Characterization of Gauss–Markov stochastic sequences for mission analysis
Astrodynamics(2024)
摘要
In real scenarios, the spacecraft deviates from the intended paths owing to uncertainties in dynamics, navigation, and command actuation. Accurately quantifying these uncertainties is crucial for assessing the observability, collision risks, and mission viability. This issue is further magnified for CubeSats because they have limited control authority and thus require accurate dispersion estimates to avoid rejecting viable trajectories or selecting unviable ones. Trajectory uncertainties arise from random variables (e.g., measurement errors and drag coefficients) and processes (e.g., solar radiation pressure and low-thrust acceleration). Although random variables generally present minimal computational complexity, handling stochastic processes can be challenging because of their noisy dynamics. Nonetheless, accurately modeling these processes is essential, as they significantly influence the uncertain propagation of space trajectories, and an inadequate representation can result in either underestimation or overestimation of the stochastic characteristics associated with a given trajectory. This study addresses the gap in characterizing process uncertainties, represented as Gauss–Markov processes in mission analysis, by presenting models, evaluating derived quantities, and providing results on the impact of spacecraft trajectories. This study emphasizes the importance of accurately modeling random processes to properly characterize stochastic spacecraft paths.
更多查看译文
关键词
Gauss–Markov process,uncertainty quantification,stochastic dynamics,Monte Carlo simulation
AI 理解论文
溯源树
样例
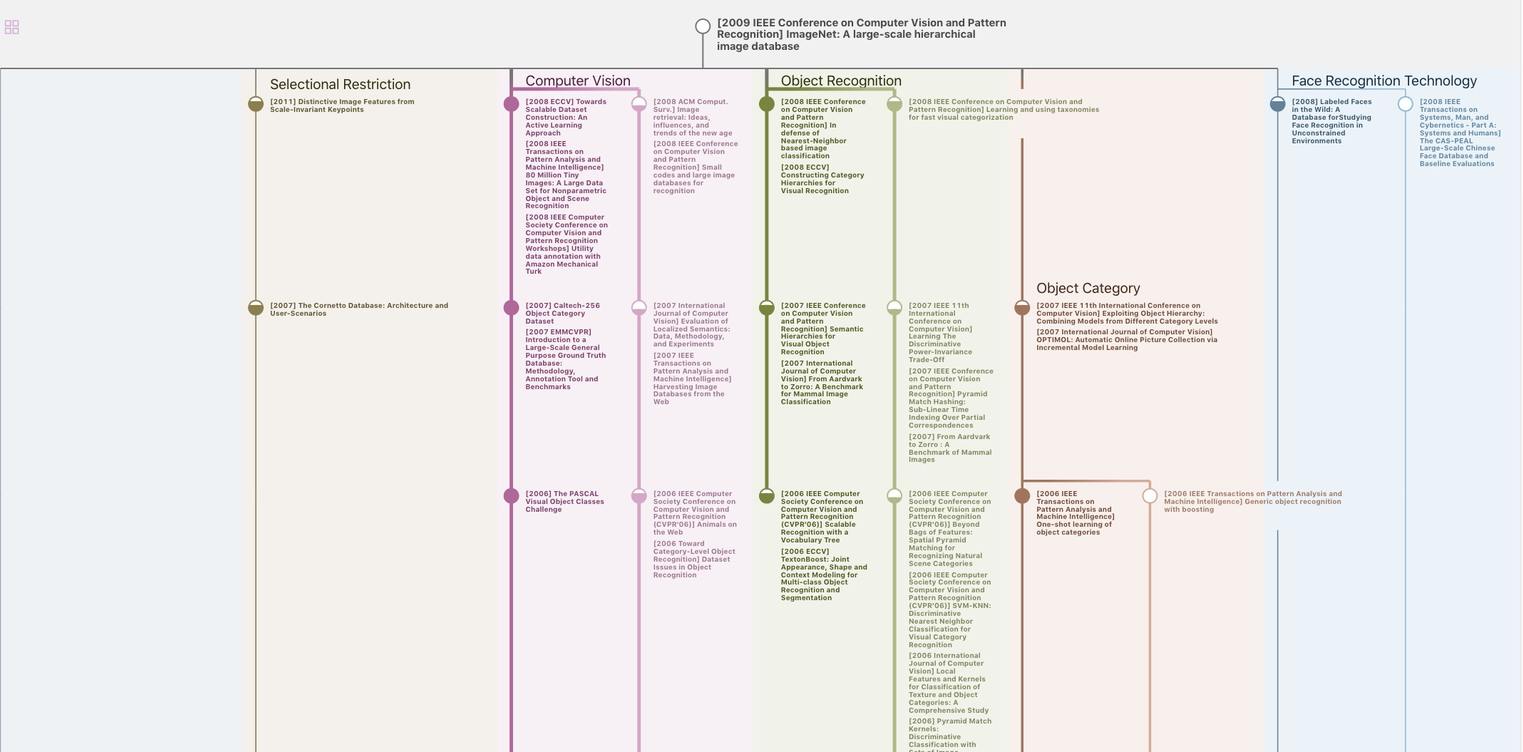
生成溯源树,研究论文发展脉络
Chat Paper
正在生成论文摘要