Bellman Conformal Inference: Calibrating Prediction Intervals For Time Series
CoRR(2024)
摘要
We introduce Bellman Conformal Inference (BCI), a framework that wraps around
any time series forecasting models and provides calibrated prediction
intervals. Unlike the existing methods, BCI is able to leverage multi-step
ahead forecasts and explicitly optimize the average interval lengths by solving
a one-dimensional stochastic control problem (SCP) at each time step. In
particular, we use the dynamic programming algorithm to find the optimal policy
for the SCP. We prove that BCI achieves long-term coverage under arbitrary
distribution shifts and temporal dependence, even with poor multi-step ahead
forecasts. We find empirically that BCI avoids uninformative intervals that
have infinite lengths and generates substantially shorter prediction intervals
on volatility forecasting problems when compared with existing methods.
更多查看译文
AI 理解论文
溯源树
样例
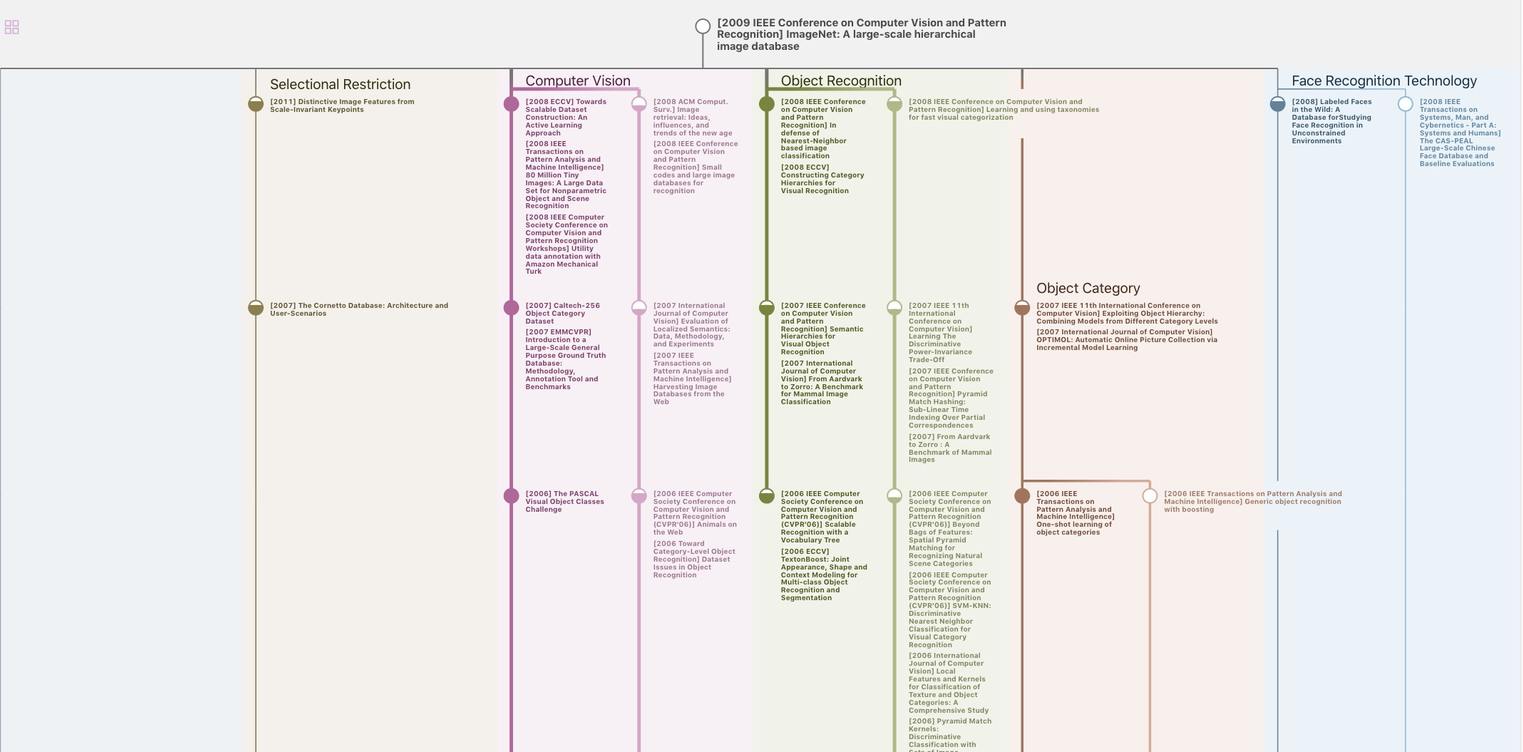
生成溯源树,研究论文发展脉络
Chat Paper
正在生成论文摘要