Golub Kahan Based Methods for Partial Multidimensional Singular Value Decomposition.
2023 13th International Conference on Dependable Systems, Services and Technologies (DESSERT)(2023)
摘要
Many applications of machine learning, data, image and signal processing require the design of algorithms capable of dealing with very large datasets. Extracting the most meaningful features out of these large datasets is often a computationally challenging task. In the matrix case, the Singular Value Decomposition (SVD) has shown to be a very efficient tool to do so. In recent years there has been a very significant increase in the amount of available data and the datasets are often formatted as tensors, for which some generalisations of the SVD have already been proposed for some specific choices of tensor-tensor products, well adapted to tensors of order 3. In this work, we propose a new generalisation, associated with the well-known Einstein product, regardless of the order of the tensors. As in the matrix case, only a few singular elements need to be computed and we address this problem by proposing an iterative approach based on the tensor Lanczos bidiagonalization applied to the Einstein product. We also propose a refinement to this method in order to increase its accuracy. We give numerical results for both synthetic and real data to illustrate the efficiency of these methods.
更多查看译文
关键词
Tensor,Einstein product,SVD,Image processing
AI 理解论文
溯源树
样例
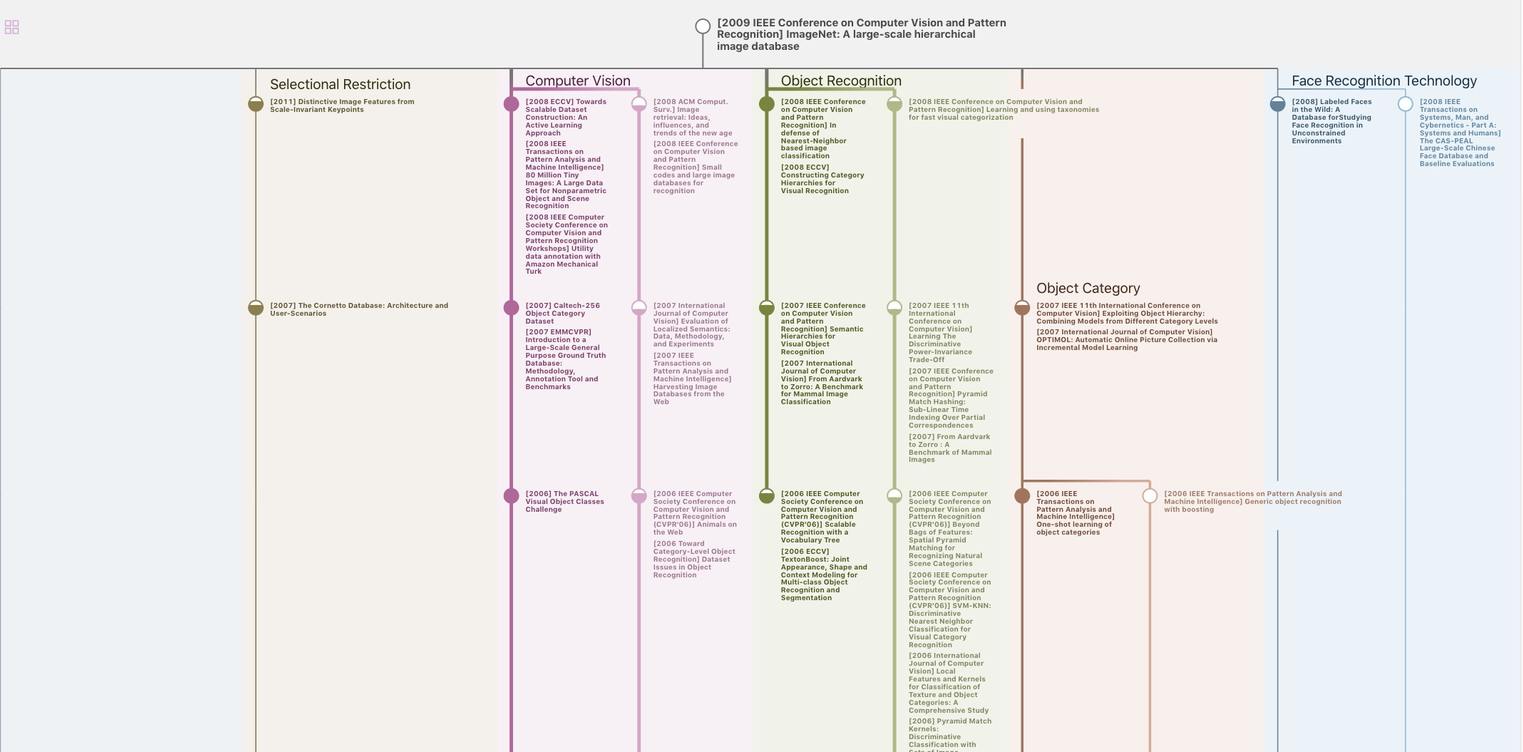
生成溯源树,研究论文发展脉络
Chat Paper
正在生成论文摘要