Wearable Neural Interfaces: Real-Time Identification of Motor Neuron Discharges in Dynamic Motor Tasks
biorxiv(2024)
摘要
Objective: Robustness to non-stationary conditions is essential to develop stable and accurate wearable neural interfaces. Approach: We propose a novel adaptive electromyography (EMG) decomposition algorithm that builds on blind source separation methods by leveraging the Kullback-Liebler divergence and kurtosis of the signals as metrics for online learning. The proposed approach provides a theoretical framework to tune the adaptation hyperparameters and compensate for non-stationarities in the mixing matrix, such as due to dynamic contractions, and to identify the underlying motor neuron (MN) discharges. The adaptation is performed in real-time (~22 ms of computational time per 100-ms batches). Main Results: The proposed adaptation algorithm significantly improved all decomposition performance metrics with respect to the absence of adaptation in a wide range of motion of the wrist (80°). The rate of agreement, sensitivity, and precision were ≥90% in ≥80% of the cases in both simulated and experimentally recorded data, according to a two-source validation approach. Significance: The findings demonstrate the feasibility of accurately decoding MN discharges in real-time during dynamic contractions from wearable systems mounted at the wrist and forearm. Moreover, the study proposes an experimental validation method for EMG decomposition in dynamic tasks.
### Competing Interest Statement
DF is Scientific Advisor for Reality Labs with compensation for this service from Meta. DY B and DF are inventors in a patent (Neural Interface. UK Patent Application No. GB1813762.0. 23 August 2018) and patent application (Neural interface. UK Patent Application No. GB2014671.8. 17 September 2020) related to the methods and applications of this work.
更多查看译文
AI 理解论文
溯源树
样例
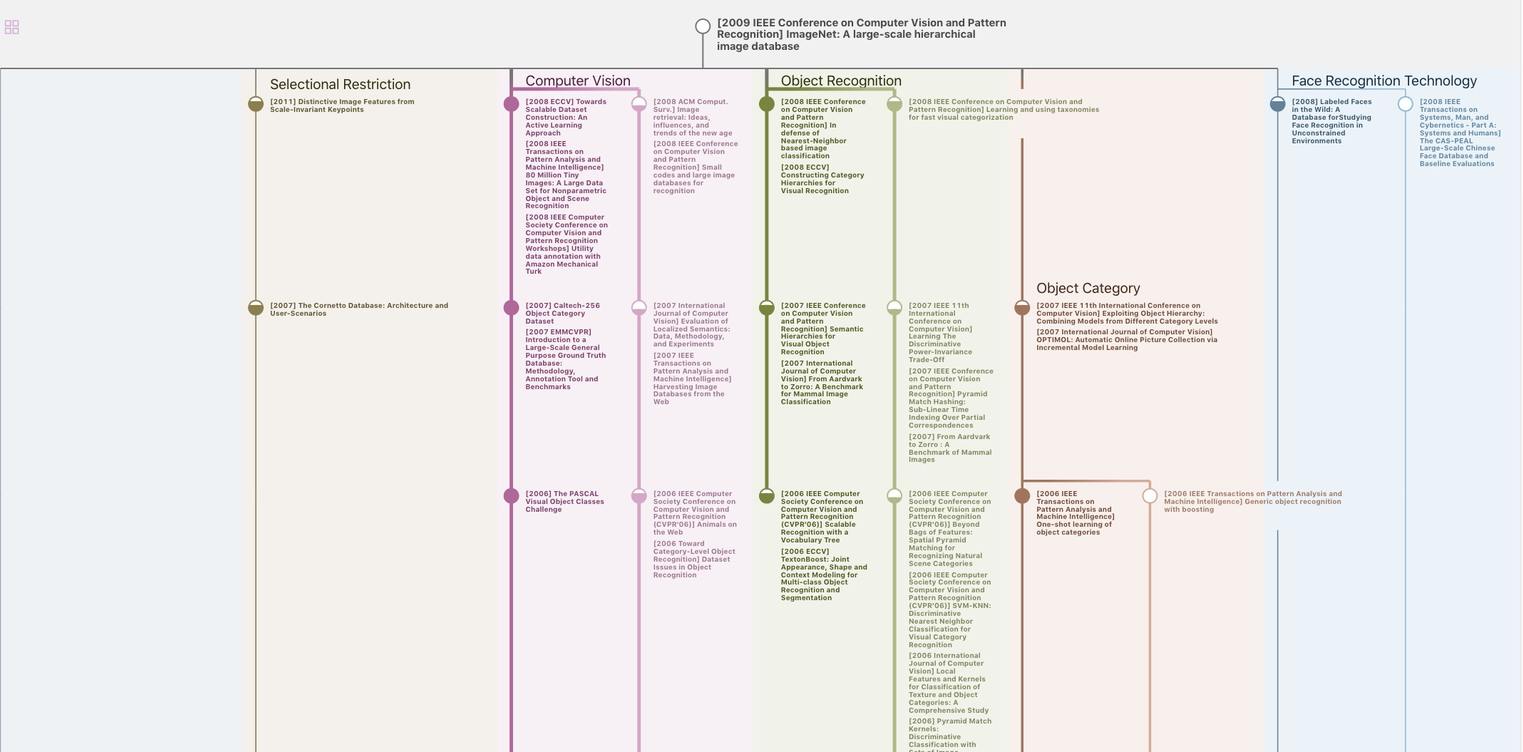
生成溯源树,研究论文发展脉络
Chat Paper
正在生成论文摘要