Physics Informed and Data Driven Simulation of Underwater Images via Residual Learning
CoRR(2024)
摘要
In general, underwater images suffer from color distortion and low contrast,
because light is attenuated and backscattered as it propagates through water
(differently depending on wavelength and on the properties of the water body).
An existing simple degradation model (similar to atmospheric image "hazing"
effects), though helpful, is not sufficient to properly represent the
underwater image degradation because there are unaccounted for and
non-measurable factors e.g. scattering of light due to turbidity of water,
reflective characteristics of turbid medium etc. We propose a deep
learning-based architecture to automatically simulate the underwater effects
where only a dehazing-like image formation equation is known to the network,
and the additional degradation due to the other unknown factors if inferred in
a data-driven way. We only use RGB images (because in real-time scenario depth
image is not available) to estimate the depth image. For testing, we have
proposed (due to the lack of real underwater image datasets) a complex image
formation model/equation to manually generate images that resemble real
underwater images (used as ground truth). However, only the classical image
formation equation (the one used for image dehazing) is informed to the
network. This mimics the fact that in a real scenario, the physics are never
completely known and only simplified models are known. Thanks to the ground
truth, generated by a complex image formation equation, we could successfully
perform a qualitative and quantitative evaluation of proposed technique,
compared to other purely data driven approaches
更多查看译文
AI 理解论文
溯源树
样例
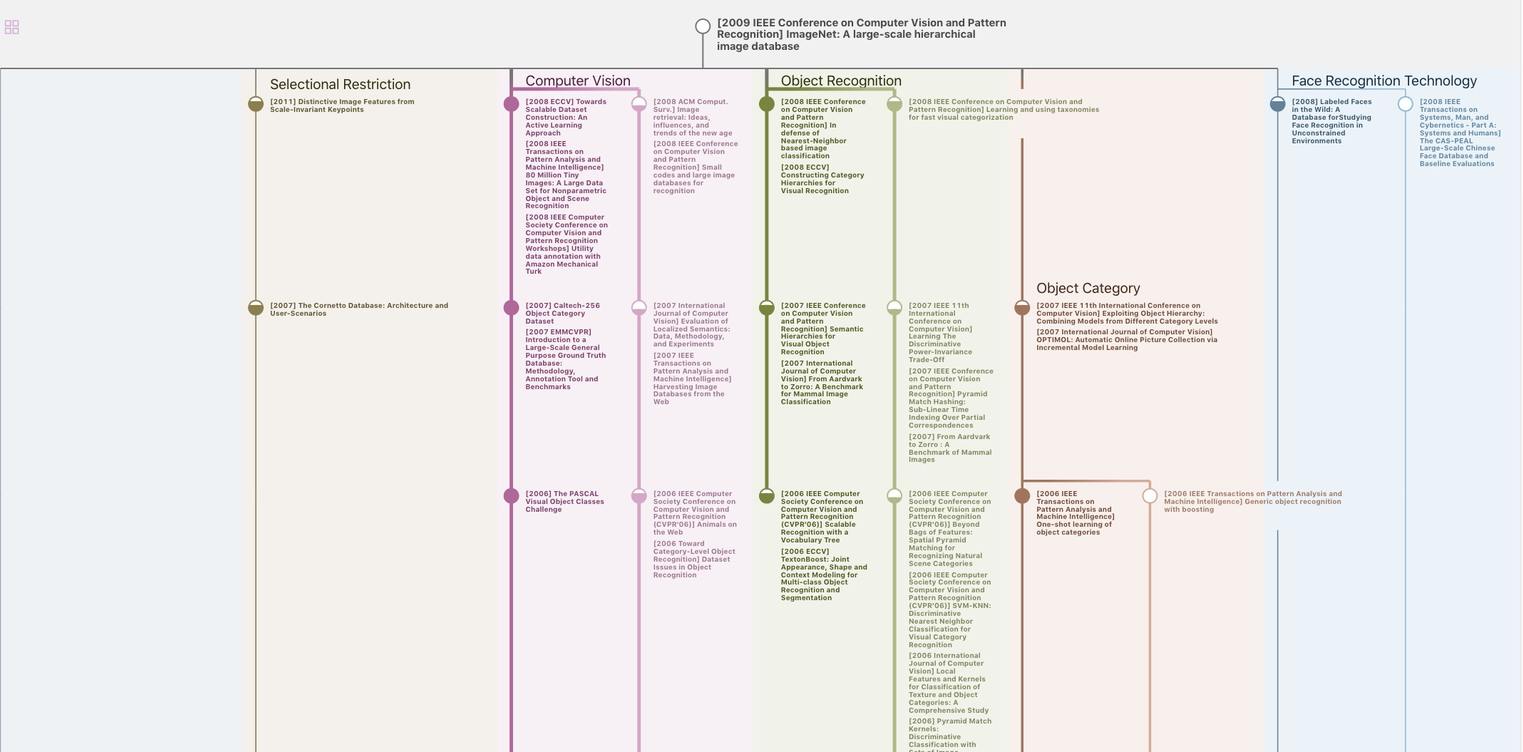
生成溯源树,研究论文发展脉络
Chat Paper
正在生成论文摘要