Perceived experts are prevalent and influential within an antivaccine community on Twitter
PNAS NEXUS(2024)
摘要
Perceived experts (i.e. medical professionals and biomedical scientists) are trusted sources of medical information who are especially effective at encouraging vaccine uptake. The role of perceived experts acting as potential antivaccine influencers has not been characterized systematically. We describe the prevalence and importance of antivaccine perceived experts by constructing a coengagement network of 7,720 accounts based on a Twitter data set containing over 4.2 million posts from April 2021. The coengagement network primarily broke into two large communities that differed in their stance toward COVID-19 vaccines, and misinformation was predominantly shared by the antivaccine community. Perceived experts had a sizable presence across the coengagement network, including within the antivaccine community where they were 9.8% of individual, English-language users. Perceived experts within the antivaccine community shared low-quality (misinformation) sources at similar rates and academic sources at higher rates compared to perceived nonexperts in that community. Perceived experts occupied important network positions as central antivaccine users and bridges between the antivaccine and provaccine communities. Using propensity score matching, we found that perceived expertise brought an influence boost, as perceived experts were significantly more likely to receive likes and retweets in both the antivaccine and provaccine communities. There was no significant difference in the magnitude of the influence boost for perceived experts between the two communities. Social media platforms, scientific communications, and biomedical organizations may focus on more systemic interventions to reduce the impact of perceived experts in spreading antivaccine misinformation.
更多查看译文
关键词
antivaccine,public health,social media,misinformation
AI 理解论文
溯源树
样例
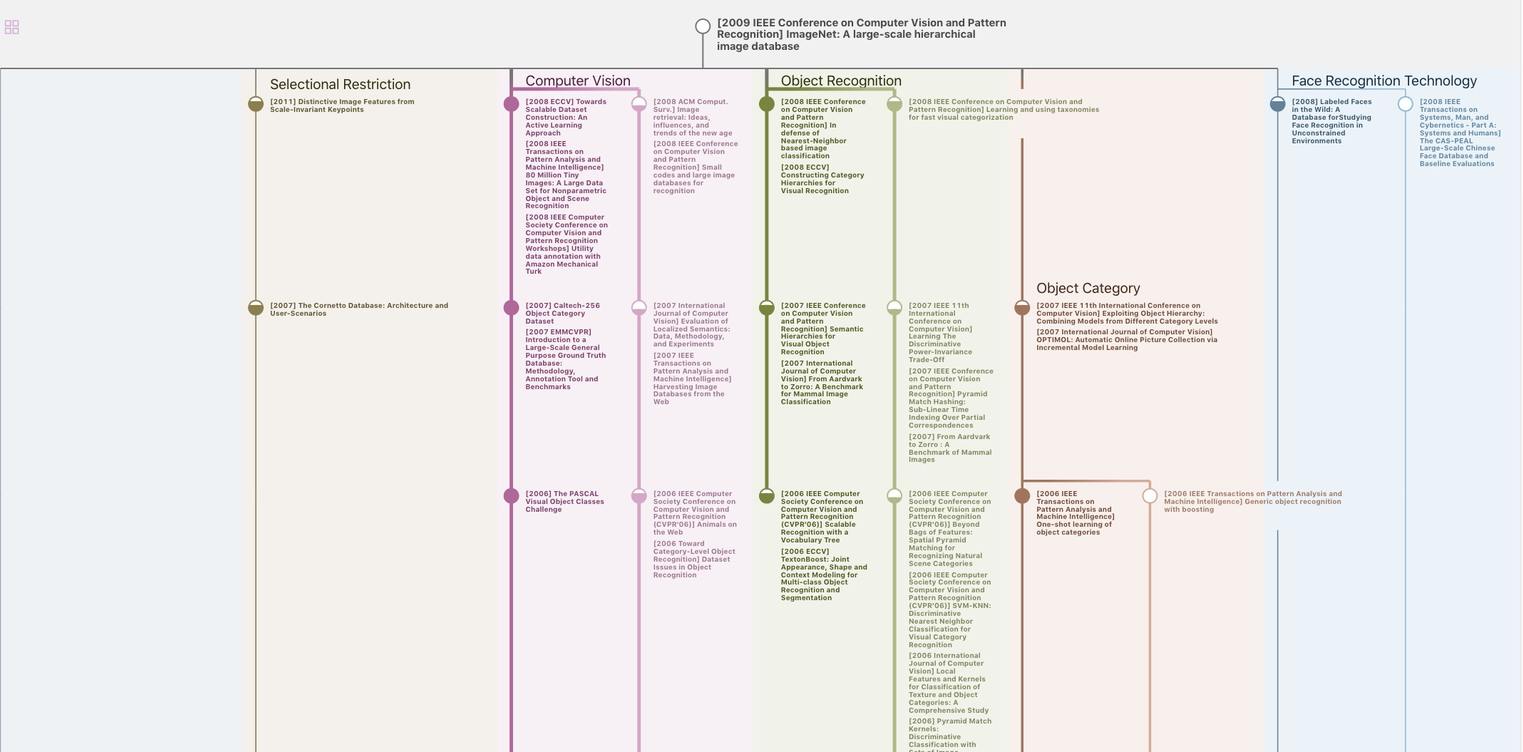
生成溯源树,研究论文发展脉络
Chat Paper
正在生成论文摘要