Comparison of methods for intravoxel incoherent motion parameter estimation in the brain from flow-compensated and non-flow-compensated diffusion-encoded data.
Magnetic resonance in medicine(2024)
摘要
PURPOSE:Joint analysis of flow-compensated (FC) and non-flow-compensated (NC) diffusion MRI (dMRI) data has been suggested for increased robustness of intravoxel incoherent motion (IVIM) parameter estimation. For this purpose, a set of methods commonly used or previously found useful for IVIM analysis of dMRI data obtained with conventional diffusion encoding were evaluated in healthy human brain.
METHODS:Five methods for joint IVIM analysis of FC and NC dMRI data were compared: (1) direct non-linear least squares fitting, (2) a segmented fitting algorithm with estimation of the diffusion coefficient from higher b-values of NC data, (3) a Bayesian algorithm with uniform prior distributions, (4) a Bayesian algorithm with spatial prior distributions, and (5) a deep learning-based algorithm. Methods were evaluated on brain dMRI data from healthy subjects and simulated data at multiple noise levels. Bipolar diffusion encoding gradients were used with b-values 0-200 s/mm2 and corresponding flow weighting factors 0-2.35 s/mm for NC data and by design 0 for FC data. Data were acquired twice for repeatability analysis.
RESULTS:Measurement repeatability as well as estimation bias and variability were at similar levels or better with the Bayesian algorithm with spatial prior distributions and the deep learning-based algorithm for IVIM parameters D $$ D $$ and f $$ f $$ , and for the Bayesian algorithm only for v d $$ {v}_d $$ , relative to the other methods.
CONCLUSION:A Bayesian algorithm with spatial prior distributions is preferable for joint IVIM analysis of FC and NC dMRI data in the healthy human brain, but deep learning-based algorithms appear promising.
更多查看译文
AI 理解论文
溯源树
样例
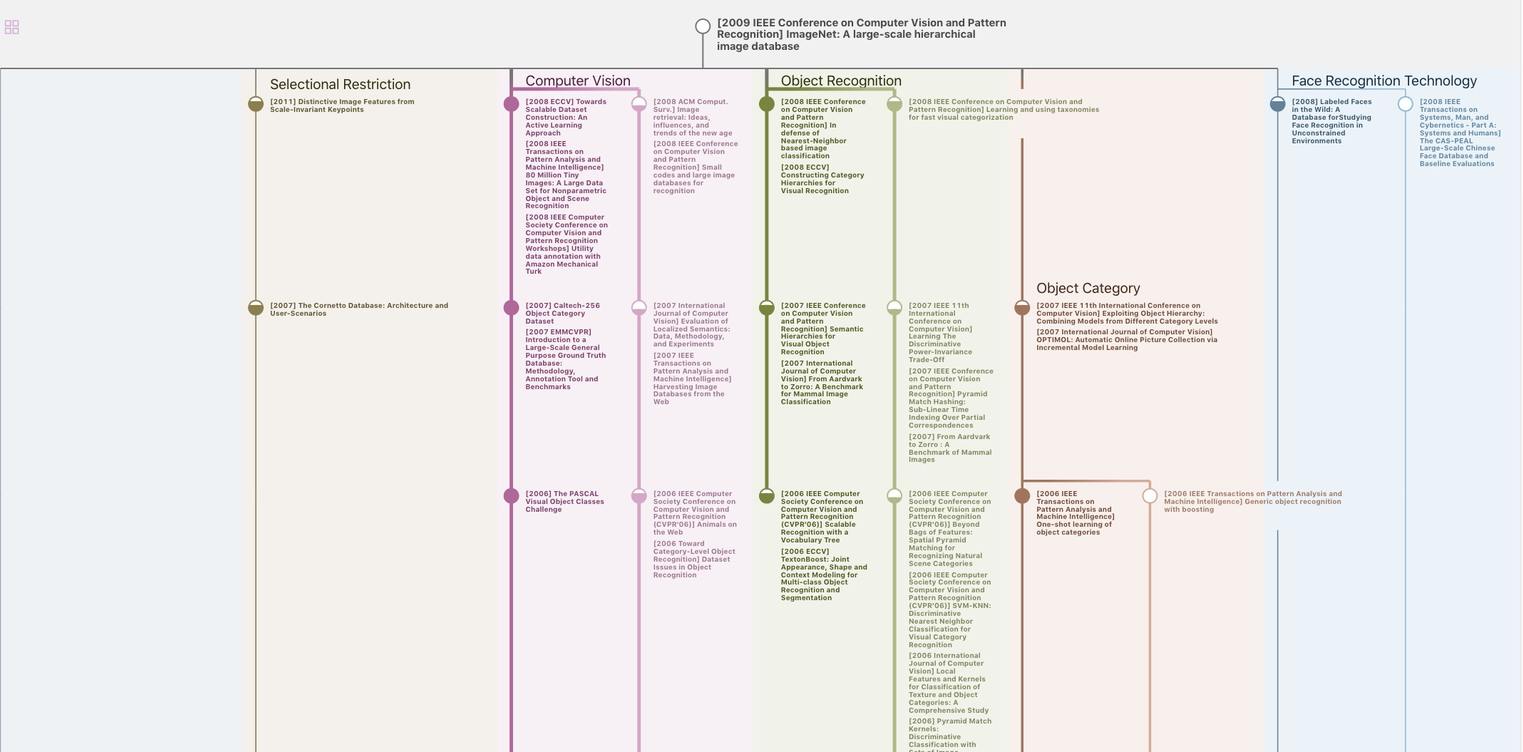
生成溯源树,研究论文发展脉络
Chat Paper
正在生成论文摘要