Toward Solving Domain Adaptation with Limited Source Labeled Data
2023 23RD IEEE INTERNATIONAL CONFERENCE ON DATA MINING WORKSHOPS, ICDMW 2023(2023)
摘要
The success of domain adaptation relies on highquality labeled data from the source domain, which is a luxury setup for applied machine learning problems. This article investigates a particular challenge: the source labeled data are neither plentiful nor sufficiently representative. We studied the challenge of limited data with an industrial application, i.e., forklift truck activity recognition. The task is to develop data-driven methods to recognize forklift usage performed in different warehouses with a large scale of signals collected from the onboard sensors. The preliminary results show that using pseudo-labeled data from the source domain can significantly improve classification performance on the target domain in some tasks. As the realworld problems are much more complex than typical research settings, it is not clearly understood in what circumstance the improvement may occur. Therefore, we provided discussions regarding this phenomenon and shared several inspirations on the difficulty of understanding and debugging domain adaptation problems in practice.
更多查看译文
关键词
Domain Adaptation,Pseudo-label,Time-Series,Limited Data,Activity Recognition,DANN
AI 理解论文
溯源树
样例
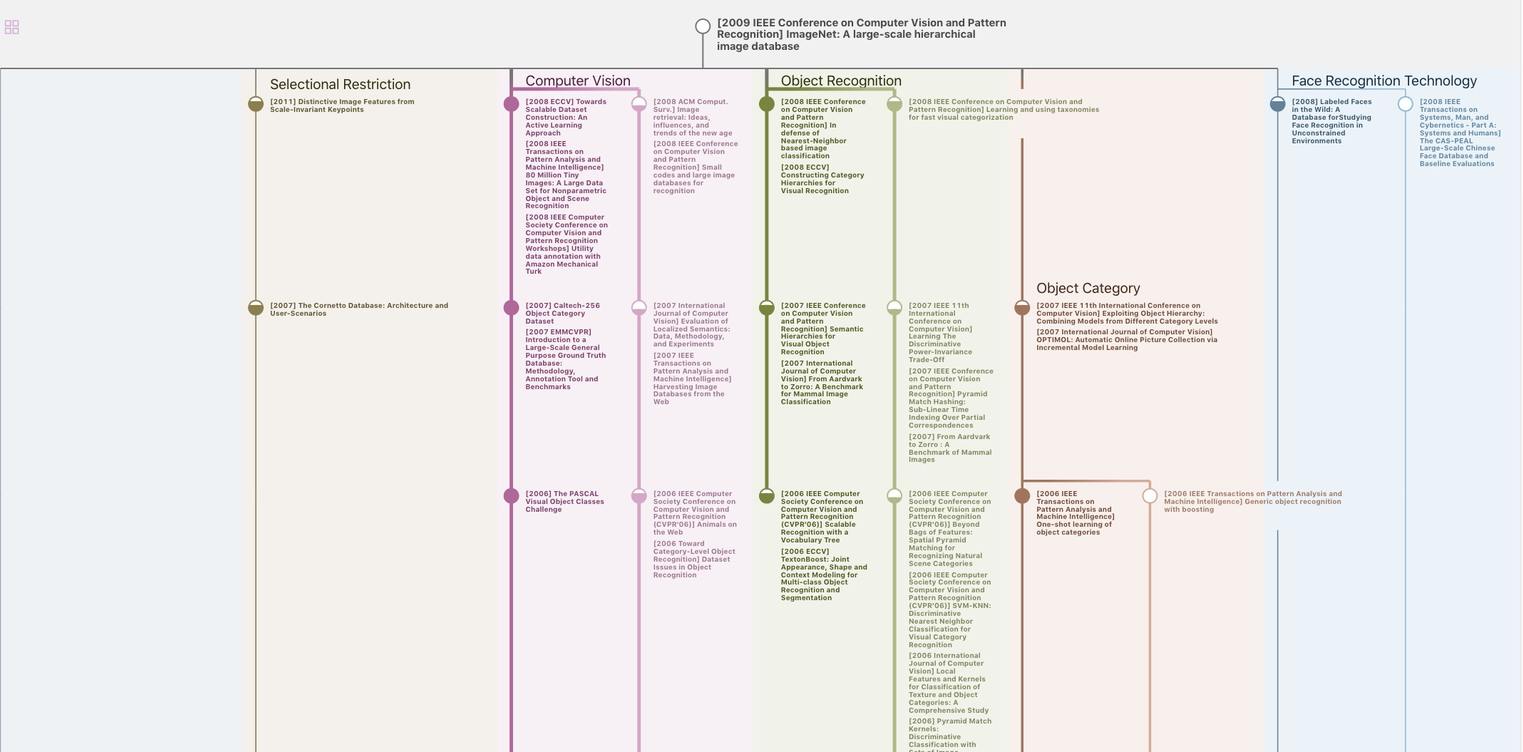
生成溯源树,研究论文发展脉络
Chat Paper
正在生成论文摘要