HeteroPush: Communication-Efficient Video Analytics by Scheduling Heterogeneous Filters.
International Conference on Big Data Computing and Communications(2023)
摘要
A large number of cameras have been deployed in different real world scenarios. Due to the limited computing power and bandwidth, a combined device-cloud framework is typically used to process the captured videos. Filtering techniques select a part of video frames to upload to the cloud, aiming to reduce the computational overhead and bandwidth consumption. As the video content may change during time, a filter that can not adjust to these changes lacks robustness during long-term operation. We find that heterogeneous filters based on various modal features have diverse performances in different time periods, which leads to time-varying local optimality of different filters. In this paper, we design an efficient method to adaptively schedule local optimal filters to perform filtering tasks, aiming to maximize the cost-effectiveness of communication overhead (for example, system accuracy improvement per unit upload). Direct application of existing methods, there are two following challenges in real systems: (1) Noisy samples that with conflict filtering labels but the same on-device inference results; (2) Unpredictability of the correct inference result before uploading frames. To address these challenges, we propose HeteroPush, the first reinforcement learning based adaptive filter scheduling framework. HeteroPush selects the local optimal filter at each moment by modeling the performance of filters in real-time. We perform extensive evaluations on two real-world datasets to demonstrate the effectiveness of our design. The results show that HeteroPush reduces the bandwidth cost by 90% with an additional 2.7% computational overhead.
更多查看译文
关键词
Filter Scheduling,Device-Cloud Collaboration,Communication-Efficient,Video Analytics
AI 理解论文
溯源树
样例
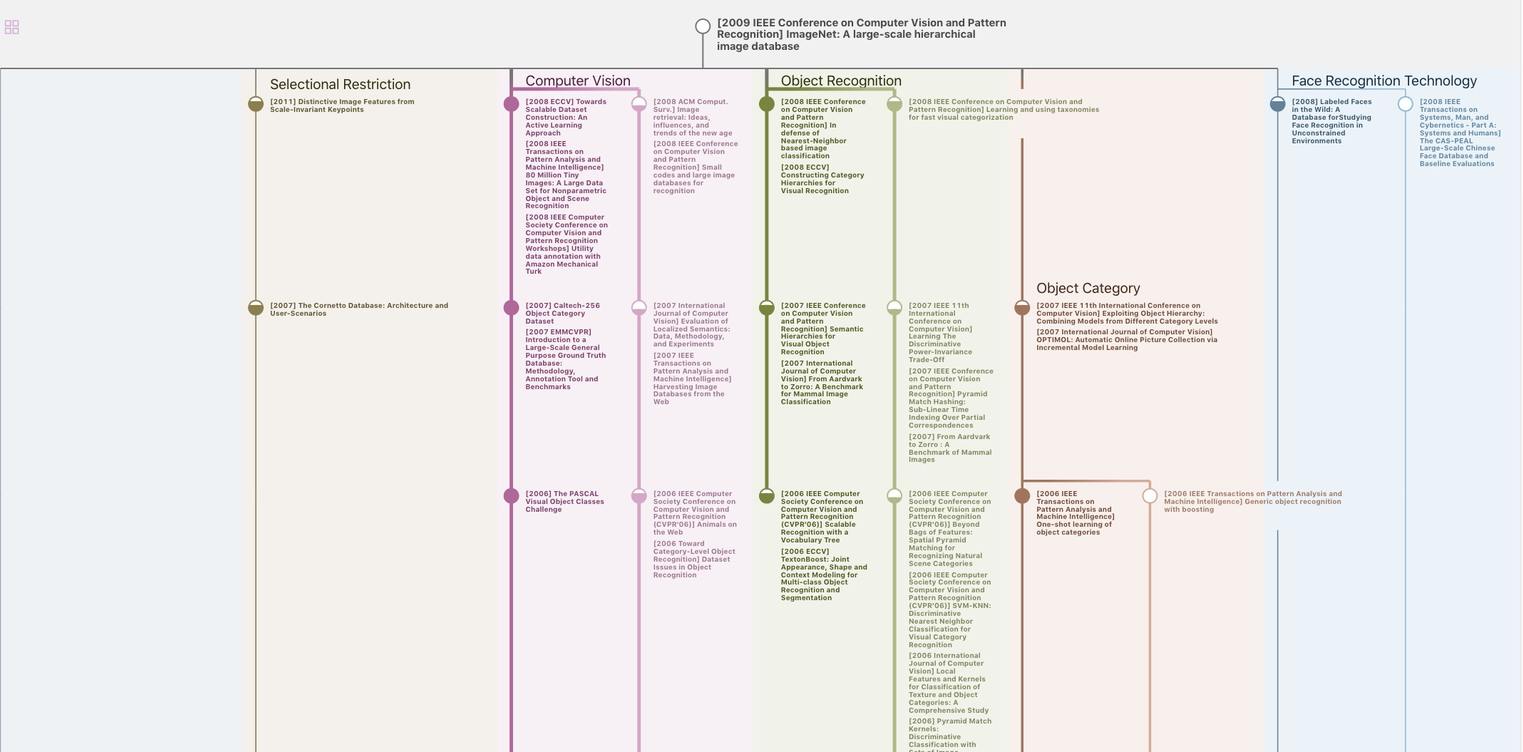
生成溯源树,研究论文发展脉络
Chat Paper
正在生成论文摘要