On Graph Representation based Re-Identification A Proof of Concept
2023 23RD IEEE INTERNATIONAL CONFERENCE ON DATA MINING WORKSHOPS, ICDMW 2023(2023)
摘要
This submission introduces an innovative method based on graph representation for re-identifying chipwood surface structures. In the specific application under consideration, it also addresses the re-identification of Euro-pallets. To achieve this objective, we propose a departure from conventional reidentification methods by advocating the substitution of the standard image representation with a significantly compressed graph representation. This not only facilitates the development of an efficient algorithm but also imparts resilience to environmental variations, including rotation and shearing. The resultant approach, named "IRAG" (Image Representation through Anomaly Graphs), manifests as a siamese graph neural network. This network is employed on a pre-existing dataset, which comprises images sourced from 502 EPAL pallet blocks and has been previously published. The obtained results from this approach show a rank-1 accuracy of 27 Additionally, the experiments conducted in this work demonstrate that the re-identification accuracy of the model is not affected by rotation or shearing, indicating the model's invariance to these environmental changes. This paper represents an updated and improved version of the paper "Towards graph representation-based re-identification of chipwood pallet blocks" published at ICMLA 2022.
更多查看译文
关键词
graph representations,resource efficient neural networks,re-identification,warehousing logistics
AI 理解论文
溯源树
样例
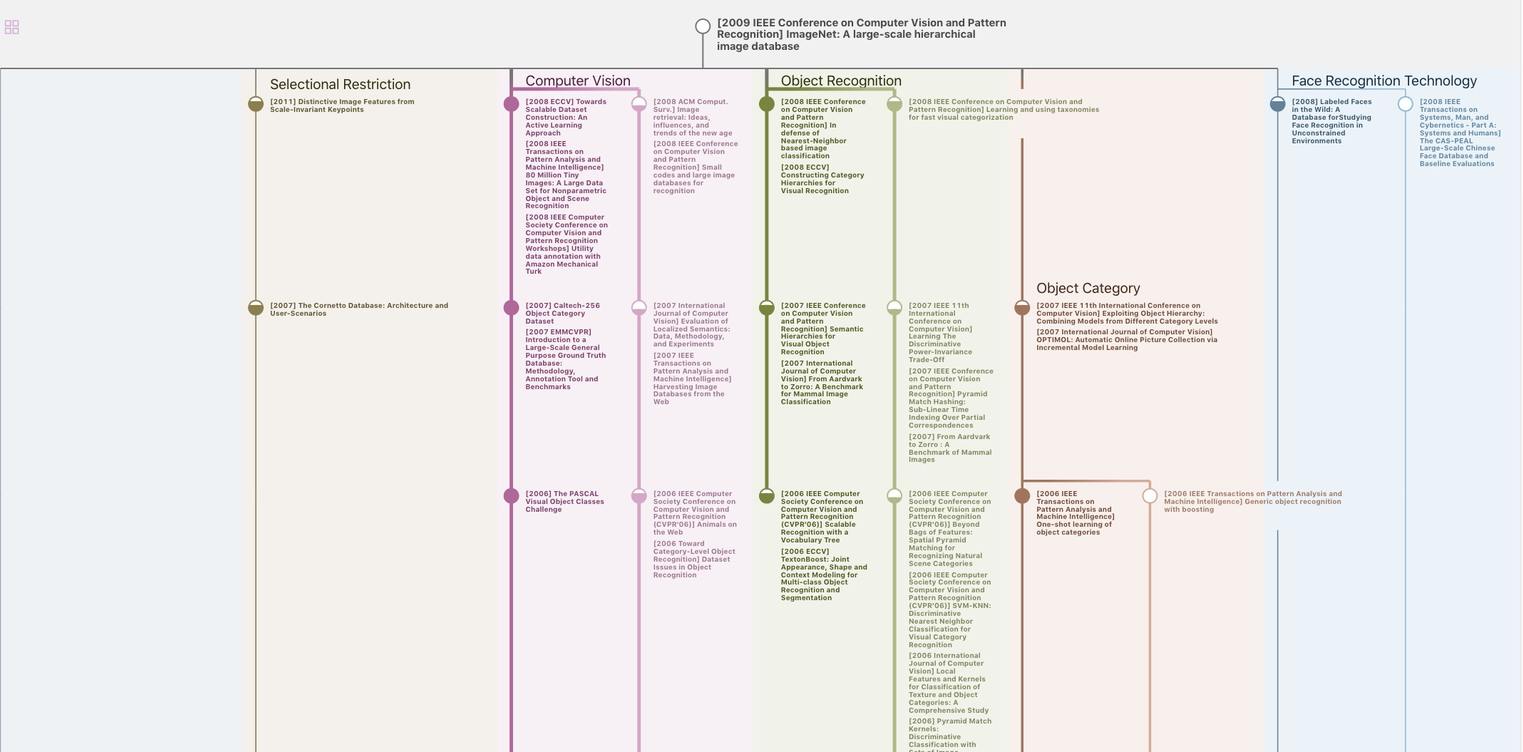
生成溯源树,研究论文发展脉络
Chat Paper
正在生成论文摘要