Empowering Trustworthy Client Selection in Edge Federated Learning Leveraging Reinforcement Learning
2023 IEEE/ACM SYMPOSIUM ON EDGE COMPUTING, SEC 2023(2023)
摘要
Federated learning (FL) is a promising approach for training AI models across multiple clients in Edge Computing (EC), without sharing raw local data. By enabling local training and aggregating updates into a global model, FL maintains privacy while facilitating collaborative learning. Nevertheless, FL encounters several challenges, including trustworthy client participation, inefficient model aggregation due to client with malicious or less accurate model. In this paper, we propose a trustworthy FL method incorporating Q-learning, trust, and reputation mechanisms, enhancing model accuracy and fairness. This method promotes client participation, mitigates malicious attacks' impact, and ensures fair model distribution. Inspired by reinforcement learning, the Q-learning algorithm optimizes client selection using the Bellman equation, enabling the server to balance exploration and exploitation for improved system performance. Furthermore, we explored the advantages of peer-to-peer FL settings. Extensive experimentation demonstrates our proposed trustworthy FL approach's effectiveness in achieving high learning accuracy while ensuring fairness across clients and maintaining efficient client selection. Our results reveal significant improvements in model performance, convergence speed, and generalization.
更多查看译文
关键词
Federated Learning,Reinforcement learning,Trust,Reputation,Privacy,Edge Computing
AI 理解论文
溯源树
样例
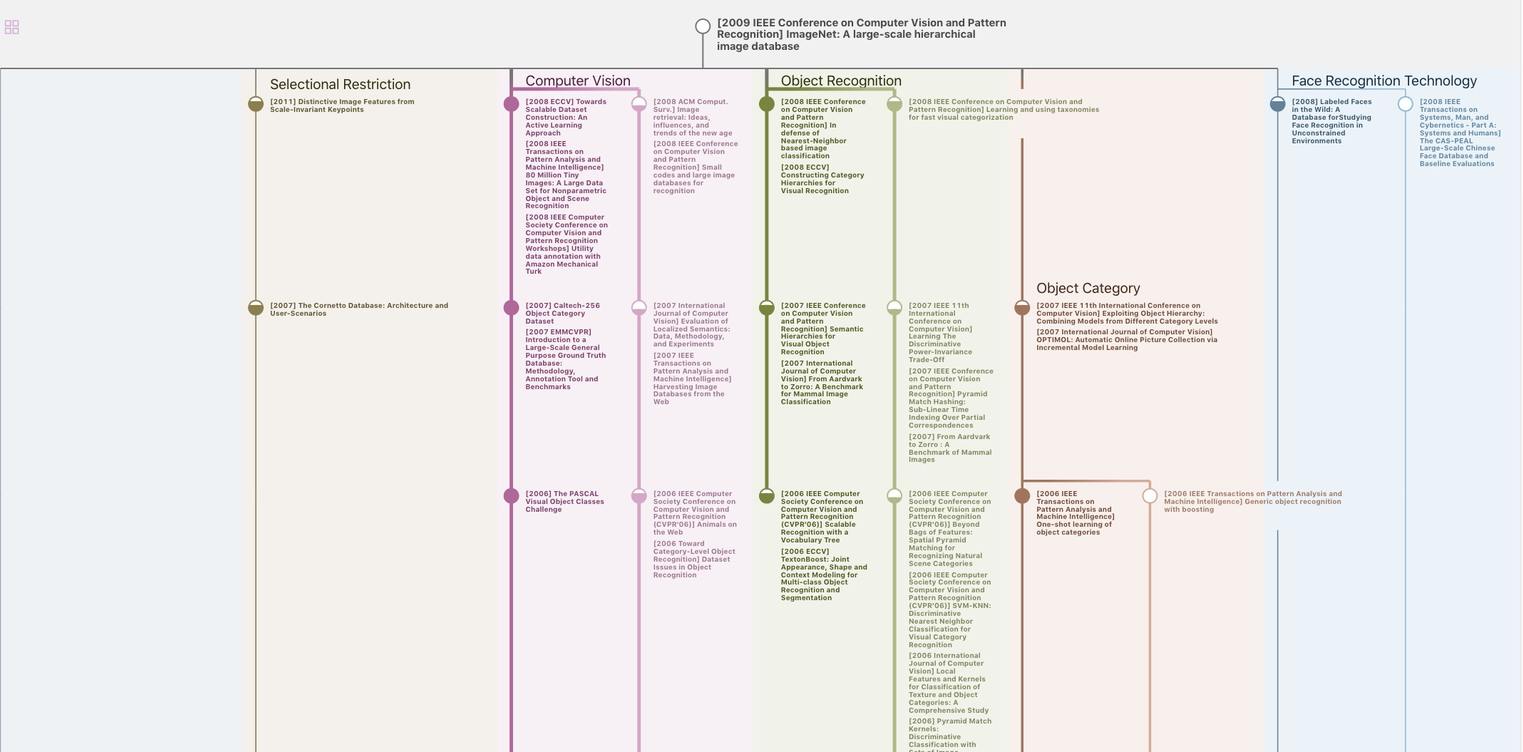
生成溯源树,研究论文发展脉络
Chat Paper
正在生成论文摘要