Multicentric clinical evaluation of a computed tomography-based fully automated deep neural network for aortic maximum diameter and volumetric measurements.
Journal of vascular surgery(2024)
摘要
OBJECTIVE:This study aims to evaluate a fully automatic deep learning-based method (augmented radiology for vascular aneurysm [ARVA]) for aortic segmentation and simultaneous diameter and volume measurements.
METHODS:A clinical validation dataset was constructed from preoperative and postoperative aortic computed tomography angiography (CTA) scans for assessing these functions. The dataset totaled 350 computed tomography angiography scans from 216 patients treated at two different hospitals. ARVA's ability to segment the aorta into seven morphologically based aortic segments and measure maximum outer-to-outer wall transverse diameters and compute volumes for each was compared with the measurements of six experts (ground truth) and thirteen clinicians.
RESULTS:Ground truth (experts') measurements of diameters and volumes were manually performed for all aortic segments. The median absolute diameter difference between ground truth and ARVA was 1.6 mm (95% confidence interval [CI], 1.5-1.7; and 1.6 mm [95% CI, 1.6-1.7]) between ground truth and clinicians. ARVA produced measurements within the clinical acceptable range with a proportion of 85.5% (95% CI, 83.5-86.3) compared with the clinicians' 86.0% (95% CI, 83.9-86.0). The median volume similarity error ranged from 0.93 to 0.95 in the main trunk and achieved 0.88 in the iliac arteries.
CONCLUSIONS:This study demonstrates the reliability of a fully automated artificial intelligence-driven solution capable of quick aortic segmentation and analysis of both diameter and volume for each segment.
更多查看译文
AI 理解论文
溯源树
样例
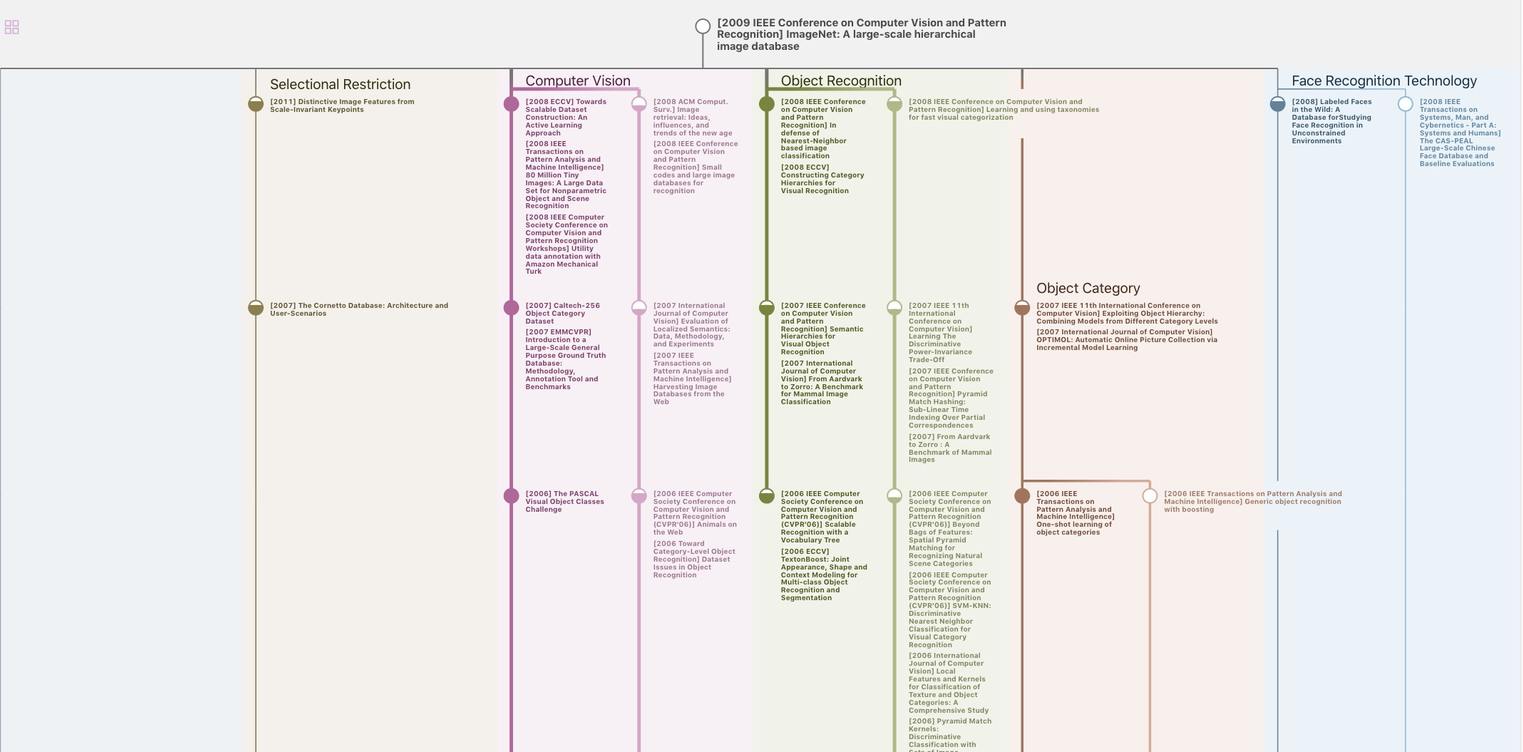
生成溯源树,研究论文发展脉络
Chat Paper
正在生成论文摘要