Evolutionary Multi-model Federated Learning on Malicious and Heterogeneous Data
2023 23RD IEEE INTERNATIONAL CONFERENCE ON DATA MINING WORKSHOPS, ICDMW 2023(2023)
摘要
The federated learning system is vulnerable to data poisoning attacks from malicious clients, who often present heterogeneous data. Poisoning such heterogeneous data can lead to significant diversity in the local datasets. Traditional singlemodel federated learning struggles to effectively handle this diverse knowledge. To address this challenge, we propose an evolutionary multi-model federated learning framework called EMFL, which trains multiple models with diversity to learn from the diverse data effectively. Specifically, we introduce a gradientbased particle swarm optimization approach, which incorporates gradient information from the models and utilizes a multielite strategy to achieve quick convergence and promote model diversity. Furthermore, we propose a client-matching strategy based on cosine similarity of multi-model updates, allowing for analyzing the similarity of distribution patterns in diverse data and matching clients with concentrated distributions, thus helping to prevent excessive models from converging to malicious data and causing extreme model diversity. The proposed EMFL employs multiple lightweight models instead of a large architecture one to overcome training challenges for clients with limited computing power. Experimental results on nine datasets demonstrate consistent outperformance of our proposed algorithm compared to its counterparts.
更多查看译文
关键词
Federated learning,particle swarm optimization,heterogeneous data,malicious data.
AI 理解论文
溯源树
样例
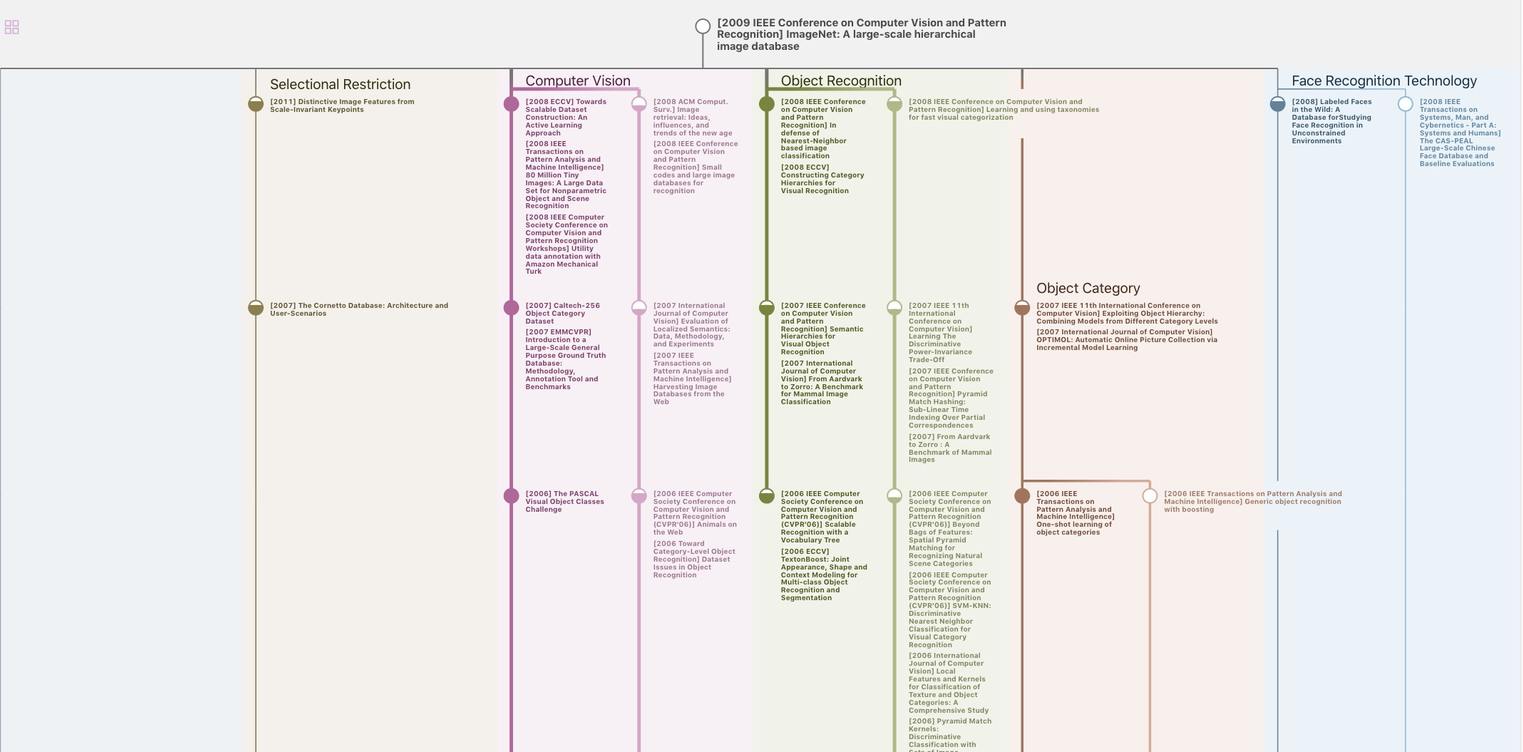
生成溯源树,研究论文发展脉络
Chat Paper
正在生成论文摘要