Sampled-Data State Estimation for LSTM
IEEE TRANSACTIONS ON NEURAL NETWORKS AND LEARNING SYSTEMS(2024)
摘要
This article first introduces a sampled-data state estimator design method for continuous-time long short-term memory (LSTM) neural networks with irregularly sampled output. To this end, the structure of the LSTM is addressed to obtain its dynamic equation. As a result, the LSTM neural network is modeled as a continuous-time linear parameter-varying system that is dependent on the gate units. For this system, the sampled-data Luenberger-and Arcak-type state estimator design methods are presented in terms of linear matrix inequalities (LMIs) by using the properties of the gate units. Lastly, the proposed method not only provides a numerical example for analyzing absolute stability but also demonstrates it in practice by applying a pre-trained behavior generation model of a robot manipulator.
更多查看译文
关键词
Biological neural networks,Neural networks,State estimation,Recurrent neural networks,Logic gates,Design methodology,Mathematical models,Linear matrix inequalities (LMIs),long short-term memory (LSTM),neural networks,sampled-data system,state estimation
AI 理解论文
溯源树
样例
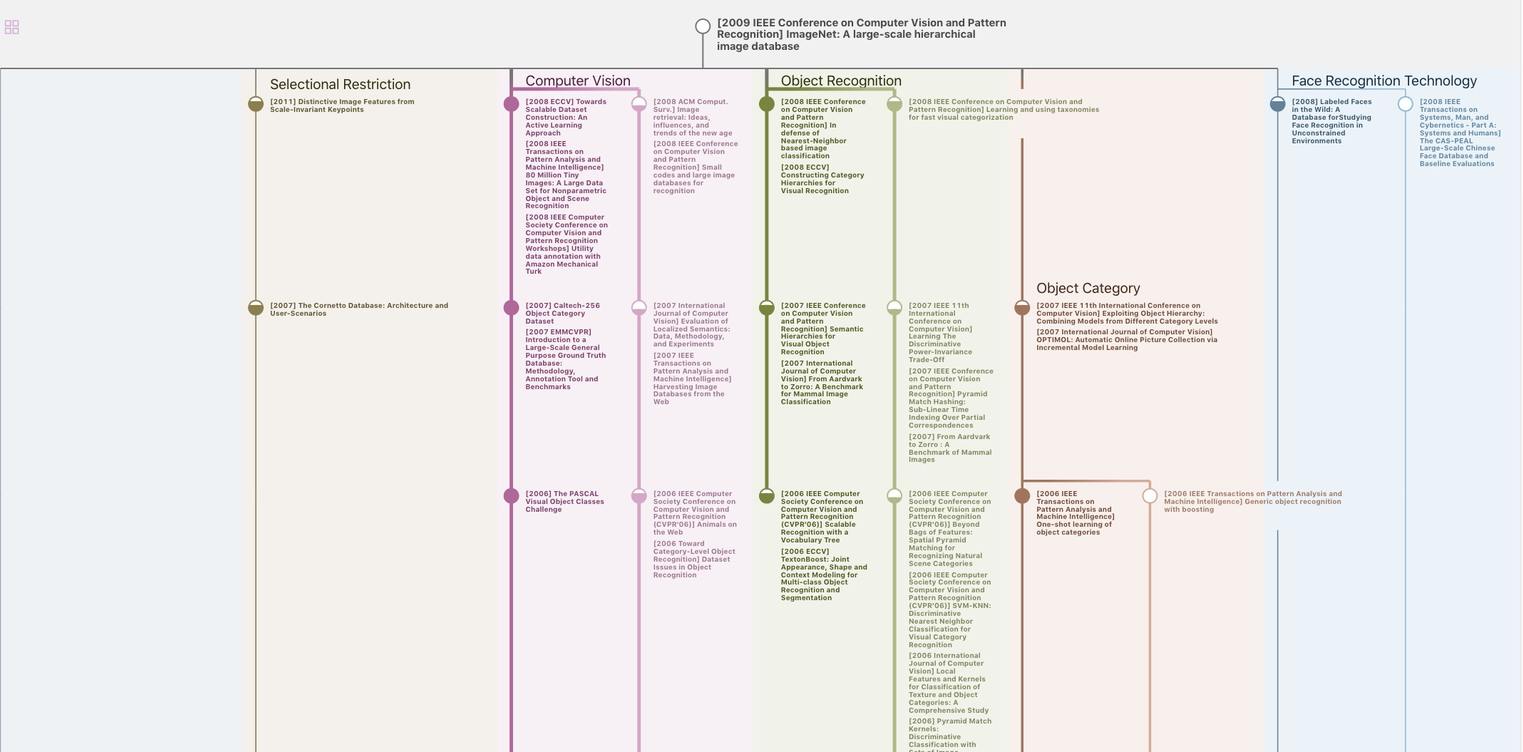
生成溯源树,研究论文发展脉络
Chat Paper
正在生成论文摘要