MIT: Mutual Information Topic Model for Diverse Topic Extraction
IEEE TRANSACTIONS ON NEURAL NETWORKS AND LEARNING SYSTEMS(2024)
摘要
To automatically mine structured semantic topics from text, neural topic modeling has arisen and made some progress. However, most existing work focuses on designing a mechanism to enhance topic coherence but sacrificing the diversity of the extracted topics. To address this limitation, we propose the first neural-based topic modeling approach purely based on mutual information maximization, called the mutual information topic (MIT) model, in this article. The proposed MIT significantly improves topic diversity by maximizing the mutual information between word distribution and topic distribution. Meanwhile, MIT also utilizes Dirichlet prior in latent topic space to ensure the quality of mined topics. The experimental results on three publicly benchmark text corpora show that MIT could extract topics with higher coherence values (considering four topic coherence metrics) than competitive approaches and has a significant improvement on topic diversity metric. Besides, our experiments prove that the proposed MIT converges faster and more stable than adversarial-neural topic models.
更多查看译文
关键词
Mutual information,Coherence,Training,Semantics,Telecommunications,Computer science,Visualization,Generative models,mutual information (MI),neural topic modeling,text mining,unsupervised learning
AI 理解论文
溯源树
样例
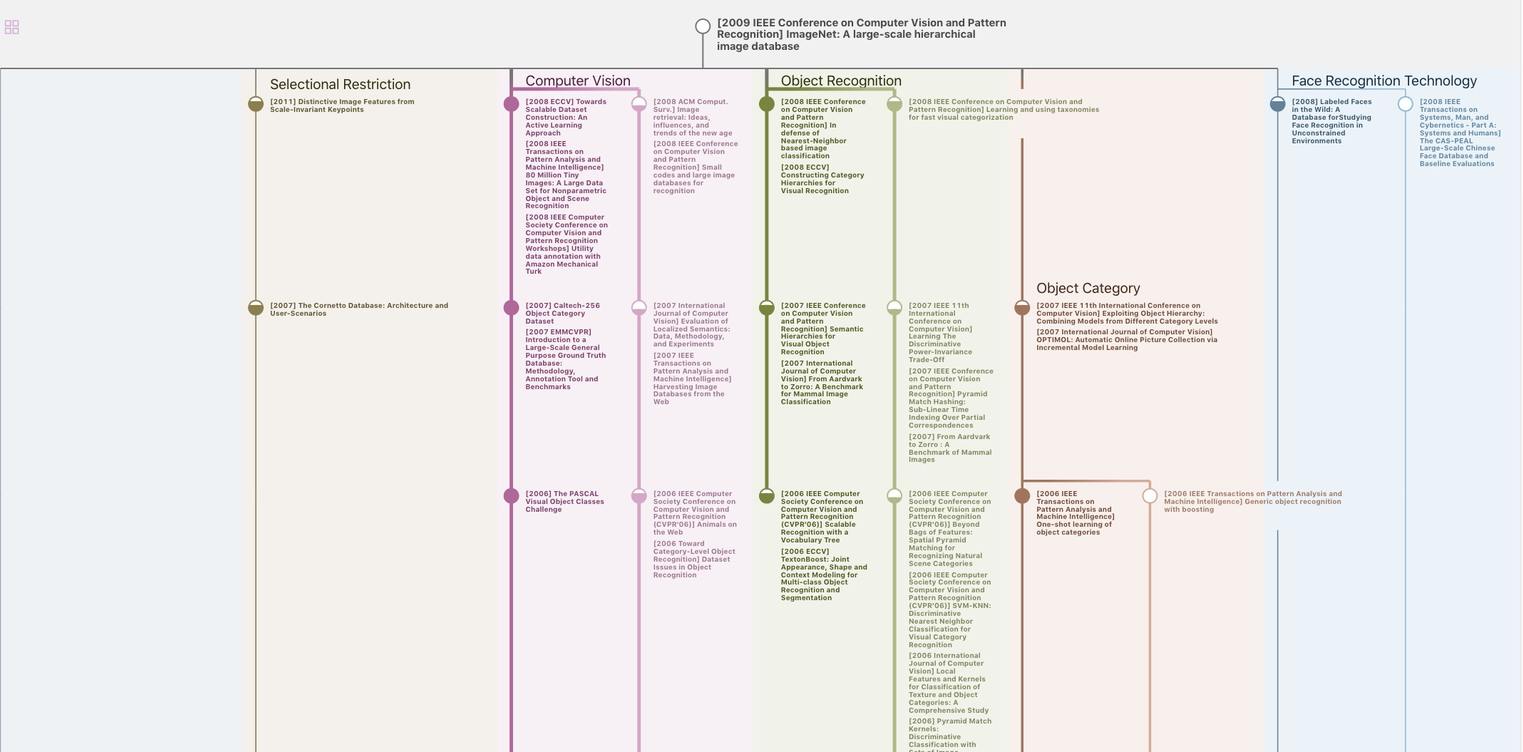
生成溯源树,研究论文发展脉络
Chat Paper
正在生成论文摘要