (EMG) signals produced by the user's residual limb muscles to predict and execute device movements. Such control becomes unreliable in high limb positions\---|a problem known as the limb position effect. Pattern recognition often uses a classification algorithm; simple to implement, but limits user-initiated control to only one device movement at a time, at a single speed. To combat position-related control deficiencies and classification controller constraints, we developed and tested two recurrent convolutional neural network (RCNN) pattern recognition-based solutions: (1) an RCNN classification controller that uses EMG plus positional inertial measurement unit (IMU) signals to offer one-speed, sequential movement control; and (2) an RCNN regression controller that uses the same data capture technique to offer simultaneous control of multiple movements and device movement velocity. We assessed both RCNN controllers by comparing them to a commonly used linear discriminant analysis classification controller (LDA-Baseline). Participants without upper limb impairment were recruited to perform multipositional tasks while wearing a simulated prosthesis. Both RCNN classification and regression controllers showed improved functional task performance over LDA-Baseline, in 11 and 38 out of 115 metrics, respectively. This work contributes an RCNN regression-based controller that provides accurate, simultaneous, and proportional movements to EMG-based technologies including prostheses, exoskeletons, and even muscle-activated video games.
### Competing Interest Statement
The authors have declared no competing interest.
更多查看译文
AI 理解论文
溯源树
样例
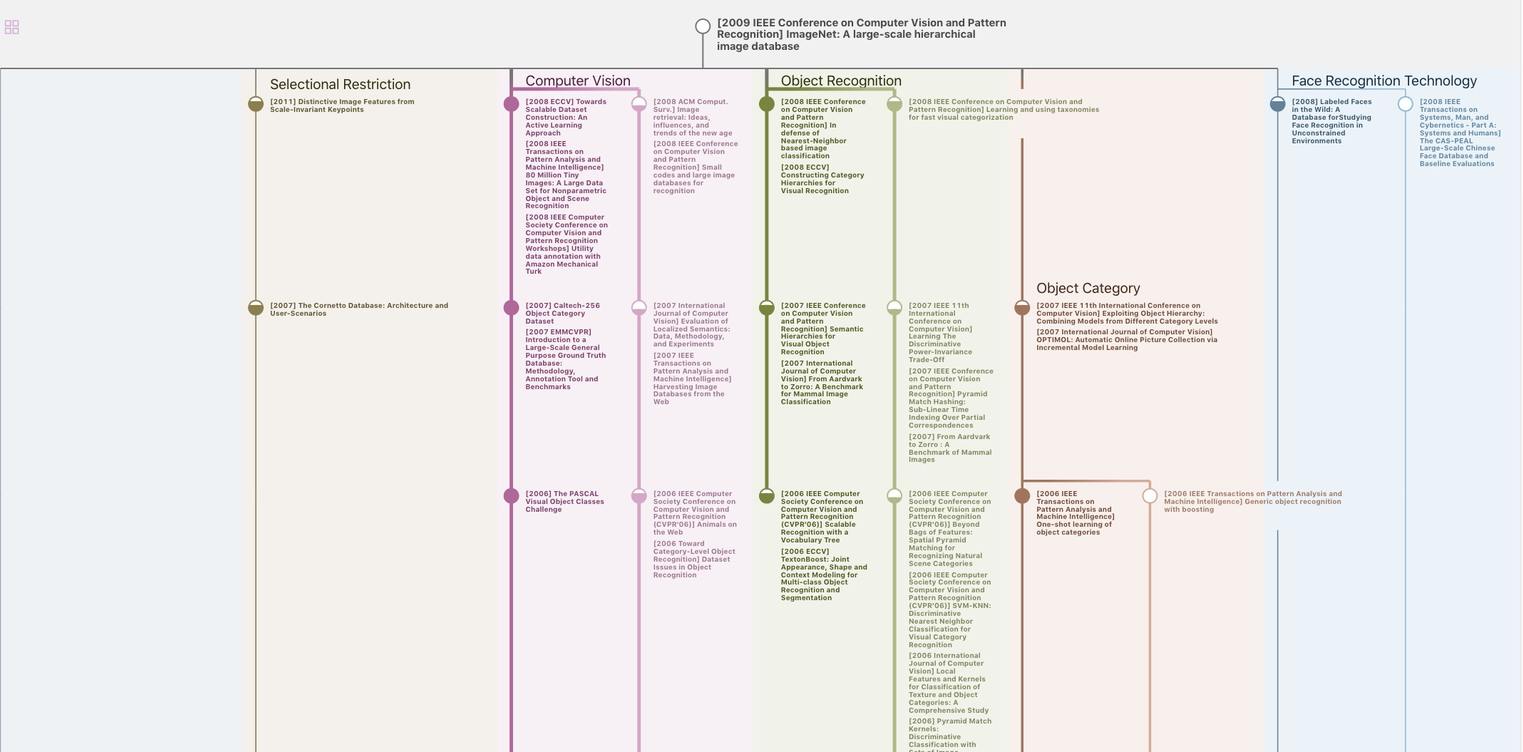
生成溯源树,研究论文发展脉络
Chat Paper
正在生成论文摘要