AirPhyNet: Harnessing Physics-Guided Neural Networks for Air Quality Prediction
CoRR(2024)
摘要
Air quality prediction and modelling plays a pivotal role in public health
and environment management, for individuals and authorities to make informed
decisions. Although traditional data-driven models have shown promise in this
domain, their long-term prediction accuracy can be limited, especially in
scenarios with sparse or incomplete data and they often rely on black-box deep
learning structures that lack solid physical foundation leading to reduced
transparency and interpretability in predictions. To address these limitations,
this paper presents a novel approach named Physics guided Neural Network for
Air Quality Prediction (AirPhyNet). Specifically, we leverage two
well-established physics principles of air particle movement (diffusion and
advection) by representing them as differential equation networks. Then, we
utilize a graph structure to integrate physics knowledge into a neural network
architecture and exploit latent representations to capture spatio-temporal
relationships within the air quality data. Experiments on two real-world
benchmark datasets demonstrate that AirPhyNet outperforms state-of-the-art
models for different testing scenarios including different lead time (24h, 48h,
72h), sparse data and sudden change prediction, achieving reduction in
prediction errors up to 10
model captures underlying physical processes of particle movement and generates
accurate predictions with real physical meaning.
更多查看译文
关键词
air quality prediction,physics-informed,spatiotemporal-learning,interpretability
AI 理解论文
溯源树
样例
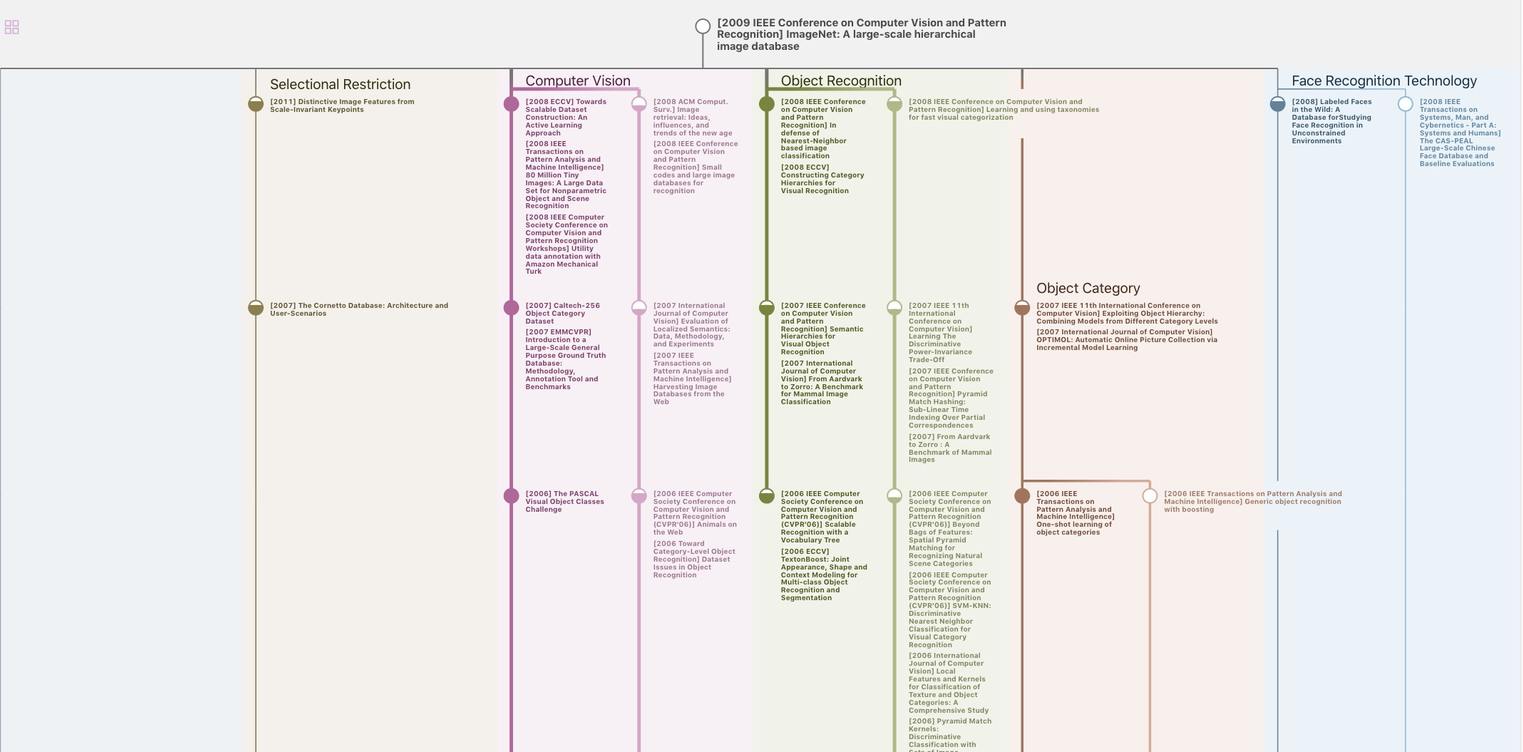
生成溯源树,研究论文发展脉络
Chat Paper
正在生成论文摘要