Distributed Tracking and Verifying: A Real-Time and High-Accuracy Visual Tracking Edge Computing Framework for Internet of Things
2023 IEEE/ACM SYMPOSIUM ON EDGE COMPUTING, SEC 2023(2023)
摘要
We observe that accurate and fast tracking in Internet of Things (IoT) devices is still a challenging problem. Several deep learning models have emerged which provide higher accuracy scores in object detection and tracking, however, due to their computationally expensive nature they are not useful in enabling real-time tracking at IoT devices. Correlation filters have emerged to show better speed in real-time tracking and provide good tracking results in cases of occlusion, rotation, illumination and other distractions. To get better speed as well as accuracy we use combination of correlation filter and deep learning methods. We propose a distributed tracking and verifying (DTAV) framework. Specifically, we run two object tracking algorithms, one on the client and another on the server. The algorithm run on the client is referred to as the Tracker, which is based on correlation filter and runs easily in real-time. The server hosts the verifier algorithm which performs high accuracy verification. Thus, while the client performs fast object tracking, the server's tracking algorithm verifies the output and corrects the server whenever required to maintain the accuracy of the model. We present our edge computing-based framework and discuss the motivation, system setup and series of experiments performed for the framework and present our experimental results. DTAV achieved 7.78% improvement on accuracy and 15% improvement in FPS.
更多查看译文
关键词
Edge Computing,Distributed Inference,Visual object tracking for IoT
AI 理解论文
溯源树
样例
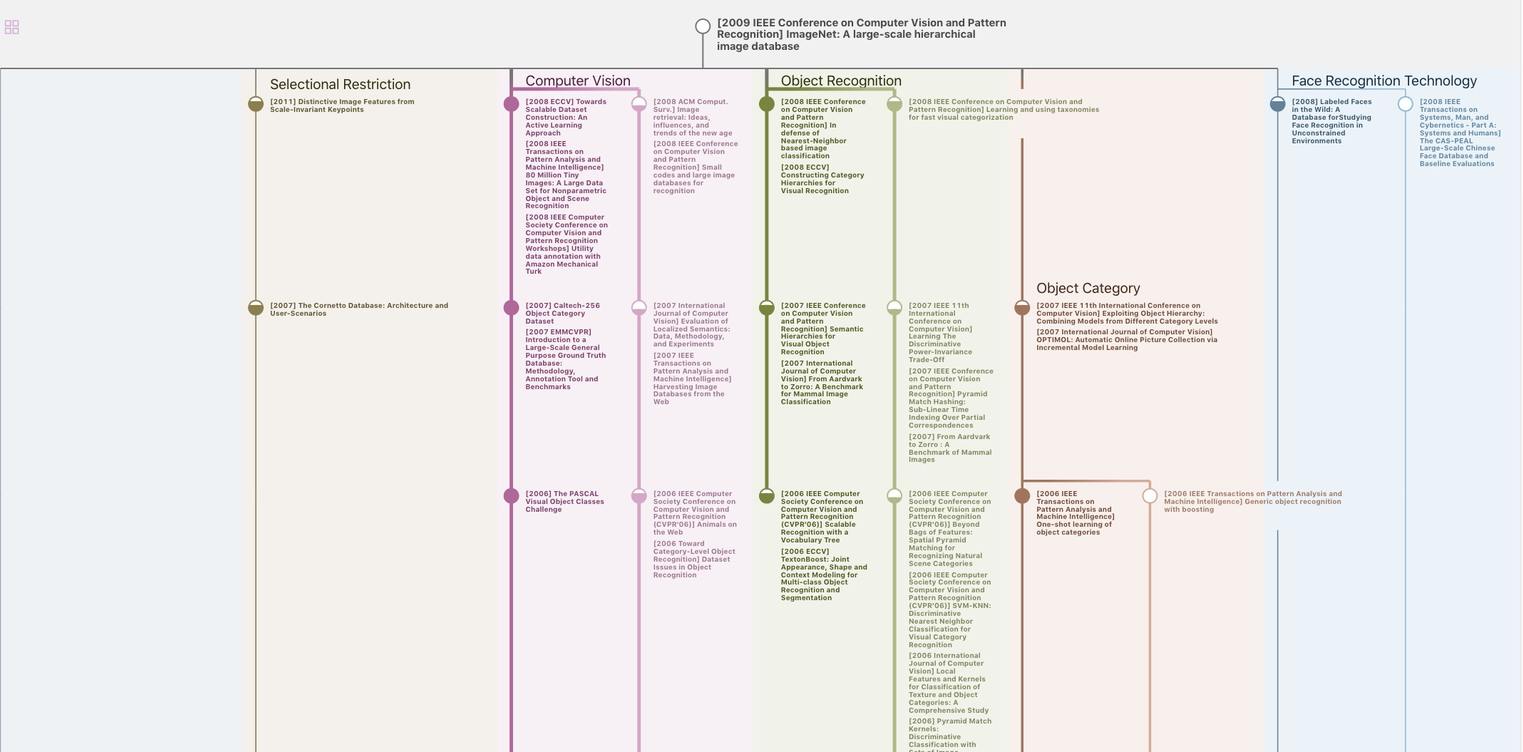
生成溯源树,研究论文发展脉络
Chat Paper
正在生成论文摘要