Cross-Modal Attention Network for Sign Language Translation
2023 23RD IEEE INTERNATIONAL CONFERENCE ON DATA MINING WORKSHOPS, ICDMW 2023(2023)
摘要
Context serves as a critical role in sign language translation (SLT), e.g., textual questions from the hearing people and visual answers from the hearing-impaired people in communication have a contextual relationship, which can facilitate the understanding of sign language. Current SLT approaches mainly focus on learning visual representations and translate it into spoken or written language sentences, while neglect the utilization of context information in question-answer (QA) scenarios. In this paper, we propose a novel and effective Cross-Modal Attention Network (CMA-Net) to better learn multimodal features (i.e., text and vision) for improving the translation accuracy of SLT with the aid of contextual information. Specifically, we design a cross-modal knowledge transfer module, which embeds the star-based attention mechanism into the Transformer model to explore a short-term and long-term interactive relationship between different modalities and achieve cross-modality message transfer. Besides, we propose a multi-task learning paradigm, i.e., using auxiliary recognition (sign2gloss) and translation (gloss2text) tasks to augment the main task with a pre-training strategy. Significant performance improvements on three public benchmark datasets demonstrate the effectiveness of CMA-Net and the usefulness of context information for SLT.
更多查看译文
关键词
cross-modal attention,knowledge transfer,multi-task learning,sign language translation
AI 理解论文
溯源树
样例
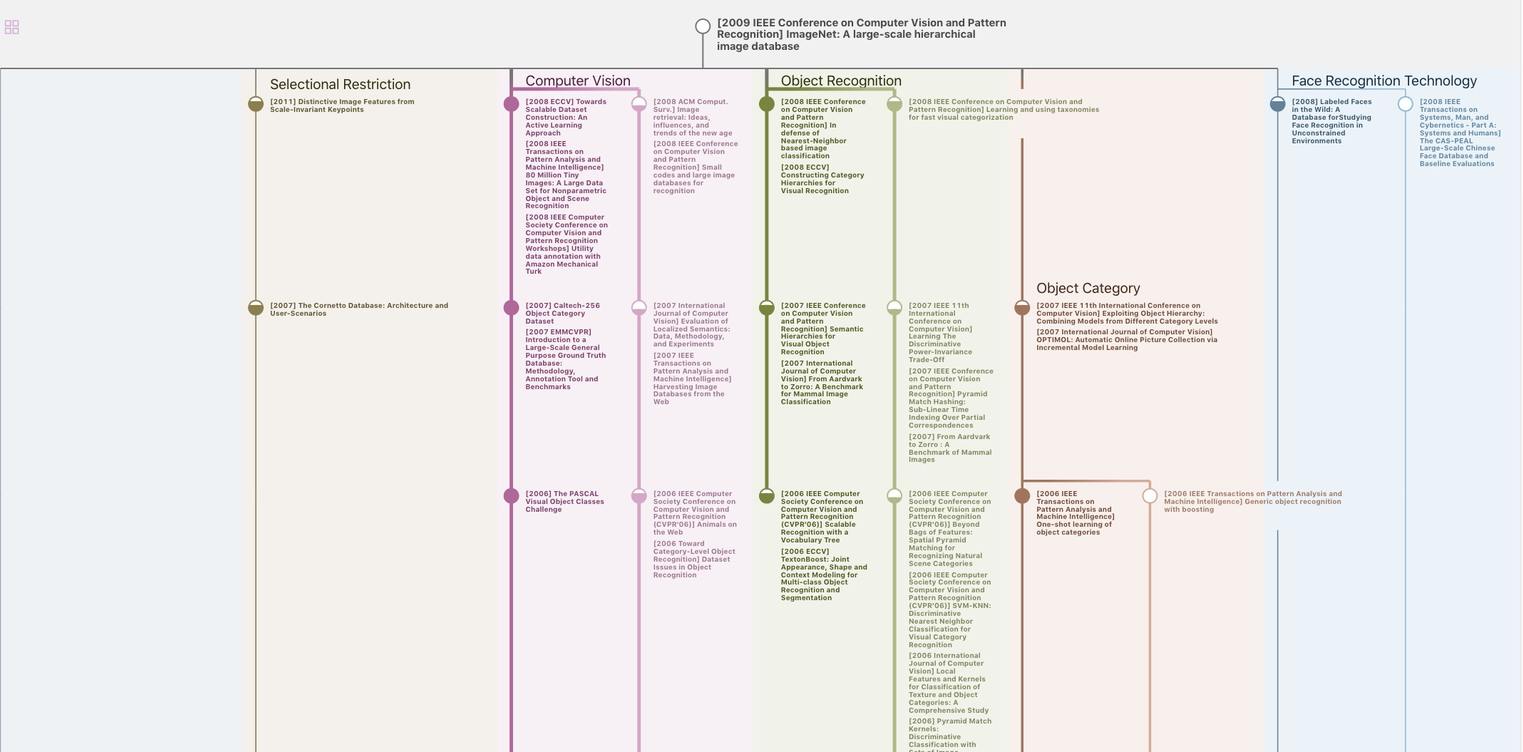
生成溯源树,研究论文发展脉络
Chat Paper
正在生成论文摘要