Online neuro-fuzzy model learning of dynamic systems with measurement noise
Nonlinear Dynamics(2024)
摘要
Model identification of nonlinear time varying dynamic systems is challenging because the system behaviours may vary significantly in different operational conditions. If the changes are insufficiently captured by training data, the trained model is unable to capture the system response well when the operational condition changes. The model performance may also be deteriorated in real-time implementation due to the noise in sensors or the environment. This paper presents a self-adaptive Neuro-Fuzzy (NF) modelling framework to address these challenges. The NF model, trained offline based on experimental data, combines the Auto-Regressive with eXogenous (ARX) models and Gaussian activation functions to capture the nonlinear system behaviours. During online implementation, the ARX model parameters are updated using new data through a recursive generalised least squares method, which embeds a noise model to eliminate effects of the noise. The online updating algorithm has a provable convergence guarantee and enables the proposed NF model to adapt to changes in system behaviours automatically. Efficacy of the algorithm is verified through two numerical examples and an experiment on a commercial automotive engine.
更多查看译文
关键词
Neuro-Fuzzy model,Recursive identification,Online learning,Measurement noise
AI 理解论文
溯源树
样例
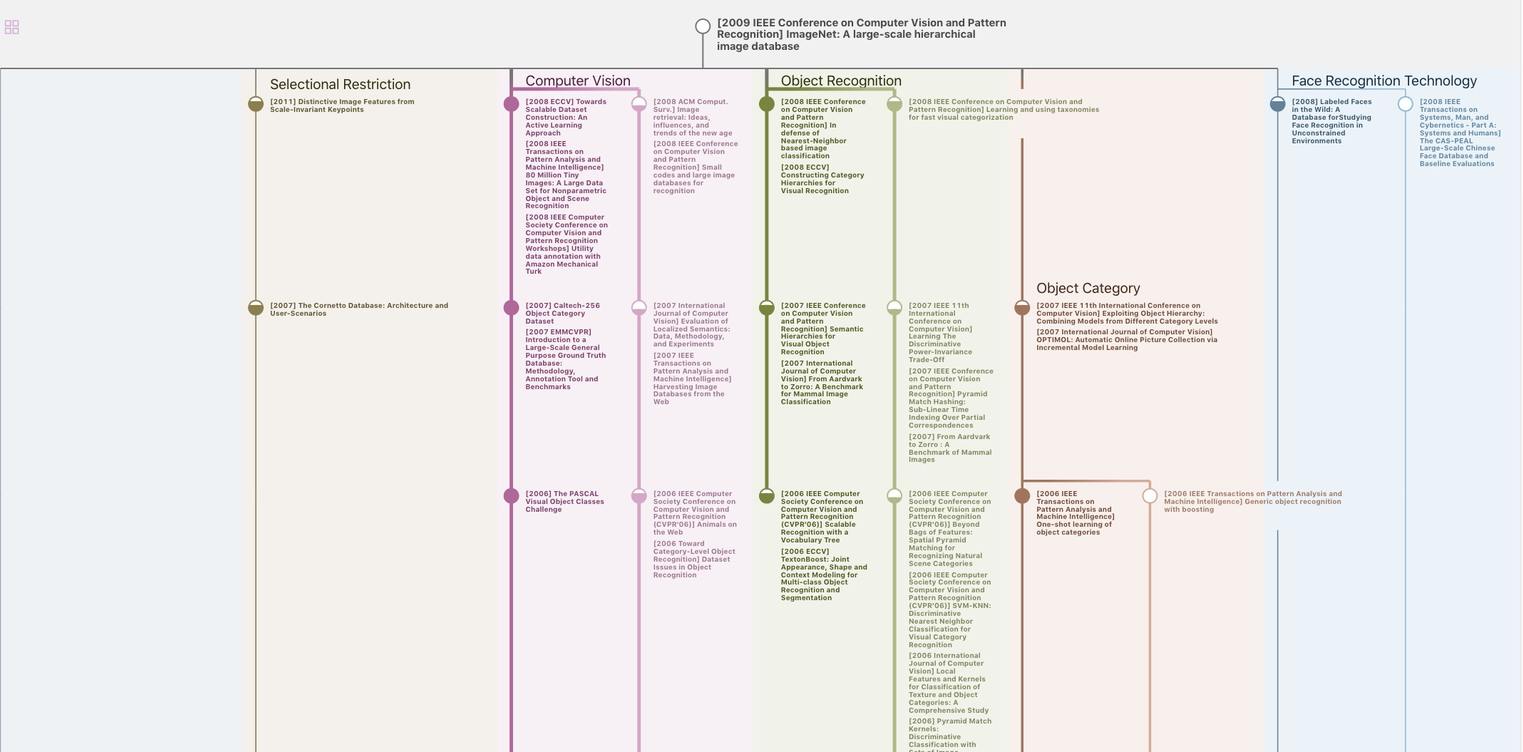
生成溯源树,研究论文发展脉络
Chat Paper
正在生成论文摘要