Hashing Beam Training for Near-Field Integrated Sensing and Communication Systems
arxiv(2024)
摘要
In millimeter-wave (mmWave) integrated sensing and communication networks,
users may be within the coverage of multiple access points (AP), which
typically employ large-scale antenna arrays to mitigate obstacle occlusion and
path loss. However, large-scale arrays generate pencil-shaped beams, which
necessitate a higher number of training beams to cover the desired space.
Furthermore, as the antenna aperture increases, users are more likely to be
situated in the near-field region of the AP antenna array. This motivates our
investigation into the near-field beam training problem to achieve effective
positioning services. To address the high complexity and low identification
accuracy of existing beam training techniques, we propose an efficient hashing
multi-arm beam (HMB) training scheme for the near-field scenario. Specifically,
we first construct a near-field single-beam training codebook for the uniform
planar arrays. Then, the hash functions are chosen independently to construct
the multi-arm beam training codebooks for each AP. All APs traverse the
predefined multi-arm beam training codeword simultaneously and the multi-AP
superimposed signals at the user are recorded. Finally, the soft decision and
voting methods are applied to obtain the correctly aligned beams only based on
the signal powers. In addition, we logically prove that the traversal
complexity is at the logarithmic level. Simulation results show that our
proposed near-field HMB training method can achieve 96.4
accuracy of the exhaustive beam training method and greatly reduce the training
overhead. Furthermore, we verify its applicability under the far-field scenario
as well.
更多查看译文
AI 理解论文
溯源树
样例
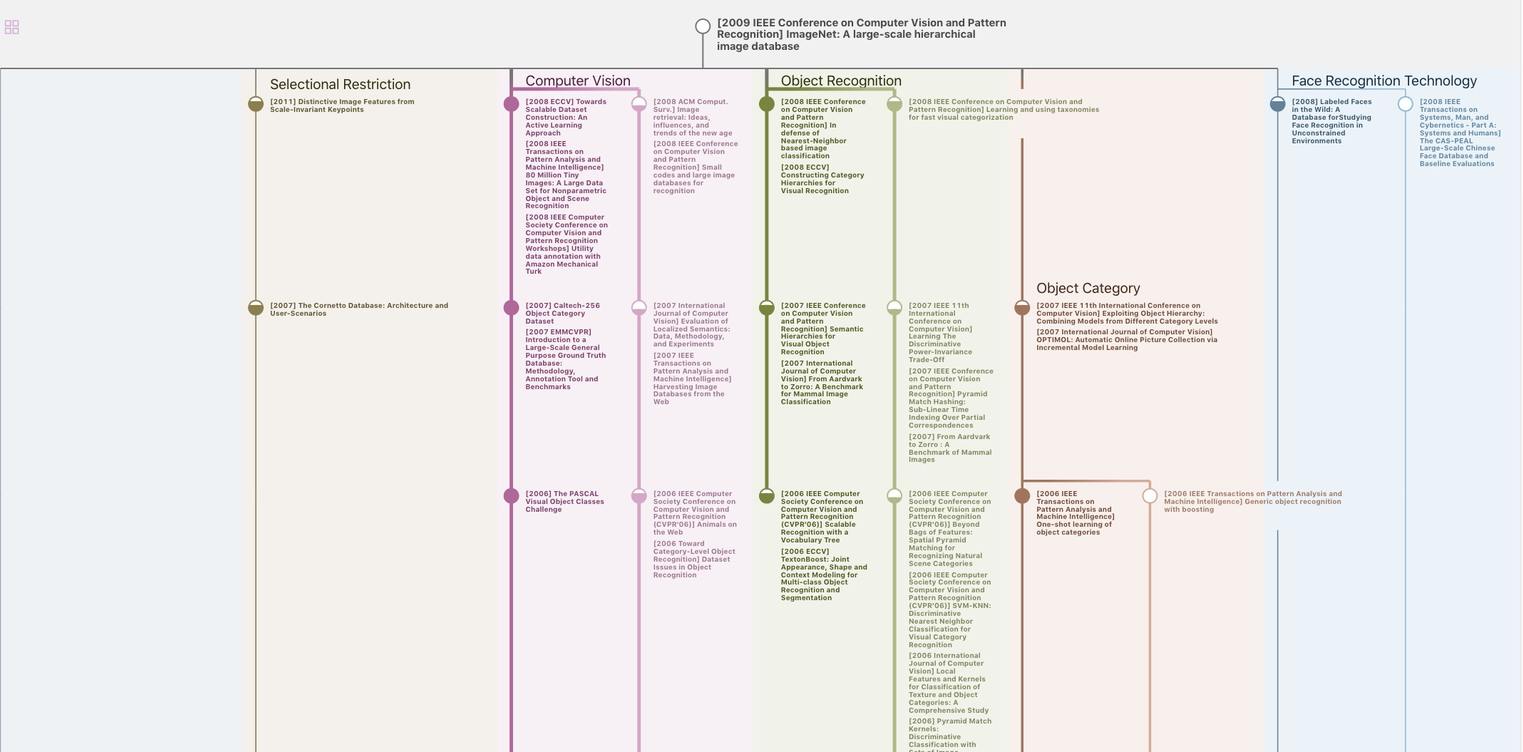
生成溯源树,研究论文发展脉络
Chat Paper
正在生成论文摘要