RAVAS: Interference-Aware Model Selection and Resource Allocation for Live Edge Video Analytics.
2023 IEEE/ACM Symposium on Edge Computing (SEC)(2023)
摘要
Numerous edge applications that rely on video analytics demand precise, low-latency processing of multiple video streams from cameras. When these cameras are mobile, such as when mounted on a car or a robot, the processing load on the shared edge GPU can vary considerably. Provisioning the edge with GPUs for the worst-case load can be expensive and, for many applications, not feasible. In this paper, we introduce RAVAS, a Real-time Adaptive stream Video Analytics System that enables efficient edge GPU sharing for processing streams from various mobile cameras. RAVAS uses Q-Learning to choose between a set of Deep Neural Network (DNN) models with varying accuracy and processing requirements based on the current GPU utilization and workload. RAVAS employs an innovative resource allocation strategy to mitigate interference during concurrent GPU execution. Compared to state-of-the-art approaches, our results show that RAVAS incurs 57% less compute overhead, achieves 41% improvement in latency, and 43% savings in total GPU usage for a single video stream. Processing multiple concurrent video streams results in up to 99% and 40% reductions in latency and overall GPU usage, respectively, while meeting the accuracy constraints.
更多查看译文
关键词
Edge Video Analytics,Model Selection,Resource Allocation,Interference-aware GPU Multiplexing
AI 理解论文
溯源树
样例
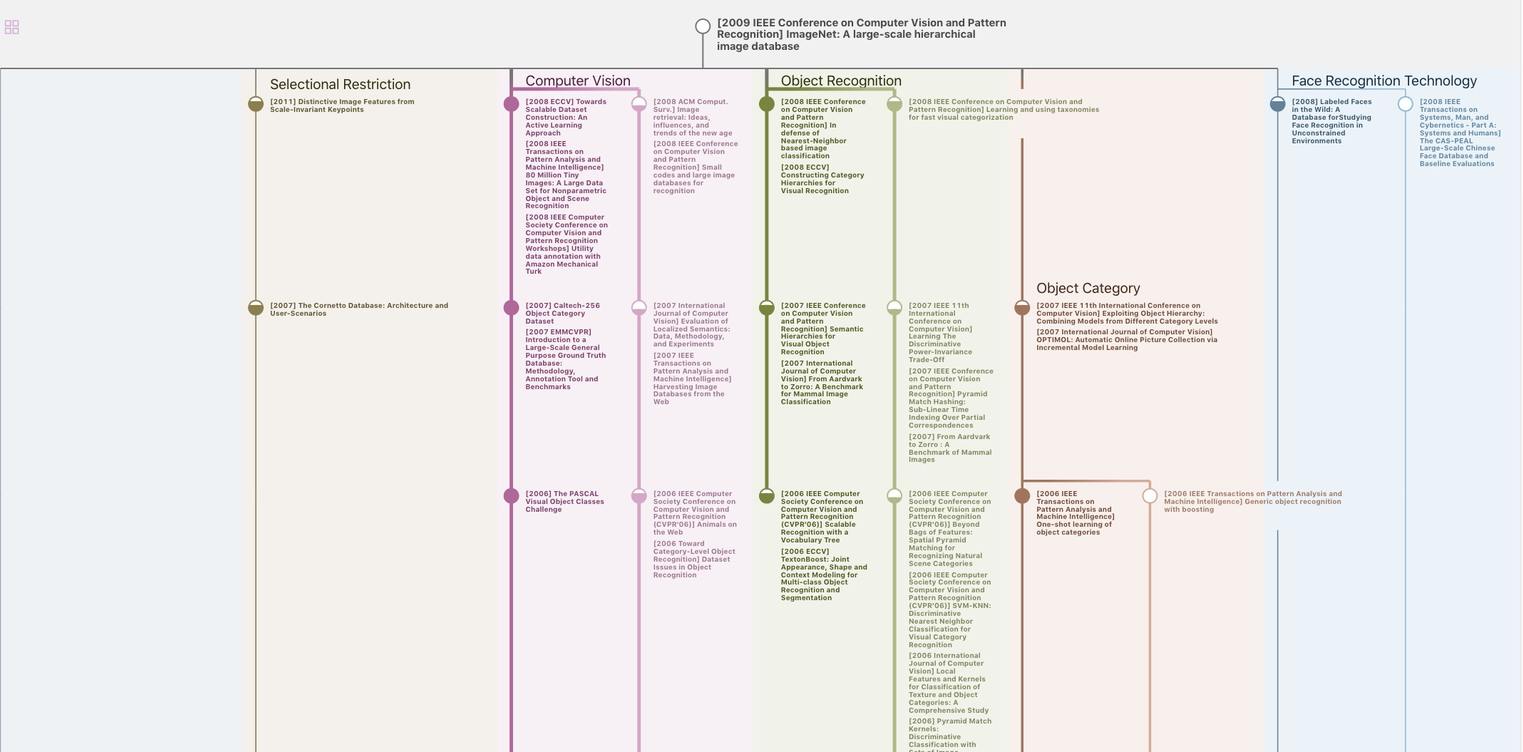
生成溯源树,研究论文发展脉络
Chat Paper
正在生成论文摘要